FINN.no Slates Dataset: A new Sequential Dataset Logging Interactions, all Viewed Items and Click Responses/No-Click for Recommender Systems Research
RECSYS(2021)
摘要
We present a novel recommender systems dataset that records the sequential interactions between users and an online marketplace. The users are sequentially presented with both recommendations and search results in the form of ranked lists of items, called slates, from the marketplace. The dataset includes the presented slates at each round, whether the user clicked on any of these items and which item the user clicked on. Although the usage of exposure data in recommender systems is growing, to our knowledge there is no open large-scale recommender systems dataset that includes the slates of items presented to the users at each interaction. As a result, most articles on recommender systems do not utilize this exposure information. Instead, the proposed models only depend on the user's click responses, and assume that the user is exposed to all the items in the item universe at each step, often called uniform candidate sampling. This is an incomplete assumption, as it takes into account items the user might not have been exposed to. This way items might be incorrectly considered as not of interest to the user. Taking into account the actually shown slates allows the models to use a more natural likelihood, based on the click probability given the exposure set of items, as is prevalent in the bandit and reinforcement learning literature. \cite{Eide2021DynamicSampling} shows that likelihoods based on uniform candidate sampling (and similar assumptions) are implicitly assuming that the platform only shows the most relevant items to the user. This causes the recommender system to implicitly reinforce feedback loops and to be biased towards previously exposed items to the user.
更多查看译文
关键词
slate recommendations, search result, candidate sampling, marketplace data, reinforcement learning, bandit, item attributes, off-policy
AI 理解论文
溯源树
样例
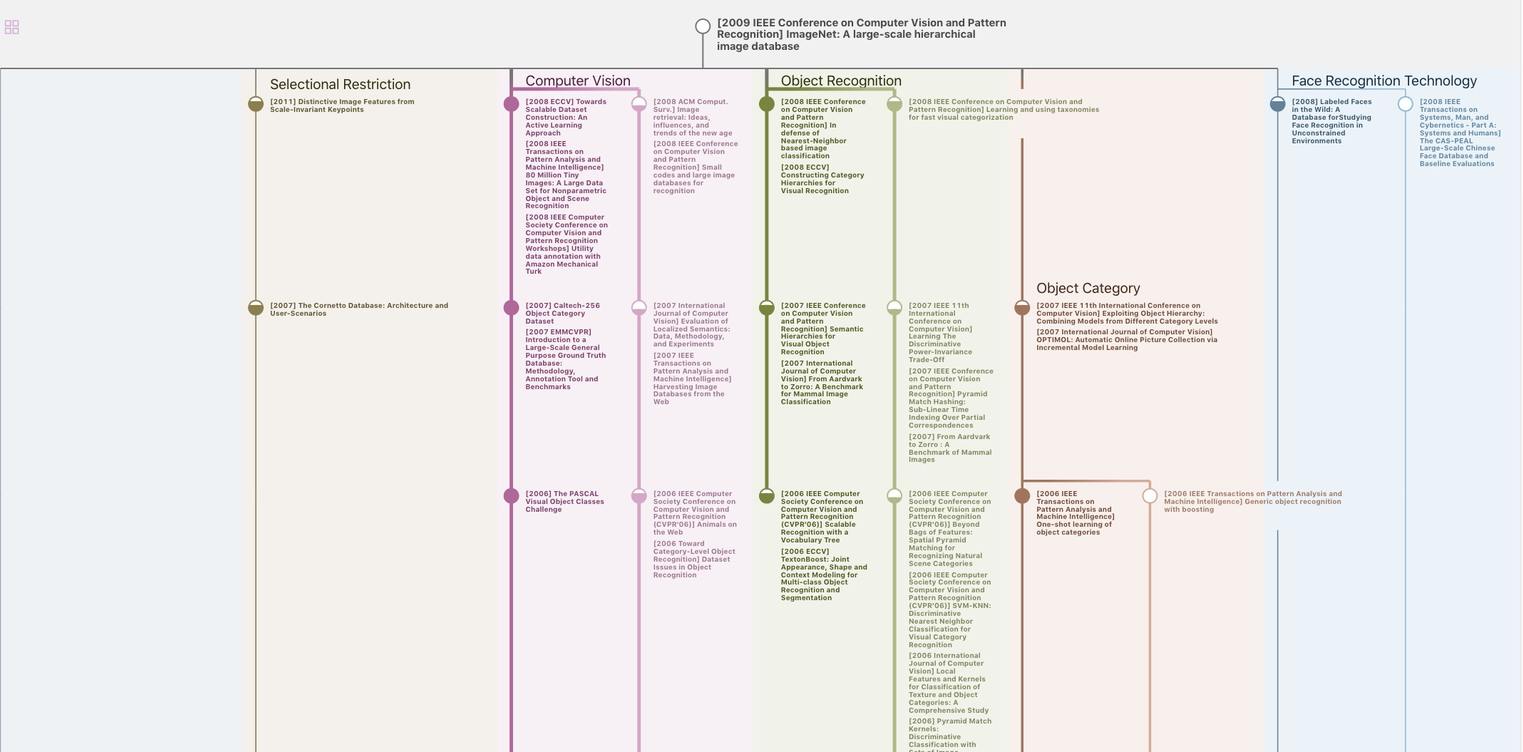
生成溯源树,研究论文发展脉络
Chat Paper
正在生成论文摘要