Non-stationary spatio-temporal point process modeling for high-resolution COVID-19 data
arxiv(2023)
摘要
Most COVID-19 studies commonly report figures of the overall infection at a state- or county-level. This aggregation tends to miss out on fine details of virus propagation. In this paper, we analyze a high-resolution COVID-19 dataset in Cali, Colombia, that records the precise time and location of every confirmed case. We develop a non-stationary spatio-temporal point process equipped with a neural network-based kernel to capture the heterogeneous correlations among COVID-19 cases. The kernel is carefully crafted to enhance expressiveness while maintaining model interpretability. We also incorporate some exogenous influences imposed by city landmarks. Our approach outperforms the state-of-the-art in forecasting new COVID-19 cases with the capability to offer vital insights into the spatio-temporal interaction between individuals concerning the disease spread in a metropolis.
更多查看译文
关键词
spatio-temporal point processes, self-exciting models, nonstationary kernel, neural networks, COVID-19
AI 理解论文
溯源树
样例
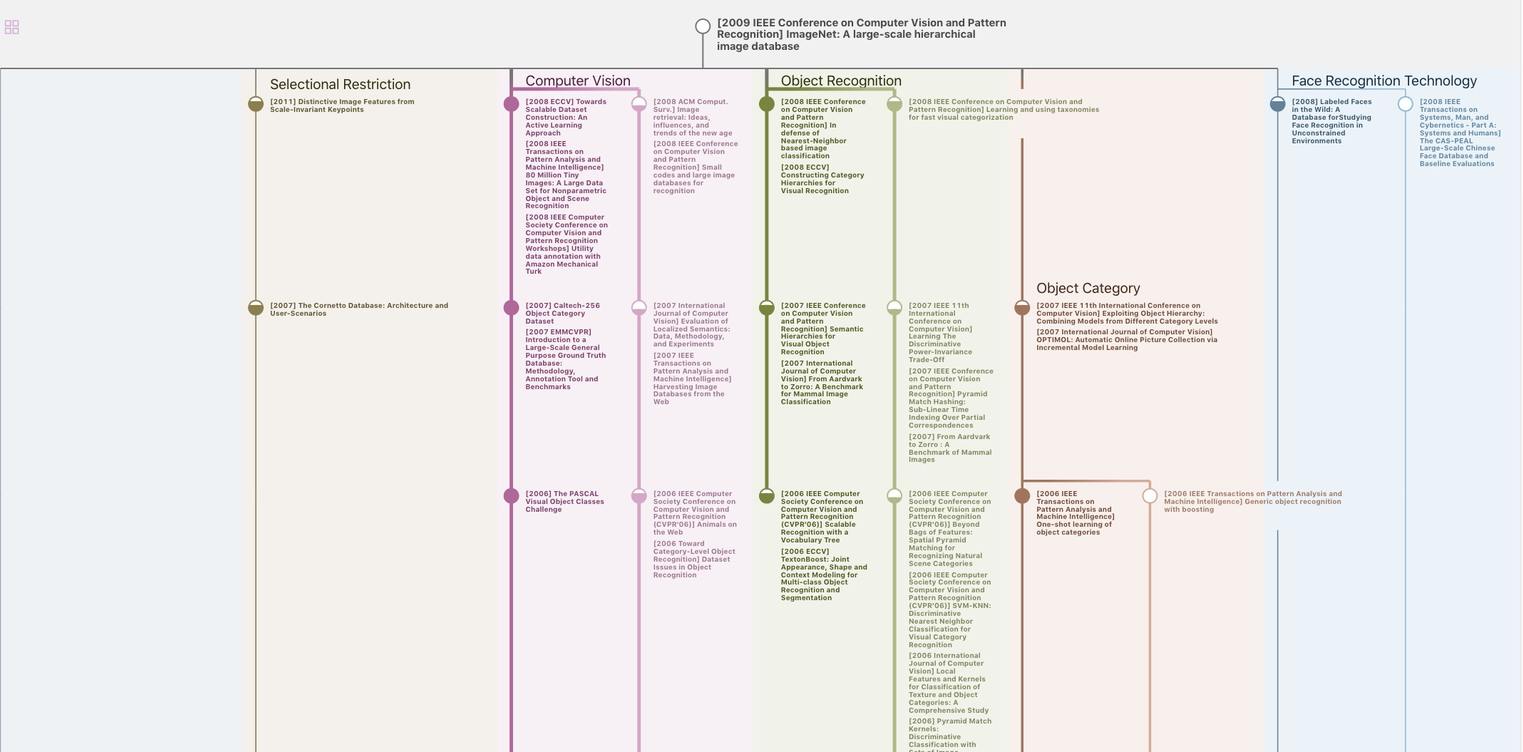
生成溯源树,研究论文发展脉络
Chat Paper
正在生成论文摘要