Attribute Subset Selection for Image Recognition. Random Forest Under Assessment
16TH INTERNATIONAL CONFERENCE ON SOFT COMPUTING MODELS IN INDUSTRIAL AND ENVIRONMENTAL APPLICATIONS (SOCO 2021)(2022)
摘要
This contribution presents an approach to take advantage of correlation and consistency measures in attribute subset selection for image recognition. The proposal has been evaluated on ten multiple class problems with up to sixty attributes, ten labels and around six thousands of samples. We focus on Random Forest due to its good performance. Two performance measures such as Cohen's kappa and Area Under receiver operating characteristic Curve (AUC) are reported. They have been averaged on thirty runs with different seeds. Additionally, the computational cost is presented. The results are very satisfactory and show the approach to be more accurate than its competitors. As an important conclusion we may state that it is worth applying directly the new proposal without devoting time to score the two supporting methods which are the basement of the approach; the overhead, over the slowest initial case and, according to the empirical results, probably the best in predictive terms, is around a ten per cent measured in seconds or in number of additional attributes.
更多查看译文
关键词
Data selection, Attribute subset selection, Data mining, Random forest
AI 理解论文
溯源树
样例
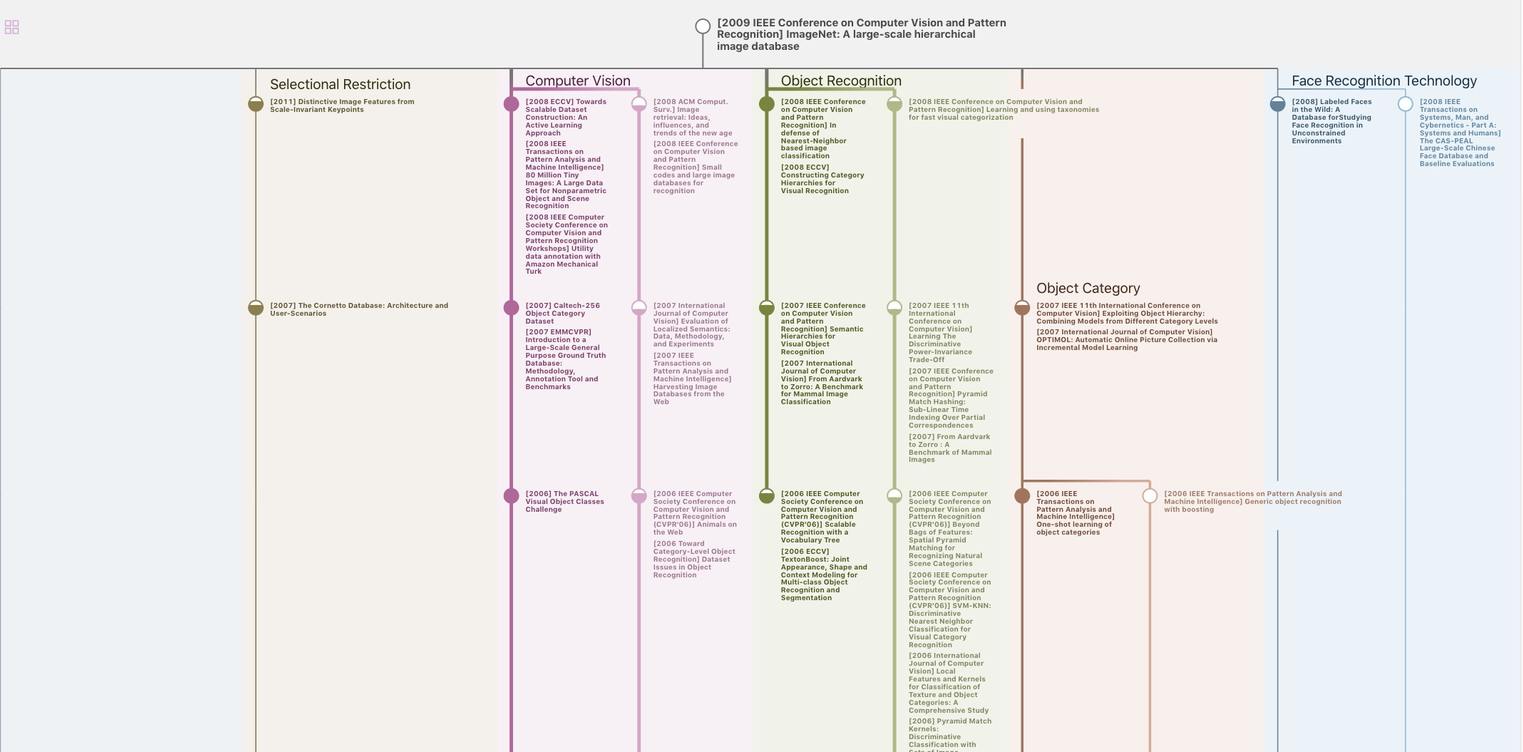
生成溯源树,研究论文发展脉络
Chat Paper
正在生成论文摘要