Studying the Transferability of Non-Targeted Adversarial Attacks
2021 INTERNATIONAL JOINT CONFERENCE ON NEURAL NETWORKS (IJCNN)(2021)
摘要
There is no doubt that the use of machine learning is increasing every day. Its applications include self-driving cars, malware detection, recommendation systems and many other fields. Although the broad scope of this technology highlights the importance of its reliability, it has been shown that machine learning models can be vulnerable to adversarial attacks. In this paper, we study a property of these attacks called transferability across different architectures and models, measuring how these attacks transfer based on a specific number of parameters among three adversarial attacks: Fast Gradient Sign Method, Projected Gradient Descent and HopSkipJumpAttack.
更多查看译文
关键词
Deep Learning, Adversarial Attacks, Convolutional Neural Networks
AI 理解论文
溯源树
样例
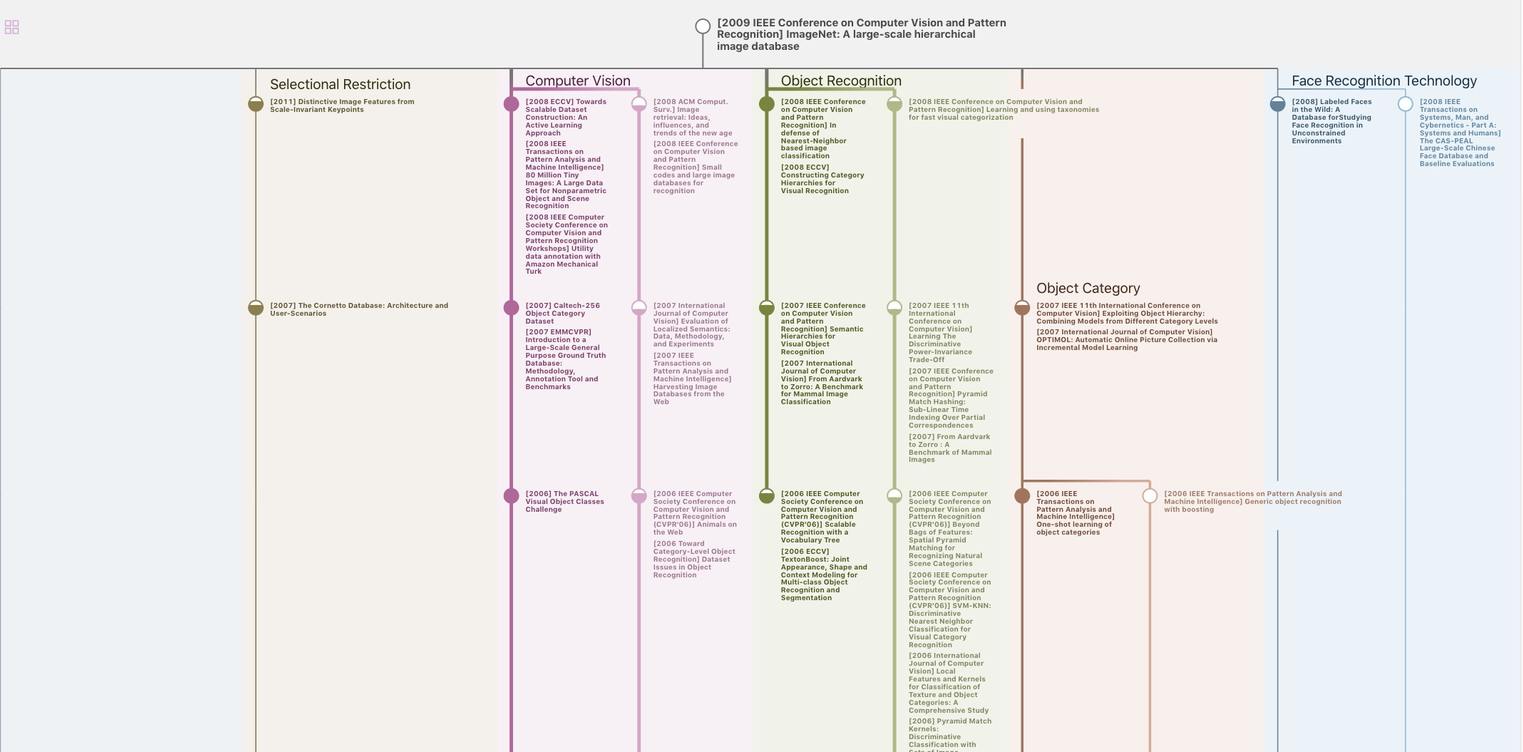
生成溯源树,研究论文发展脉络
Chat Paper
正在生成论文摘要