Eeg-Based Motor Imagery Classification Using Digraph Fourier Transforms And Extreme Learning Machines
BIOMEDICAL SIGNAL PROCESSING AND CONTROL(2021)
摘要
Motor imagery patterns are extensively exploited in brain-computer interface systems in order to control outer devices without using peripheral nerves or muscles. Classification of these patterns can be based on the associated electroencephalogram (EEG) signals. Recent approaches addressed this classification problem through techniques exploiting mainly information from one or two EEG channels. However, these approaches overlook correlations between multiple EEG channels. In this paper, we create motor-imagery classification systems based on graph-theoretic models of multichannel EEG signals. In particular, multivariate autoregressive models are used to establish the relations between the EEG channels and construct directed graph signals. Also, we constructed undirected graph signal models with Gaussian-weighted distances between graph nodes. Then, a novel variant of the graph Fourier transform is applied to the directed and undirected graph models with and without edge weights. Distinctive features were thus extracted from the transform coefficients. Additional features were computed using common spatial patterns, polynomial representations and principal components of EEG signals. Significant performance improvements were achieved using extreme learning machine (ELM) classifiers. For Dataset Ia of the BCI Competition 2003, our approach led to a classification accuracy of 96.58% with fullyconnected weighted directed graph features computed on the delta-band EEG signals. For the six subjects of the Dataset 1 of the BCI Competition IV, our approach compared well with other state-of-the-art methods in the alpha and beta EEG bands.
更多查看译文
关键词
EEG, Motor imagery, Classification, Multivariate autoregressive models, Graph signals, Graph Fourier transform
AI 理解论文
溯源树
样例
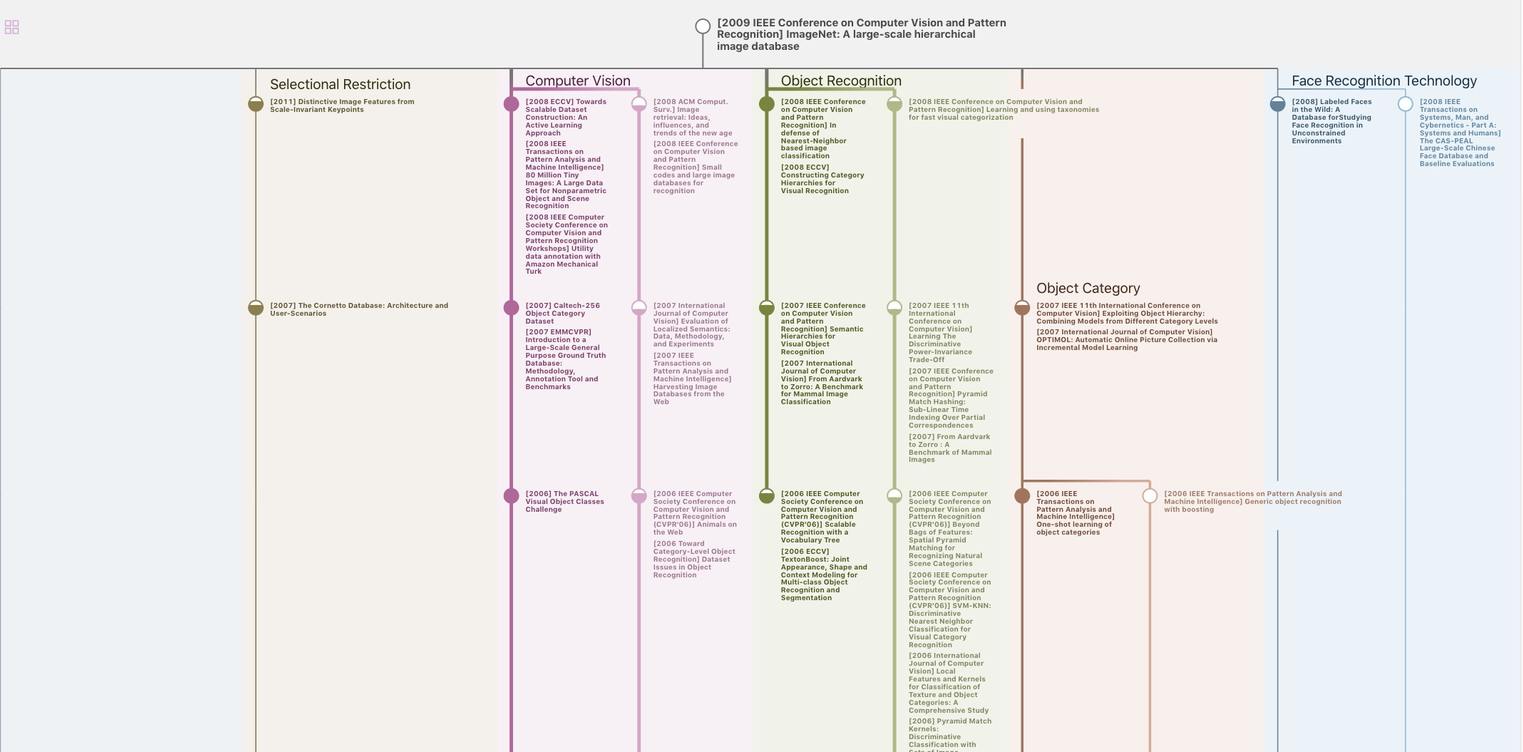
生成溯源树,研究论文发展脉络
Chat Paper
正在生成论文摘要