GeoDualCNN: Geometry-Supporting Dual Convolutional Neural Network for Noisy Point Clouds
IEEE Transactions on Visualization and Computer Graphics(2023)
摘要
We propose a geometry-supporting dual convolutional neural network (GeoDualCNN) for both point cloud normal estimation and denoising. GeoDualCNN fuses the geometry domain knowledge that the underlying surface of a noisy point cloud is piecewisely smooth with the fact that a point normal is properly defined only when local surface smoothness is guaranteed. Centered around this insight, we define the homogeneous neighborhood (HoNe) which stays clear of surface discontinuities, and associate each HoNe with a point whose geometry and normal orientation is mostly consistent with that of HoNe. Thus, we not only obtain initial estimates of the point normals by performing PCA on HoNes, but also for the first time optimize these initial point normals by learning the mapping from two proposed geometric descriptors to the ground-truth point normals. GeoDualCNN consists of two parallel branches that remove noise using the first geometric descriptor (a
homogeneous height map
, which encodes the point-position information), while preserving surface features using the second geometric descriptor (a
homogeneous normal map
, which encodes the point-normal information). Such geometry-supporting network architectures enable our model to leverage previous geometry expertise and to benefit from training data. Experiments with noisy point clouds show that GeoDualCNN outperforms the state-of-the-art methods in terms of both noise-robustness and feature preservation.
更多查看译文
关键词
GeoDualCNN,normal estimation,point cloud denoising,geometry domain knowledge,neural network
AI 理解论文
溯源树
样例
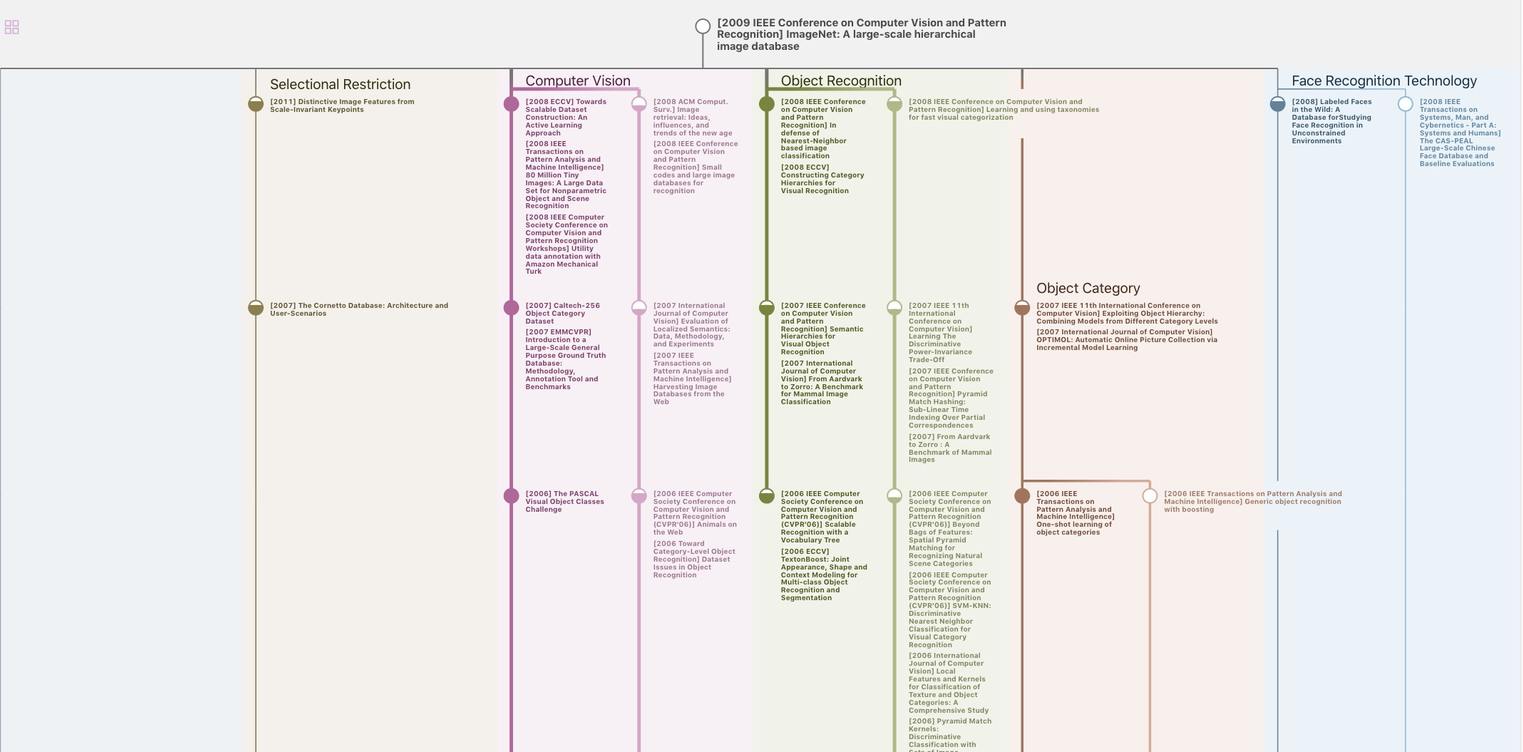
生成溯源树,研究论文发展脉络
Chat Paper
正在生成论文摘要