Sequential Data Imputation with Evolving Generative Adversarial Networks
2021 INTERNATIONAL JOINT CONFERENCE ON NEURAL NETWORKS (IJCNN)(2021)
摘要
Data imputation is an important and widely researched problem in data analysis with applications to a variety of problem domains. Existing literature explores different methods to estimate missing values in a dataset. In this paper, we analyze the task of imputing multiple incomplete numerical datasets that arrive sequentially, each with an overlapping yet distinguishable set of features.We propose a novel procedure based on the Generative Adversarial Network (GAN) architecture, called EvoGAN, which uses transfer learning to build a unified model to perform imputation for all datasets in the sequence. Our methods exploit overlap between datasets and utilize the knowledge gathered from previous rounds. We validate our model on several sequences of datasets and find compelling results for compression rate and acceleration which outperform the state-of-the-art imputation models that are based on an input of a single dataset.
更多查看译文
关键词
Data Imputation, Missing Values, Generative Adversarial Network, Machine Learning, Deep Learning, Neural Network
AI 理解论文
溯源树
样例
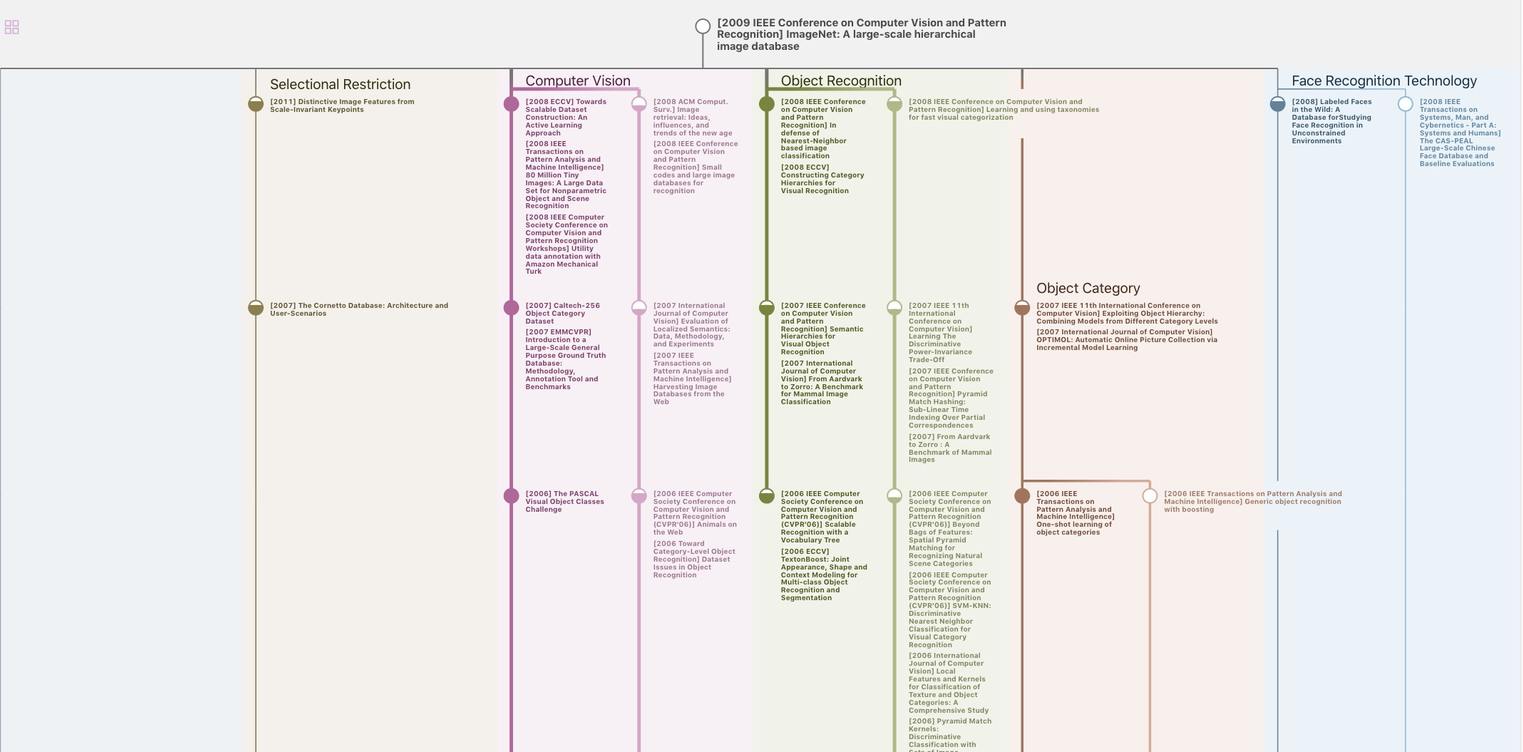
生成溯源树,研究论文发展脉络
Chat Paper
正在生成论文摘要