Inductive Link Prediction with Interactive Structure Learning on Attributed Graph
MACHINE LEARNING AND KNOWLEDGE DISCOVERY IN DATABASES, ECML PKDD 2021: RESEARCH TRACK, PT II(2021)
摘要
Link prediction is one of the most important tasks in graph machine learning, which aims at predicting whether two nodes in a network have an edge. Real-world graphs typically contain abundant node and edge attributes, thus how to perform link prediction by simultaneously learning structure and attribute information from both interactions/paths between two associated nodes and local neighborhood among node's ego subgraph is intractable. To address this issue, we develop a novel Path-aware Graph Neural Network (PaGNN) method for link prediction, which incorporates interaction and neighborhood information into graph neural networks via broadcasting and aggregating operations. And a cache strategy is developed to accelerate the inference process. Extensive experiments show a superior performance of our proposal over state-of-the-art methods on real-world link prediction tasks.
更多查看译文
关键词
interactive structure learning,graph,prediction
AI 理解论文
溯源树
样例
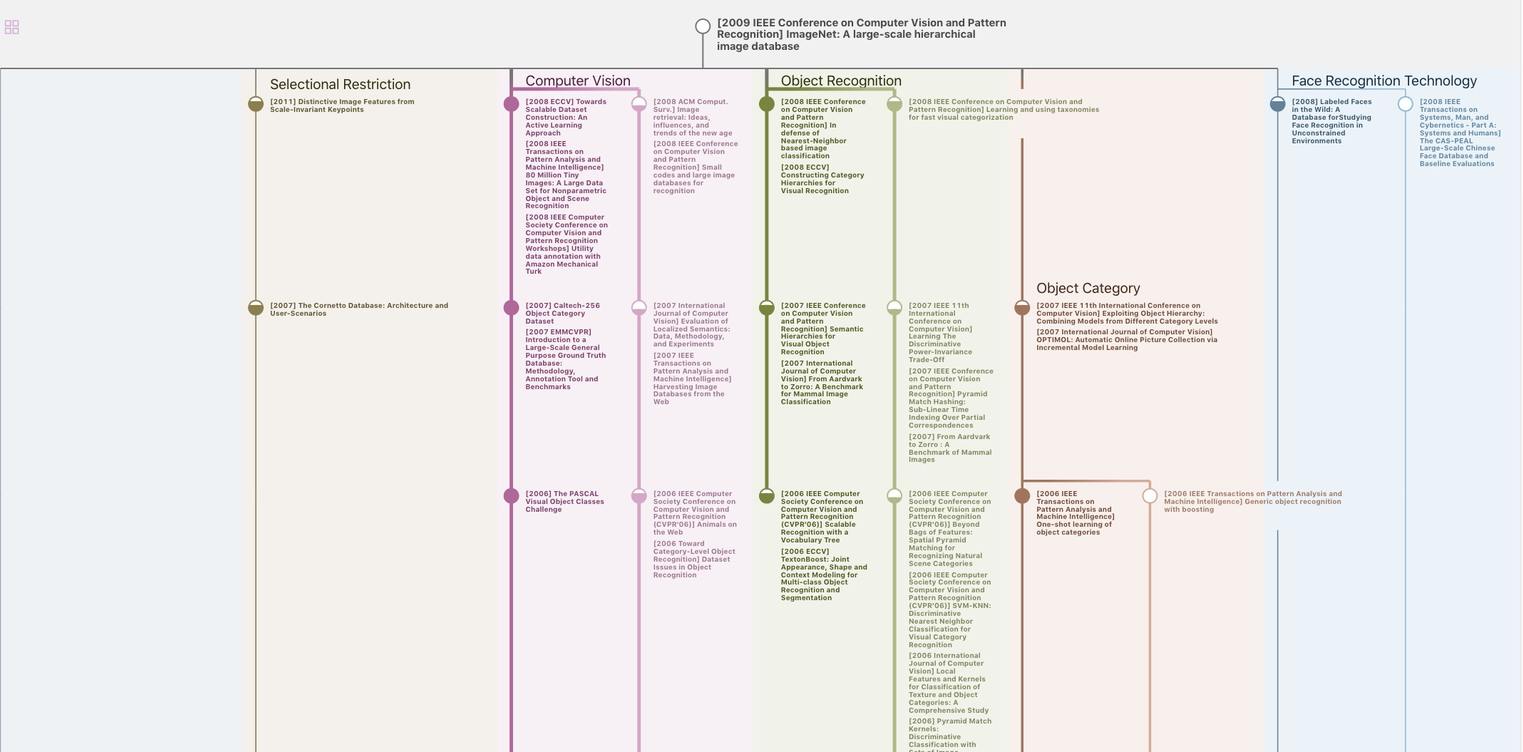
生成溯源树,研究论文发展脉络
Chat Paper
正在生成论文摘要