Deep Neural Network to Curate LTR Retrotransposon Libraries from Plant Genomes
PRACTICAL APPLICATIONS OF COMPUTATIONAL BIOLOGY & BIOINFORMATICS, PACBB 2021(2022)
摘要
Transposable elements are mobile sequences in all eukaryotic genomes. LTR (Long Terminal Repeat) retrotransposons are the most abundant elements in plant genomes where they play a fundamental role in evolution, gene function and genetic diversity. It is therefore important to develop bioinformatic tools to identify them in sequenced genomes and to classify them, taking into account that over time these elements may undergo deletions, insertions or recombination, generating incomplete and inactive elements, which are no longer considered a valid reference for identification and classification studies. LTR retrotransposons play fundamental roles in evolution and genetic diversity, hence the importance of understanding their function and studying in depth the variations that they may present. With the increase of whole genome sequencing, it is necessary to automate the analysis process and reduce the execution time, and to develop more advanced tools. Here, we propose an automatic curator of plant LTR retrotransposons libraries, based on Deep Learning (DL), in which a percentage F1-score of 91.18% was obtained for the test dataset. Generalization tests using four different genomes were performed, obtaining the best results for Oryza granulata, with a performance of 93.6% F1-score, and with an execution time of 22.61 seconds for the prediction by the neural network, using LTR retrotransposons obtained with the LTR_STRUC software. Taking into account that the conventional bioinformatics methods require a time of approximately six hours to curate the same genome, we conclude that our proposed method is efficient and can speed up the curation of libraries of LTR retrotransposons of plants genomes published in massive sequencing projects.
更多查看译文
关键词
LTR retrotransposons, Curation, Nesting insertions, Bioinformatics, Machine learning, Deep neural networks, k-mer-based methods
AI 理解论文
溯源树
样例
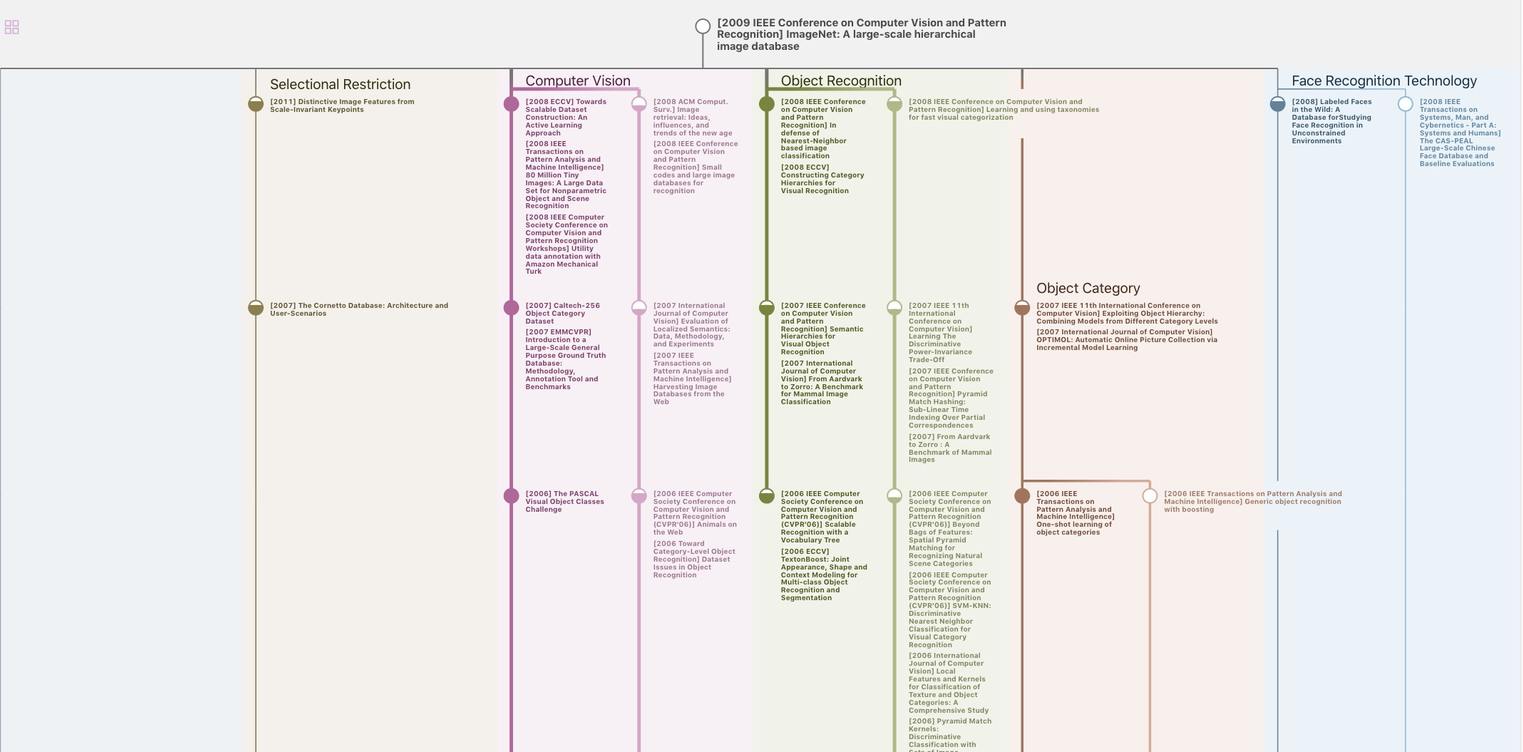
生成溯源树,研究论文发展脉络
Chat Paper
正在生成论文摘要