Automated detection of severe diabetic retinopathy using deep learning method
Graefe's Archive for Clinical and Experimental Ophthalmology(2021)
摘要
Purpose The purpose of this study is to develop and validate the intelligent diagnosis of severe DR with lesion recognition based on color fundus photography. Methods The Kaggle public dataset for DR grading is used in the project, including 53,576 fundus photos in the test set, 28,101 in the training set, and 7,025 in the validation set. We randomly select 4,192 images for lesion annotation. Inception V3 structure is adopted as the classification algorithm. Both 299 × 299 pixel images and 896 × 896 pixel images are used as the input size. ROC curve, AUC, sensitivity, specificity, and their harmonic mean are used to evaluate the performance of the models. Results The harmonic mean and AUC of the model of 896 × 896 input are higher than those of the 299 × 299 input model. The sensitivity, specificity, harmonic mean, and AUC of the method with 896 × 896 resolution images as input for severe DR are 0.925, 0.907, 0.916, and 0.968, respectively. The prediction error mainly occurs in moderate NPDR, and cases with more hard exudates and cotton wool spots are easily predicted as severe cases. Cases with preretinal hemorrhage and vitreous hemorrhage are easily identified as severe cases, and IRMA is the most difficult lesion to recognize. Conclusions We have studied the intelligent diagnosis of severe DR based on color fundus photography. This artificial intelligence–based technology offers a possibility to increase the accessibility and efficiency of severe DR screening.
更多查看译文
关键词
Severe diabetic retinopathy,Color fundus photography,Deep learning,Intraretinal microvascular abnormality
AI 理解论文
溯源树
样例
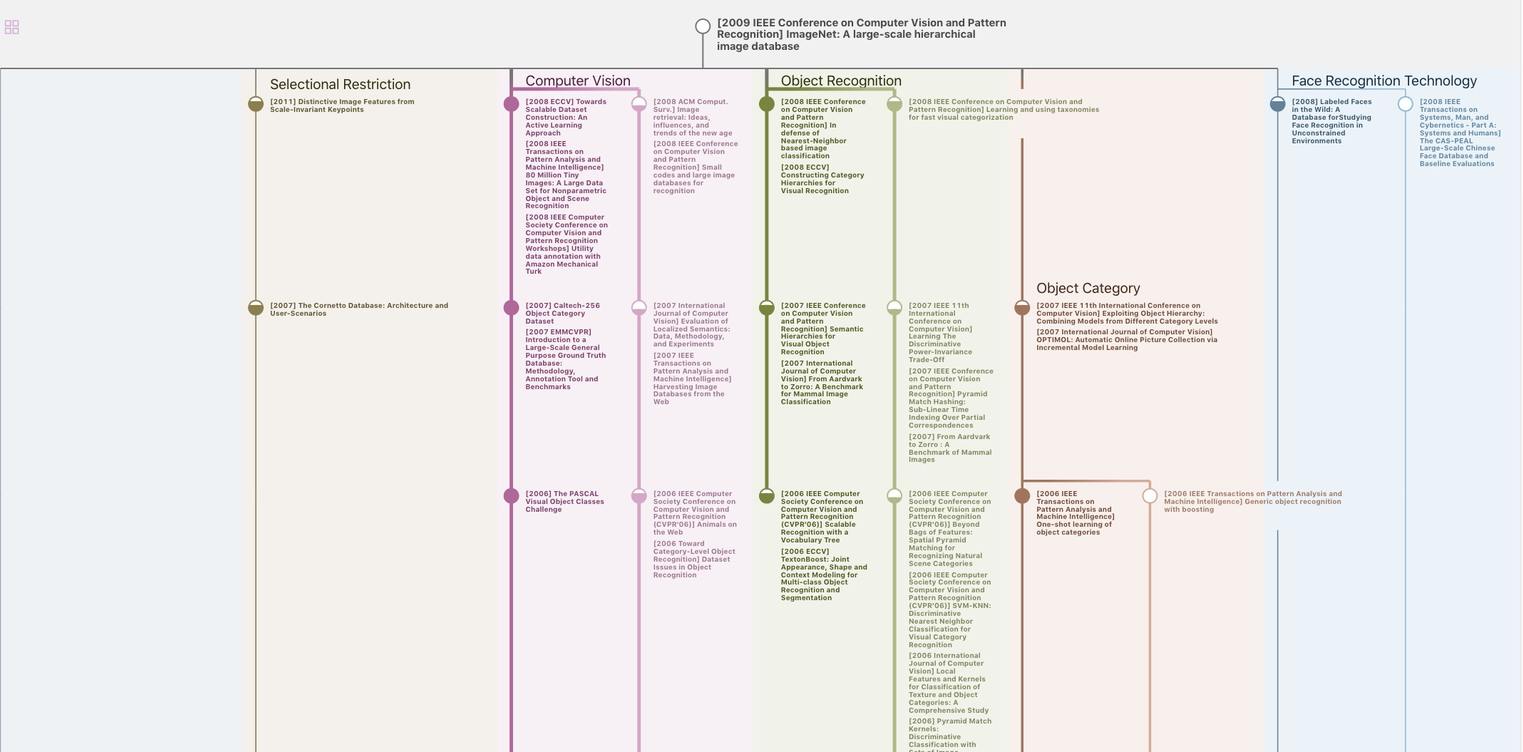
生成溯源树,研究论文发展脉络
Chat Paper
正在生成论文摘要