Multi-Task Pre-Training for Plug-and-Play Task-Oriented Dialogue System
PROCEEDINGS OF THE 60TH ANNUAL MEETING OF THE ASSOCIATION FOR COMPUTATIONAL LINGUISTICS (ACL 2022), VOL 1: (LONG PAPERS)(2022)
摘要
Pre-trained language models have been recently shown to benefit task-oriented dialogue (TOD) systems. Despite their success, existing methods often formulate this task as a cascaded generation problem which can lead to error accumulation across different sub-tasks and greater data annotation overhead. In this study, we present PPTOD, a unified plug-and-play model for task-oriented dialogue. In addition, we introduce a new dialogue multi-task pre-training strategy that allows the model to learn the primary TOD task completion skills from heterogeneous dialog corpora. We extensively test our model on three benchmark TOD tasks, including end-to-end dialogue modelling, dialogue state tracking, and intent classification. Experimental results show that PPTOD achieves new state of the art on all evaluated tasks in both high-resource and low-resource scenarios. Furthermore, comparisons against previous SOTA methods show that the responses generated by PPTOD are more factually correct and semantically coherent as judged by human annotators.(1)
更多查看译文
关键词
dialogue,multi-task,pre-training,plug-and-play,task-oriented
AI 理解论文
溯源树
样例
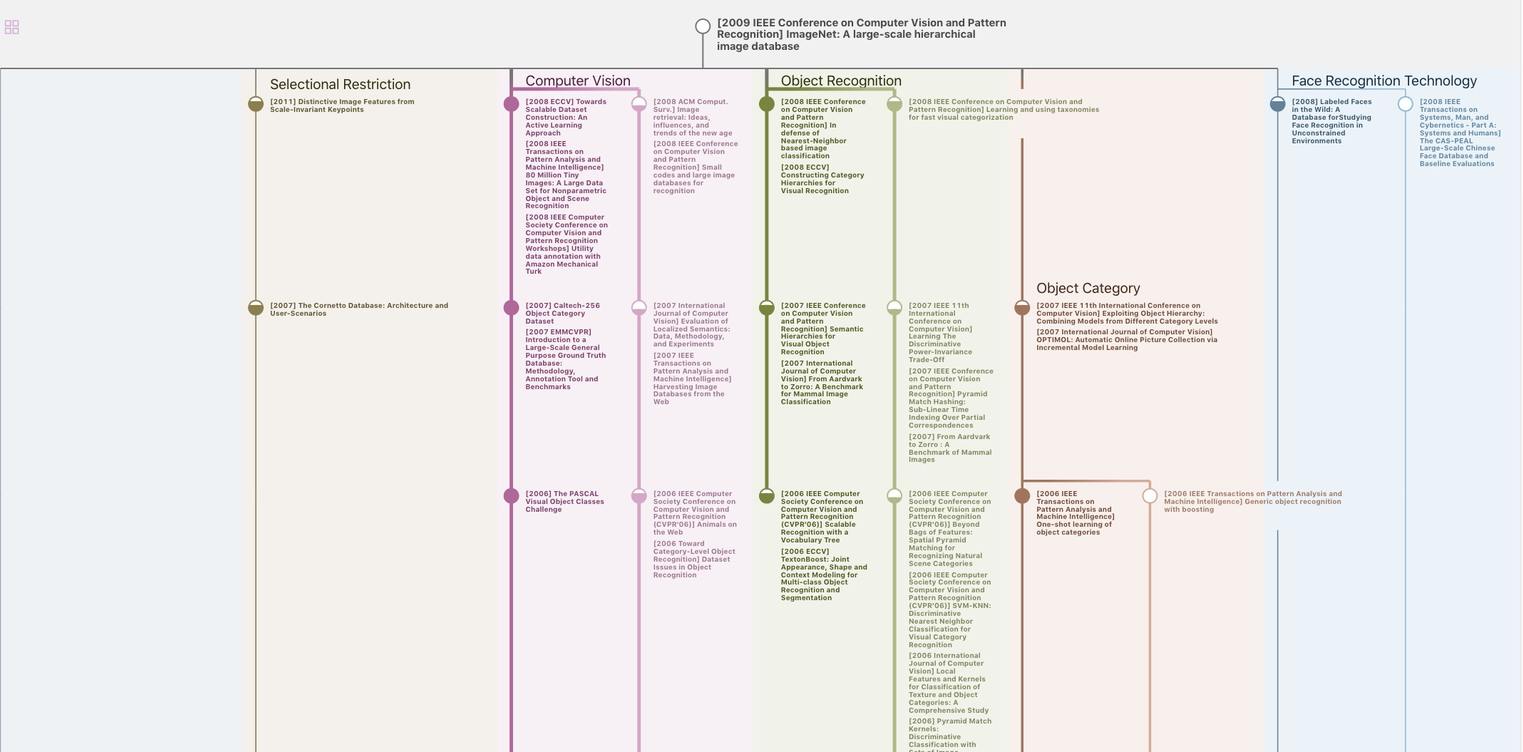
生成溯源树,研究论文发展脉络
Chat Paper
正在生成论文摘要