Solving Challenging Control Problems Using Two-Staged Deep Reinforcement Learning
arxiv(2023)
摘要
We present a deep reinforcement learning (deep RL) algorithm that consists of learning-based motion planning and imitation to tackle challenging control problems. Deep RL has been an effective tool for solving many high-dimensional continuous control problems, but it cannot effectively solve challenging problems with certain properties, such as sparse reward functions or sensitive dynamics. In this work, we propose an approach that decomposes the given problem into two deep RL stages: motion planning and motion imitation. The motion planning stage seeks to compute a feasible motion plan by leveraging the powerful planning capability of deep RL. Subsequently, the motion imitation stage learns a control policy that can imitate the given motion plan with realistic sensors and actuation models. This new formulation requires only a nominal added cost to the user because both stages require minimal changes to the original problem. We demonstrate that our approach can solve challenging control problems, rocket navigation, and quadrupedal locomotion, which cannot be solved by the monolithic deep RL formulation or the version with Probabilistic Roadmap.
更多查看译文
关键词
challenging control problems,deep reinforcement
AI 理解论文
溯源树
样例
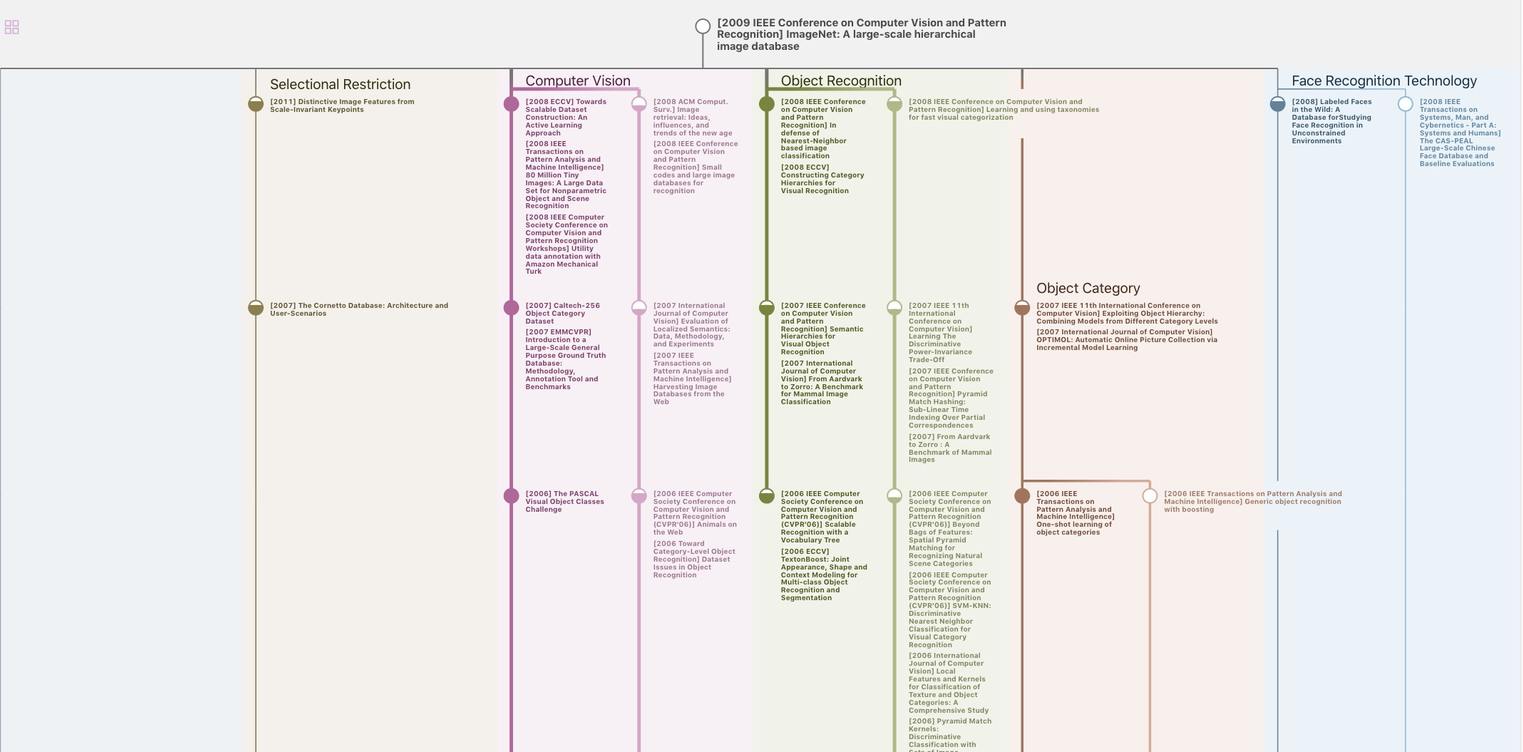
生成溯源树,研究论文发展脉络
Chat Paper
正在生成论文摘要