Self-Attentive Recommendation for Multi-Source Review Package
2021 International Joint Conference on Neural Networks (IJCNN)(2021)
摘要
With the diversified sources satisfying users' needs, many online service platforms collect information from multiple sources in order to provide a set of useful information to the users. However, existing recommendation systems are mostly designed for single-source data, and thus fail to recommend multi-source review packages since the interplay between the reviews of different sources is not properly modeled. In fact, modeling the interplay between different sources is challenging because 1) two reviews may conflict with each other, 2) different users have different preferences on review sources, and 3) users' preferences to each source may change under different scenarios. To address these challenges, we propose Self-Attentive Recommendation for multi-source review Package (SARP), for predicting how useful the user feels to the package, while simultaneously reflecting how much the user is affected by each review. Specifically, SARP jointly considers the relationships of every user, purpose, and review source to learn better latent representations. A self-attention module is further used for integrating source representations and the review ratings, following a multi-layer perceptron (MLP) for the prediction tasks. Experimental results on the self-constructed dataset and public dataset demonstrate that the proposed model outperforms the state-of-the-art approaches.
更多查看译文
关键词
multi-source review,recommendation system,deep learning
AI 理解论文
溯源树
样例
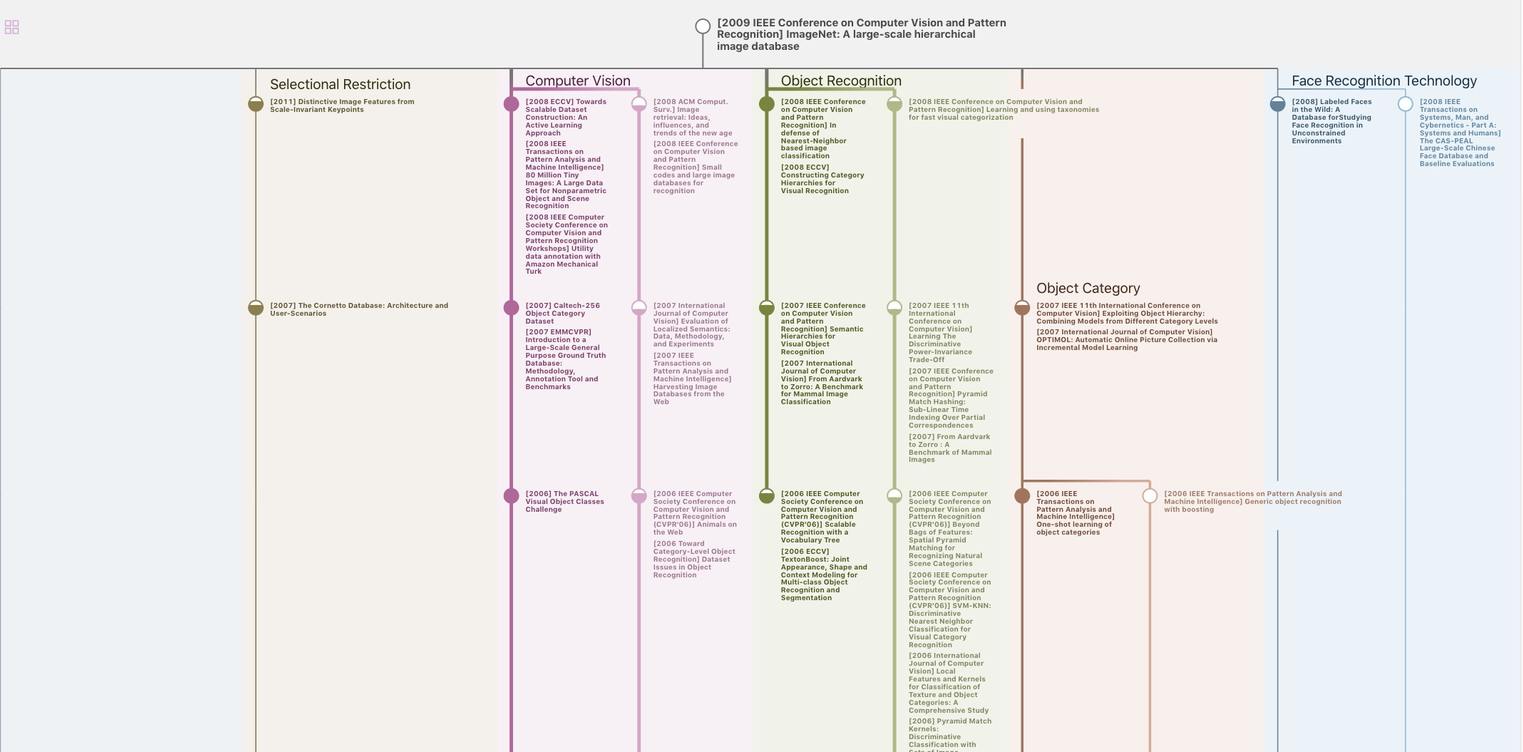
生成溯源树,研究论文发展脉络
Chat Paper
正在生成论文摘要