Unbiased Gradient Estimation with Balanced Assignments for Mixtures of Experts
arxiv(2021)
摘要
Training large-scale mixture of experts models efficiently on modern hardware requires assigning datapoints in a batch to different experts, each with a limited capacity. Recently proposed assignment procedures lack a probabilistic interpretation and use biased estimators for training. As an alternative, we propose two unbiased estimators based on principled stochastic assignment procedures: one that skips datapoints which exceed expert capacity, and one that samples perfectly balanced assignments using an extension of the Gumbel-Matching distribution [29]. Both estimators are unbiased, as they correct for the used sampling procedure. On a toy experiment, we find the `skip'-estimator is more effective than the balanced sampling one, and both are more robust in solving the task than biased alternatives.
更多查看译文
关键词
mixtures,experts,gradient,estimation,balanced assignments
AI 理解论文
溯源树
样例
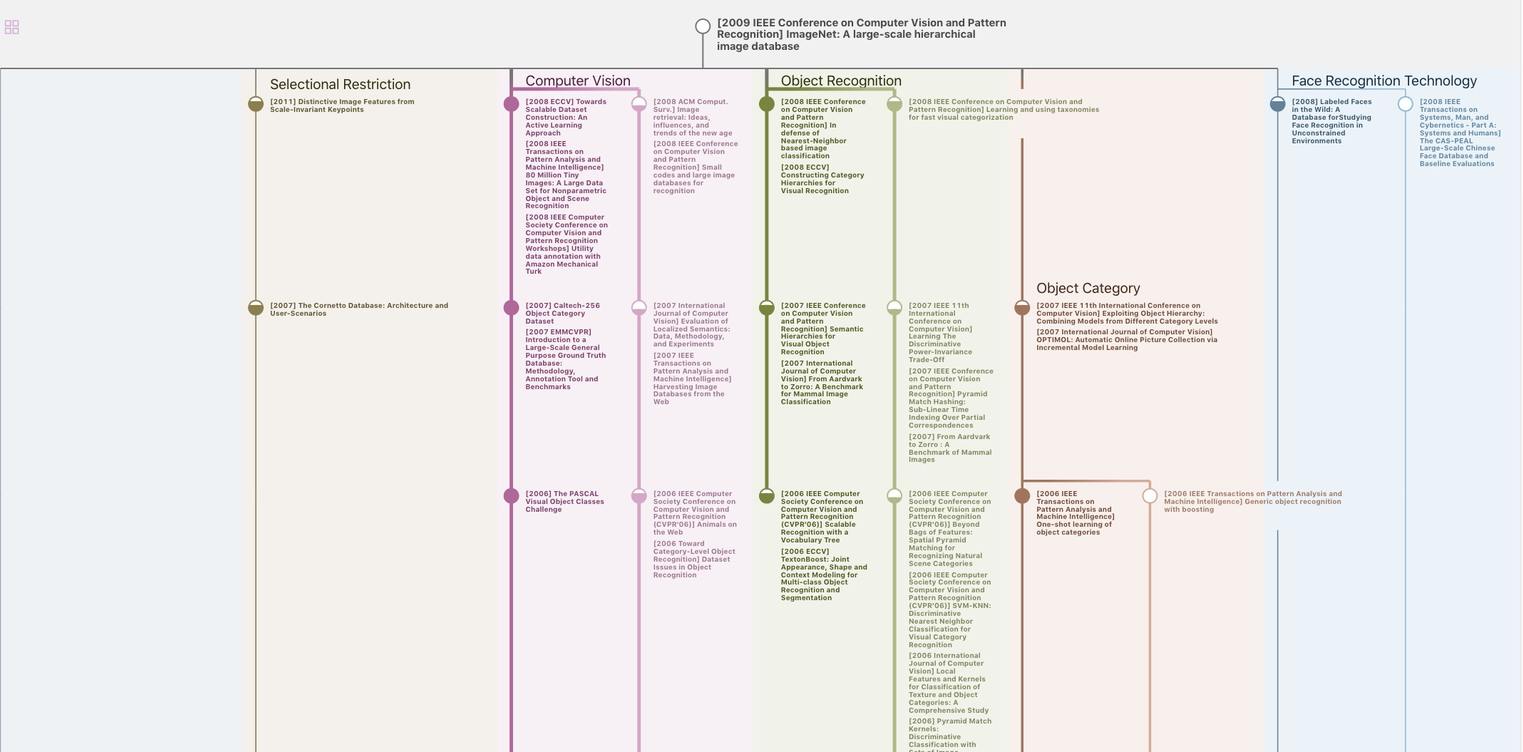
生成溯源树,研究论文发展脉络
Chat Paper
正在生成论文摘要