Sample-Label View Transfer Active Learning for Time Series Classification
ARTIFICIAL NEURAL NETWORKS AND MACHINE LEARNING, ICANN 2021, PT V(2021)
摘要
In many real-world applications, Time Series Data are captured over the course of time and exhibit temporal dependencies that cause two or otherwise identical points of time to belong to different classes or exhibit different characteristic. Although time series classification has attracted increasing attention in recent years, it remains a challenging task considering the nature of data dimensionality, voluminousness and continuous updates. Most of existing Deep Learning methods often depend on hand-crafted feature extraction techniques, that are expensive for real-world time series data mining applications which in addition, require expert knowledge. In practice, training a quality classifier is highly dependent on large number of labeled samples which is mostly inadequate in real-world time series datasets. In this paper, we present a novel Deep Learning approach for time series classification problems, called Transfer Active Learning (TAL) which jointly evaluates informativeness and representativeness of a candidate sample-label pair. TAL learns to map each input into a latent space from both sample and sample-label views which is more effective. For similar tasks, TAL is able to reuse model skill with further reduction on feature extraction costs. Extensive experiments on both classification datasets and real-world prediction tasks demonstrate the efficiency of the proposed approach on exponential reduction of training cost.
更多查看译文
关键词
Transfer learning, Active learning, Time series classification
AI 理解论文
溯源树
样例
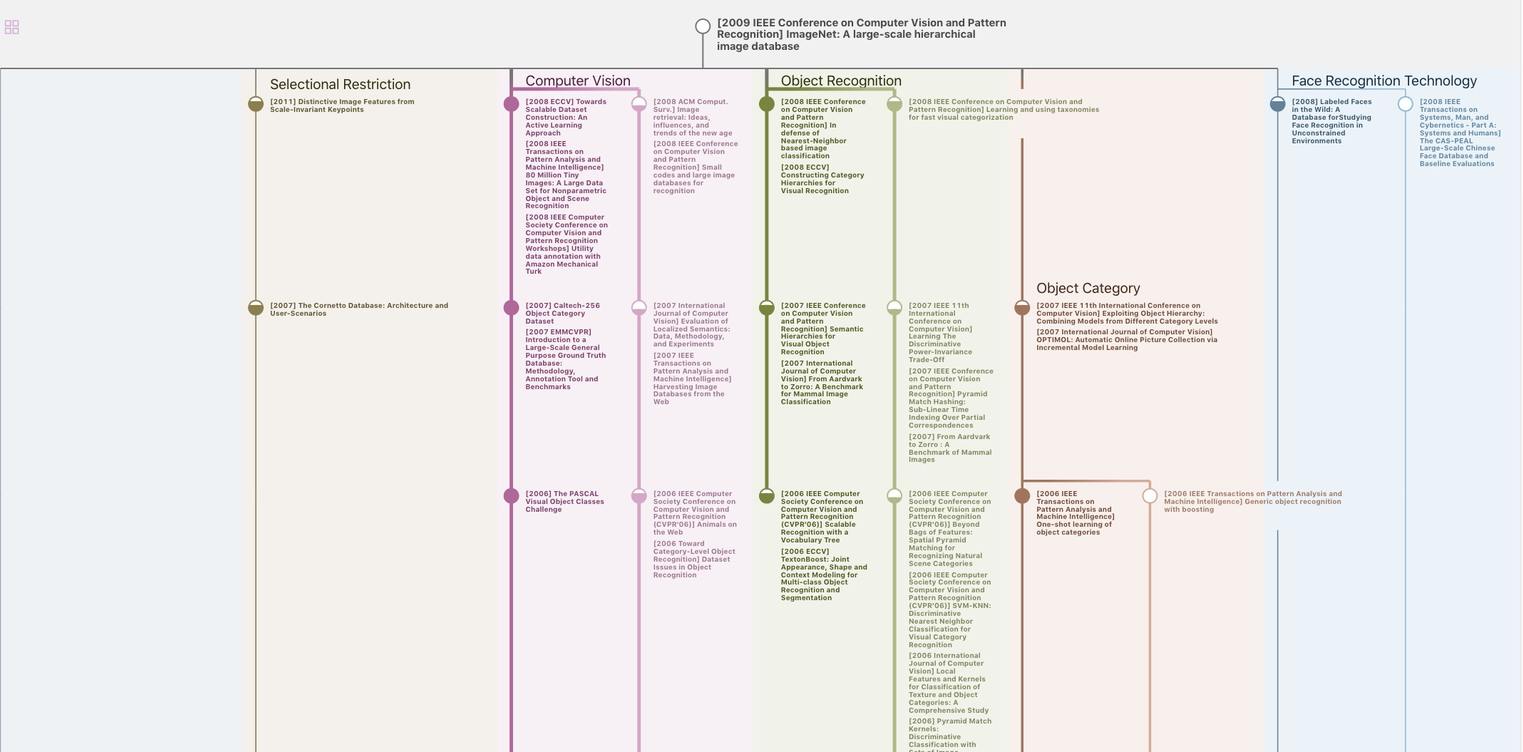
生成溯源树,研究论文发展脉络
Chat Paper
正在生成论文摘要