Adversarial Scrubbing of Demographic Information for Text Classification.
EMNLP(2021)
摘要
Contextual representations learned by language models can often encode undesirable attributes, like demographic associations of the users, while being trained for an unrelated target task. We aim to scrub such undesirable attributes and learn fair representations while maintaining performance on the target task. In this paper, we present an adversarial learning framework "Adversarial Scrubber" (ADS), to debias contextual representations. We perform theoretical analysis to show that our framework converges without leaking demographic information under certain conditions. We extend previous evaluation techniques by evaluating debiasing performance using Minimum Description Length (MDL) probing. Experimental evaluations on 8 datasets show that ADS generates representations with minimal information about demographic attributes while being maximally informative about the target task.
更多查看译文
关键词
demographic information,text classification
AI 理解论文
溯源树
样例
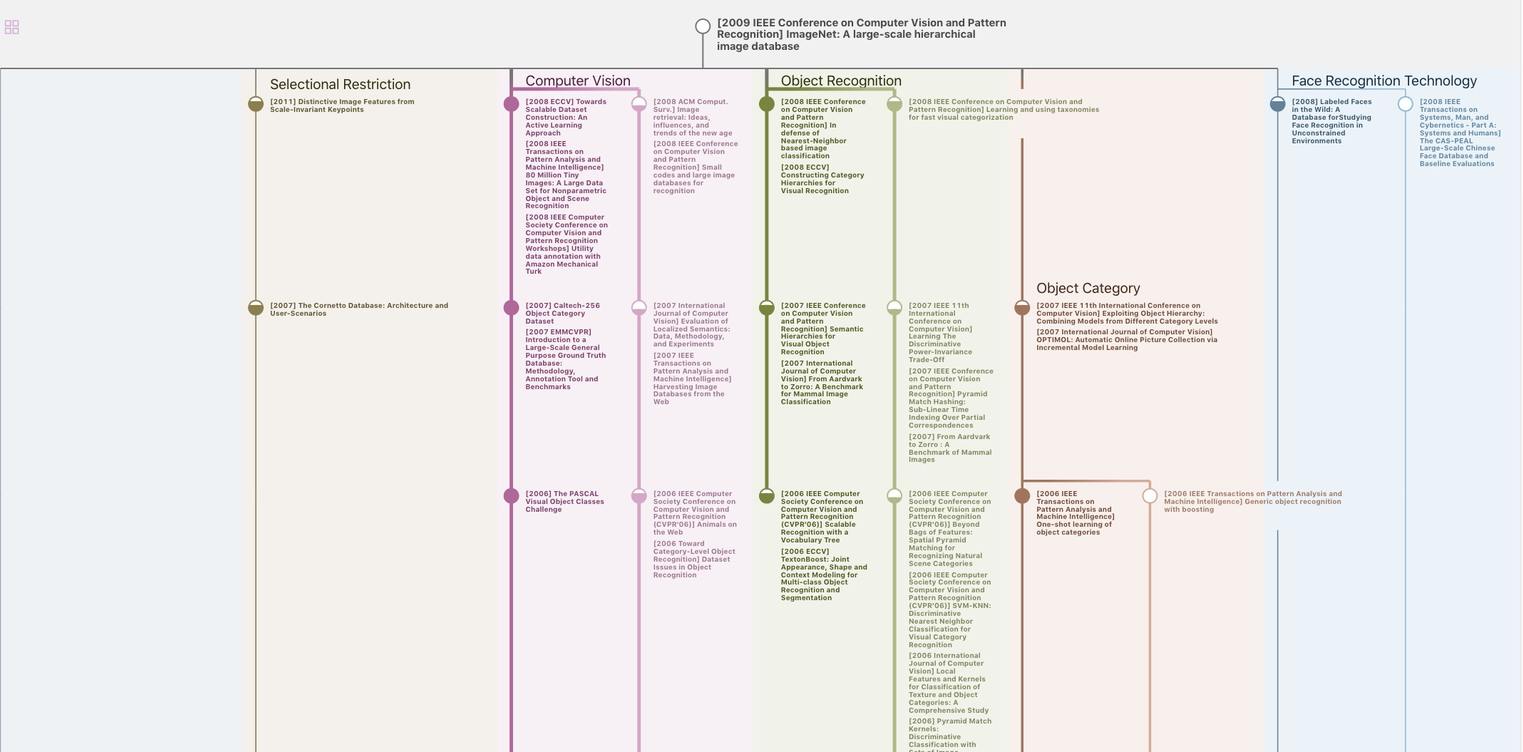
生成溯源树,研究论文发展脉络
Chat Paper
正在生成论文摘要