LOS: Local-Optimistic Scheduling of Periodic Model Training For Anomaly Detection on Sensor Data Streams in Meshed Edge Networks
2021 IEEE International Conference on Autonomic Computing and Self-Organizing Systems (ACSOS)(2021)
摘要
Anomaly detection is increasingly important to handle the amount of sensor data in Edge and Fog environments, Smart Cities, as well as in Industry 4.0. To ensure good results, the utilized ML models need to be updated periodically to adapt to seasonal changes and concept drifts in the sensor data. Although the increasing resource availability at the edge can allow for in-situ execution of model training directly on the devices, it is still often offloaded to fog devices or the cloud. In this paper, we propose Local-Optimistic Scheduling (LOS), a method for executing periodic ML model training jobs in close proximity to the data sources, without overloading lightweight edge devices. Training jobs are offloaded to nearby neighbor nodes as necessary and the resource consumption is optimized to meet the training period while still ensuring enough resources for further training executions. This scheduling is accomplished in a decentralized, collaborative and opportunistic manner, without full knowledge of the infrastructure and workload. We evaluated our method in an edge computing testbed on real-world datasets. The experimental results show that LOS places the training executions close to the input sensor streams, decreases the deviation between training time and training period by up to 40% and increases the amount of successfully scheduled training jobs compared to an in-situ execution.
更多查看译文
关键词
Edge computing,autonomic resource management,ad-hoc networks,decentralized scheduling,anomaly detection
AI 理解论文
溯源树
样例
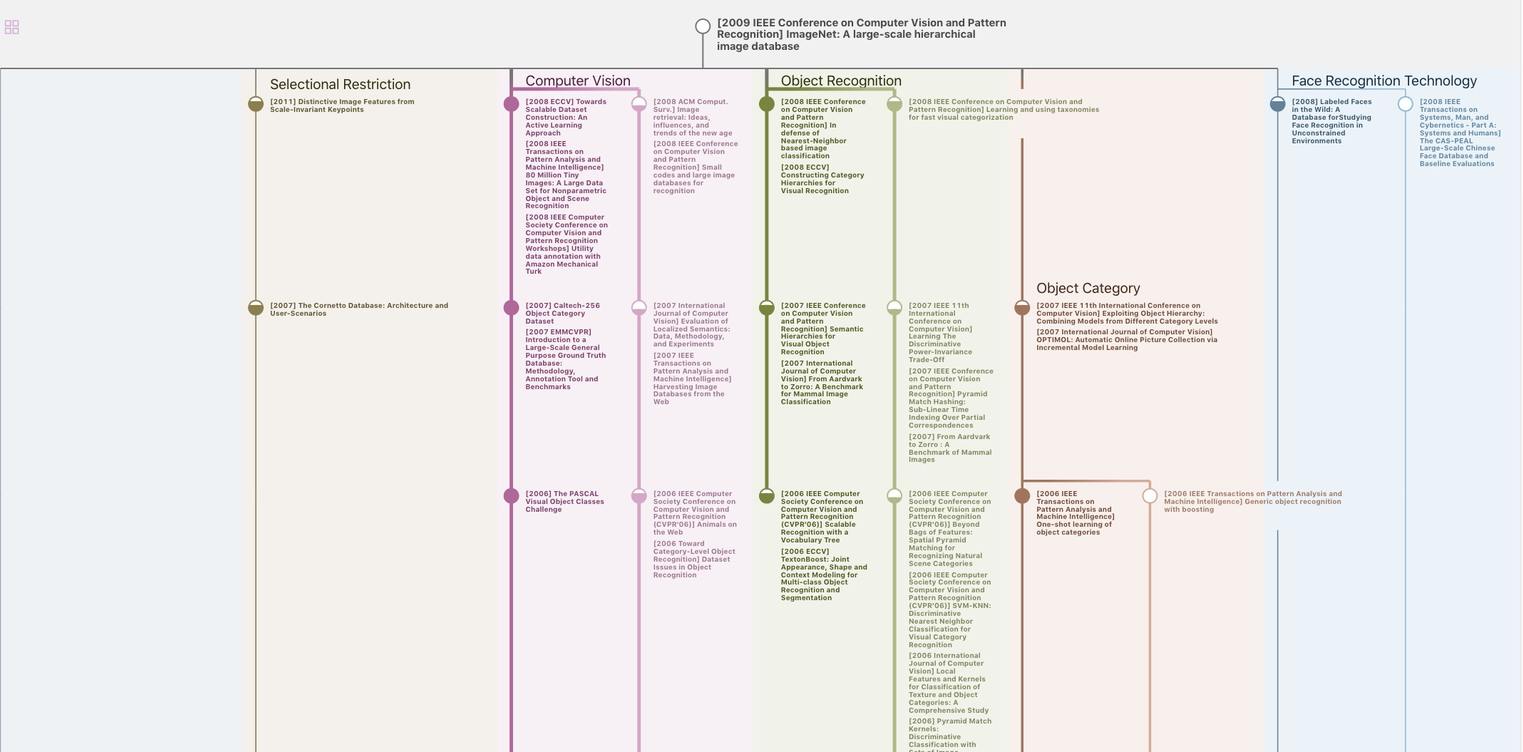
生成溯源树,研究论文发展脉络
Chat Paper
正在生成论文摘要