Recurrent neural networks for partially observed dynamical systems
PHYSICAL REVIEW E(2022)
摘要
Complex nonlinear dynamics are ubiquitous in many fields. Moreover, we rarely have access to all of the relevant state variables governing the dynamics. Delay embedding allows us, in principle, to account for unobserved state variables. Here we provide an algebraic approach to delay embedding that permits explicit approximation of error. We also provide the asymptotic dependence of the first-order approximation error on the system size. More importantly, this formulation of delay embedding can be directly implemented using a recurrent neural network (RNN). This observation expands the interpretability of both delay embedding and the RNN and facilitates principled incorporation of structure and other constraints into these approaches.
更多查看译文
AI 理解论文
溯源树
样例
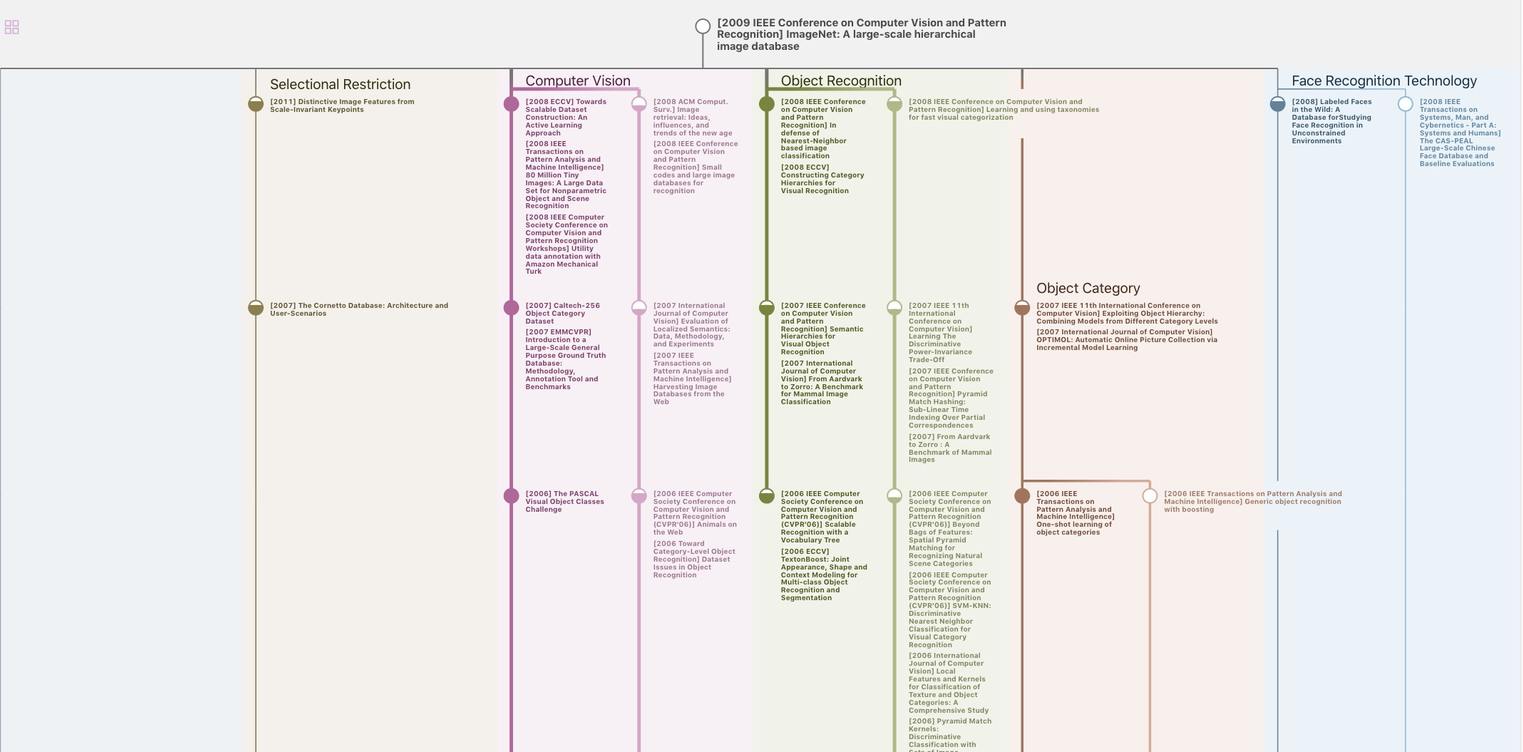
生成溯源树,研究论文发展脉络
Chat Paper
正在生成论文摘要