Biomedical Named Entity Recognition via Knowledge Guidance and Question Answering
ACM Transactions on Computing for Healthcare(2021)
摘要
AbstractIn this work, we formulated the named entity recognition (NER) task as a multi-answer knowledge guided question-answer task (KGQA) and showed that the knowledge guidance helps to achieve state-of-the-art results for 11 of 18 biomedical NER datasets. We prepended five different knowledge contexts—entity types, questions, definitions, and examples—to the input text and trained and tested BERT-based neural models on such input sequences from a combined dataset of the 18 different datasets. This novel formulation of the task (a) improved named entity recognition and illustrated the impact of different knowledge contexts, (b) reduced system confusion by limiting prediction to a single entity-class for each input token (i.e., B, I, O only) compared to multiple entity-classes in traditional NER (i.e., Bentity1, Bentity2, Ientity1, I, O), (c) made detection of nested entities easier, and (d) enabled the models to jointly learn NER-specific features from a large number of datasets. We performed extensive experiments of this KGQA formulation on the biomedical datasets, and through the experiments, we showed when knowledge improved named entity recognition. We analyzed the effect of the task formulation, the impact of the different knowledge contexts, the multi-task aspect of the generic format, and the generalization ability of KGQA. We also probed the model to better understand the key contributors for these improvements.
更多查看译文
关键词
BERT-CNN,BIO tagging,NER,Named entity recognition,biomedical,multitask training,question answering,text tagging,transfer learning
AI 理解论文
溯源树
样例
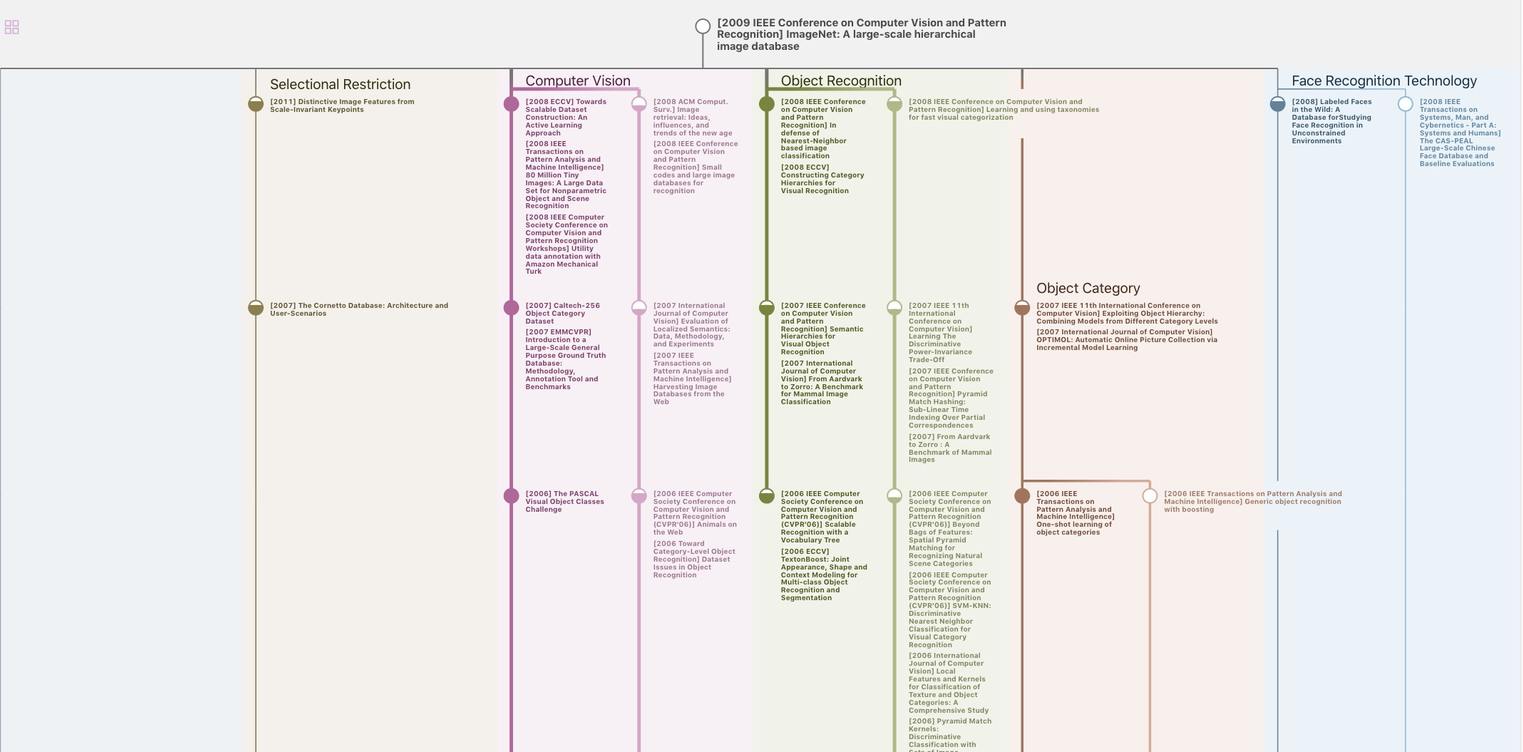
生成溯源树,研究论文发展脉络
Chat Paper
正在生成论文摘要