On Generalization in Coreference Resolution
arxiv(2021)
摘要
While coreference resolution is defined independently of dataset domain, most models for performing coreference resolution do not transfer well to unseen domains. We consolidate a set of 8 coreference resolution datasets targeting different domains to evaluate the off-the-shelf performance of models. We then mix three datasets for training; even though their domain, annotation guidelines, and metadata differ, we propose a method for jointly training a single model on this heterogeneous data mixture by using data augmentation to account for annotation differences and sampling to balance the data quantities. We find that in a zero-shot setting, models trained on a single dataset transfer poorly while joint training yields improved overall performance, leading to better generalization in coreference resolution models. This work contributes a new benchmark for robust coreference resolution and multiple new state-of-the-art results.
更多查看译文
关键词
generalization
AI 理解论文
溯源树
样例
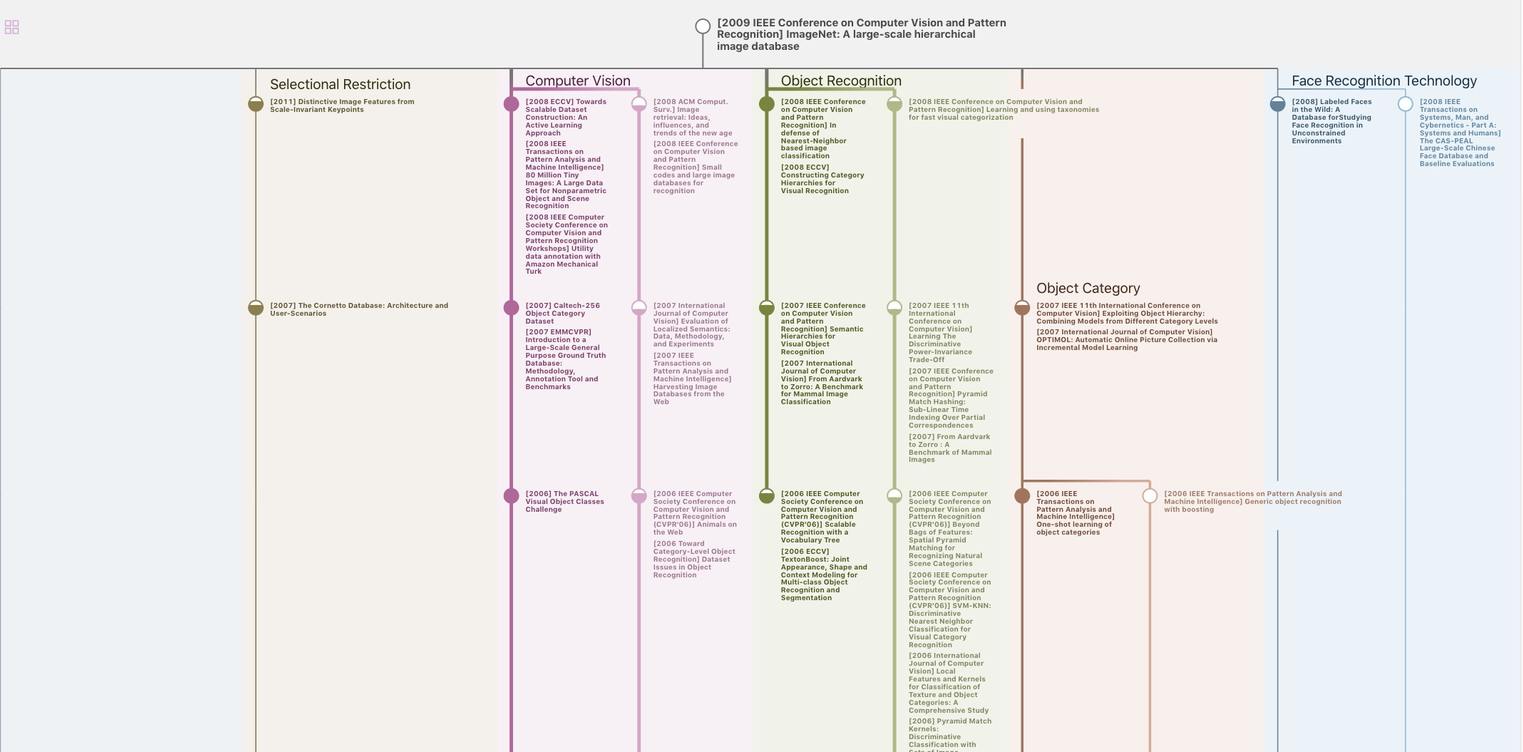
生成溯源树,研究论文发展脉络
Chat Paper
正在生成论文摘要