Multi-Source Video Domain Adaptation With Temporal Attentive Moment Alignment Network
arxiv(2023)
摘要
Multi-Source Domain Adaptation (MSDA) is a more practical domain adaptation scenario in real-world scenarios, which relaxes the assumption in conventional Unsupervised Domain Adaptation (UDA) that source data are sampled from a single domain and match a uniform data distribution. The MSDA is more challenging due to the existence of different domain shifts between distinct domain pairs. When considering videos, the negative transfer would be provoked by spatial-temporal features and can be formulated into a more challenging Multi-Source Video Domain Adaptation (MSVDA) problem. In this paper, we address the MSVDA problem by proposing a novel Temporal Attentive Moment Alignment Network (TAMAN) which aims for effective feature transfer by dynamically aligning both spatial and temporal feature moments. The TAMAN further constructs robust global temporal features by attending to dominant domain-invariant local temporal features with high local classification confidence and low disparity between global and local feature discrepancies. To facilitate future research on the MSVDA problem, we introduce comprehensive benchmarks, covering extensive MSVDA scenarios. Empirical results demonstrate a superior performance of the proposed TAMAN across multiple MSVDA benchmarks.
更多查看译文
关键词
temporal attentive moment alignment,adaptation,video,multi-source
AI 理解论文
溯源树
样例
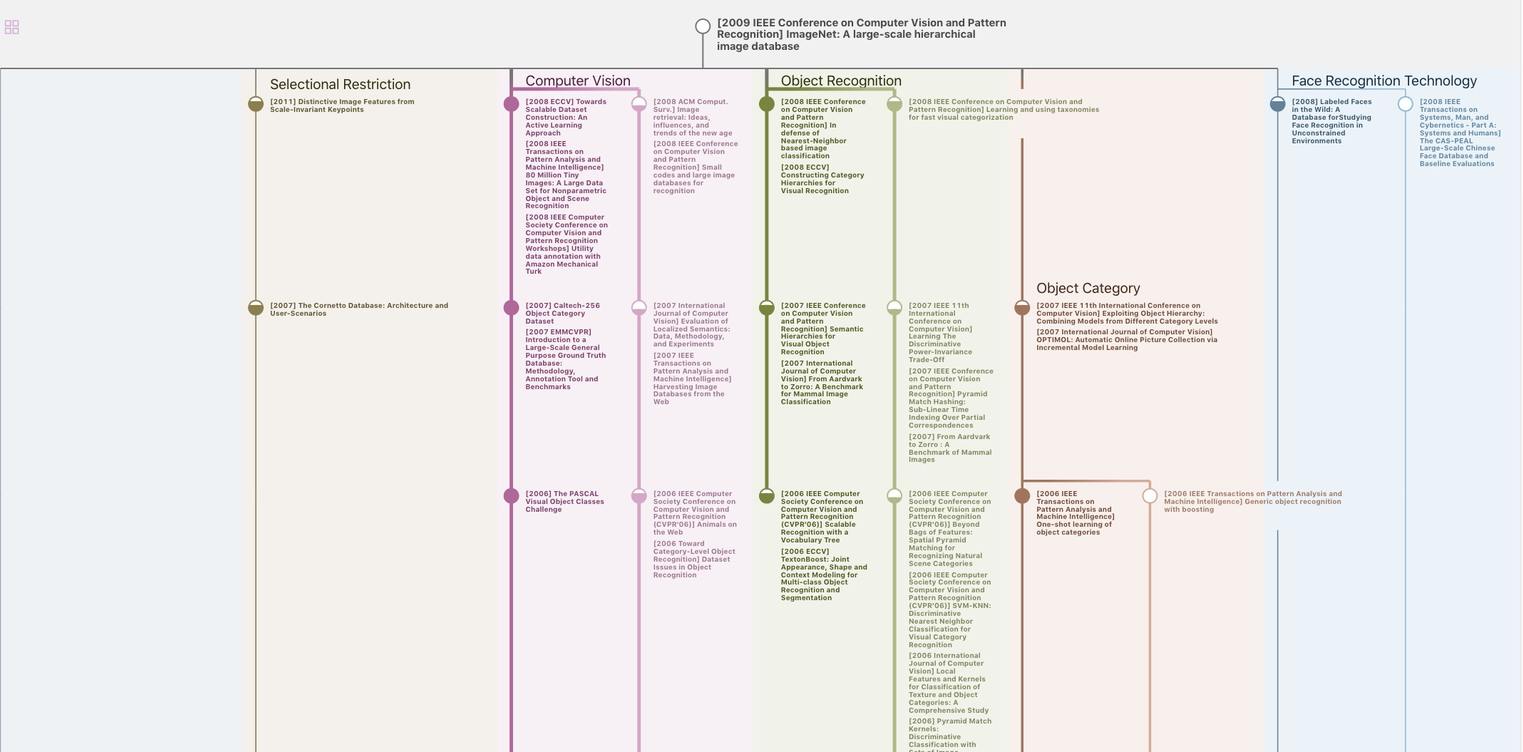
生成溯源树,研究论文发展脉络
Chat Paper
正在生成论文摘要