A Novel Methodology for the Characterization of Cutting Conditions in Turning Processes Using Machine Learning Models and Acoustic Emission Signals
16TH INTERNATIONAL CONFERENCE ON SOFT COMPUTING MODELS IN INDUSTRIAL AND ENVIRONMENTAL APPLICATIONS (SOCO 2021)(2022)
摘要
In the last few years, the industry requires to know in real-time the condition of their assets. Acoustic Emission (AE) technique has been widely used to understand the real-time condition of manufacturing processes such as the degree of tool wear or tool breakage. Traditionally, to fulfil that goal, the information extracted from the signal sensors of the machines has been processed with mathematical models. This methodology is changing, and instead of developing complex physical models (where an in-depth knowledge of the system being modelled is required), the current trend is to use Machine Learning (ML) models which are based on previous data. Signal pre-processing and feature extraction is a complex task that usually generates a high amount of predicting variables. Therefore, this paper proposes a methodology to identify the best pre-processing tools, AE features and ML models to characterize cutting condition processes. This methodology is validated identifying cutting conditions in a turning process based on AE signals. To classify the cutting condition with the highest accuracy, several techniques are applied, (including wavelet transform for multiresolution analysis, Recursive Feature Elimination (RFE) technique, different classifiers (Decision Tree (DT), Random Forests (RF), Support Vector Machine (SVM), Gaussian Process (GP), K-Nearest Neighbor (KNN) and Multilayer Perceptron (MLP) classifiers) and different signal segmentation lengths. These techniques were evaluated using the data captured in a turning process when cutting a 19NiMoCr6 steel under pre-established cutting conditions. The best accuracy of predicting the cutting conditions based on AE signals was 99.7%, and it was achieved combining the wavelet packet transform (WPT) with RFE, with a segmentation time of 0.05 s and RF as classifier.
更多查看译文
关键词
Machine learning, Acoustic emission, Cutting characterization, Wavelet transform, Recursive feature elimination
AI 理解论文
溯源树
样例
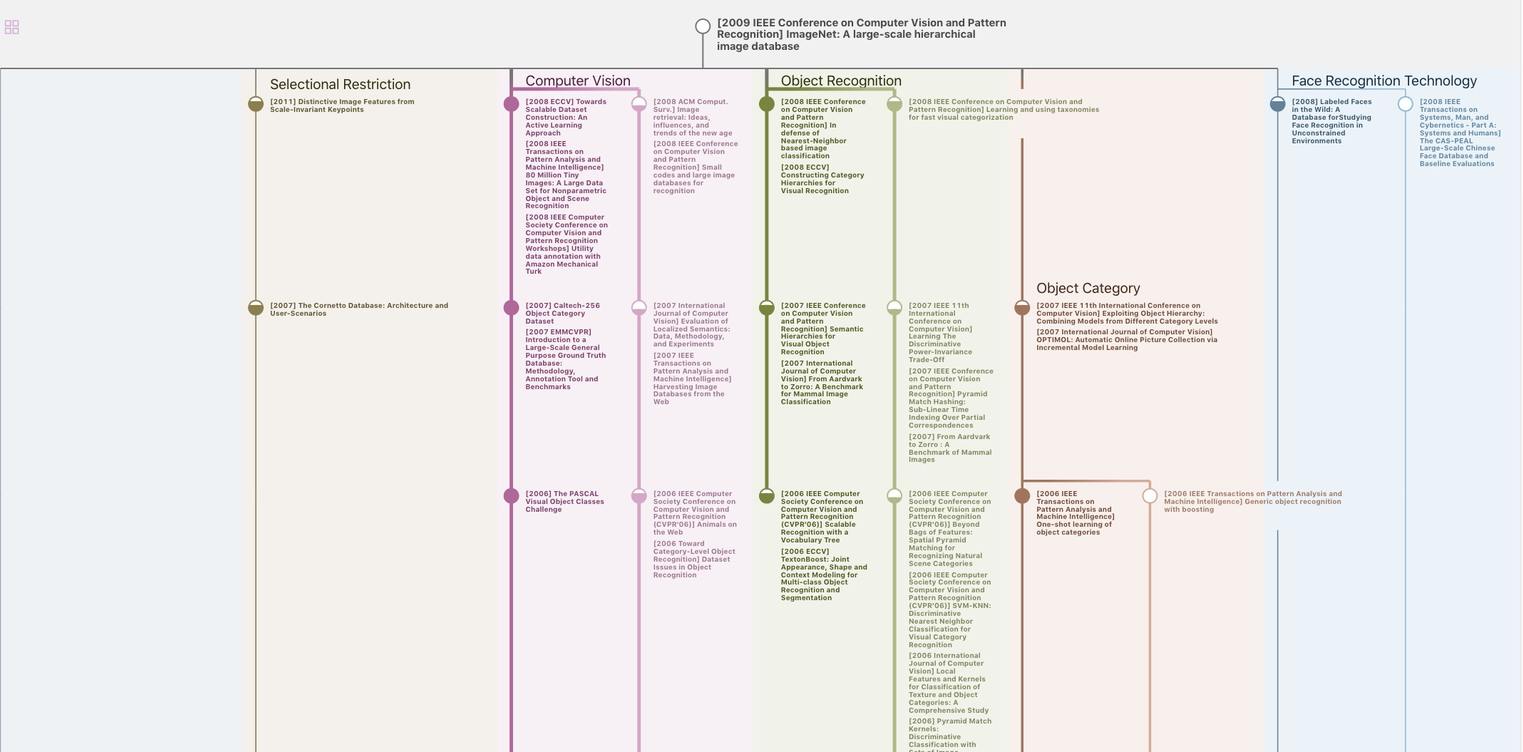
生成溯源树,研究论文发展脉络
Chat Paper
正在生成论文摘要