Classification Of Colposcopic Images Using A Multi-Breakpoints Discretization Approach On Temporal Patterns
BIOMEDICAL SIGNAL PROCESSING AND CONTROL(2021)
摘要
Cervical cancer represents the fourth cause of death in women worldwide. One of the efforts to decrease this mortality has focused on implementing automatic tools for supporting the experts in diagnosing this illness. In this work, eMODiTS was implemented to explore its performance in this particular domain. A comparison among the most used symbolic discretization (EP, SAX, alpha SAX, ESAX, ESAXKMeans, 1D-SAX, rSAX, and TD4C) methods and the well-known machine learning algorithms (kNN with k =1,5,7, SVM, J48, and MLP) was done to analyze the eMODiTS performance. Results suggest that eMODiST outperforms most of the symbolic discretization methods concerning the statistical test results; only the results of the 1D-SAX algorithm do not present a sig-nificant statistical difference compared with eMODiTS. Although our proposal results are comparable with 1D-SAX, eMODiTS achieves these results with a more compressed data version. Concerning the comparison of machine learning-based methods, MLP outperforms eMODiTS. However, the classification performance and the ability to detect truly healthy and non-diseased cases are not sacrificed by eMODiTS. Moreover, the colposcopist validated the classification results confirming that the algorithm matched the real valorization. Finally, eMODiTS provides a visual tool for making decisions by experts about cervical cancer detection in the early stages.
更多查看译文
关键词
Multi-breakpoints approach, Time series discretization, Cervical cancer, Dimensional reduction, Acetowhite response function
AI 理解论文
溯源树
样例
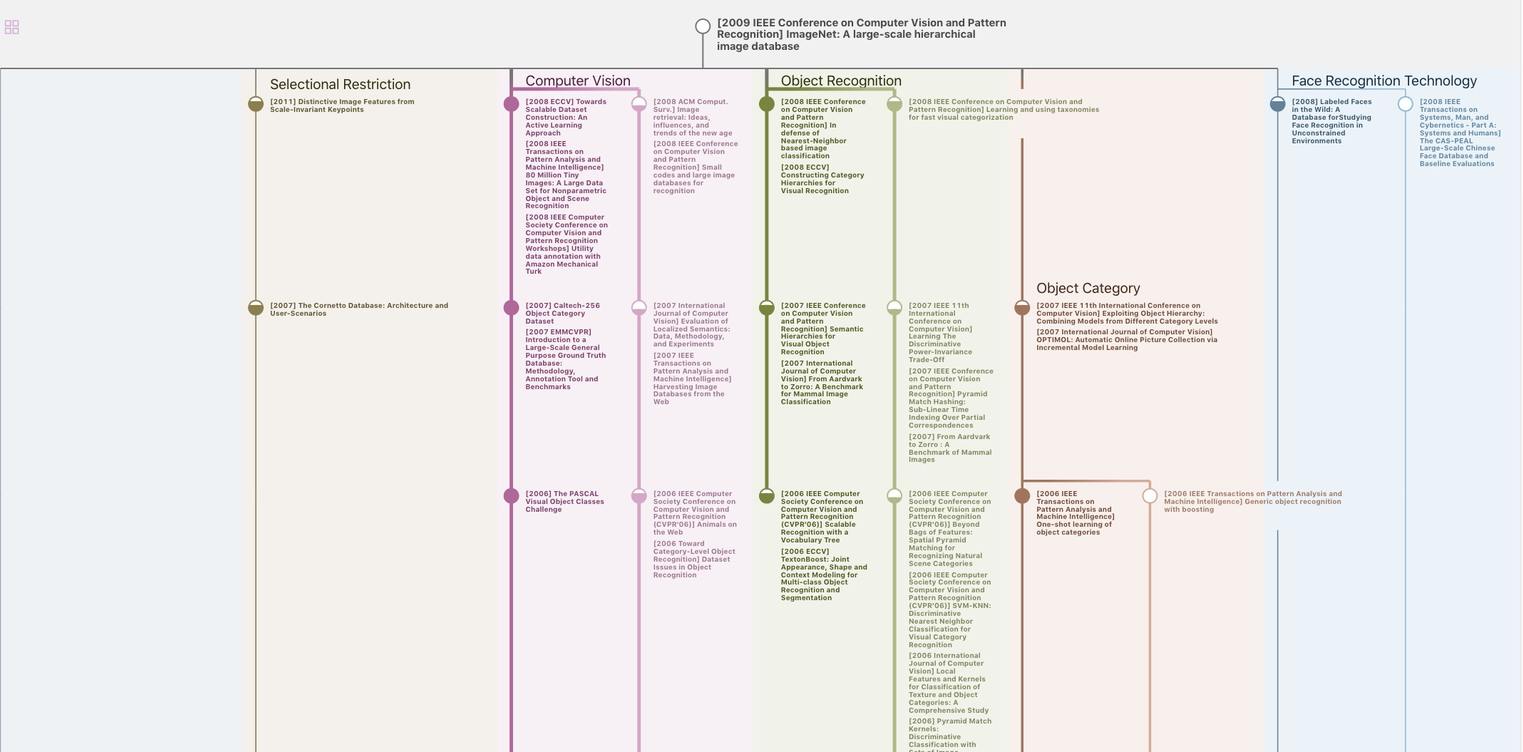
生成溯源树,研究论文发展脉络
Chat Paper
正在生成论文摘要