Empirical Study of Data-Free Iterative Knowledge Distillation
ARTIFICIAL NEURAL NETWORKS AND MACHINE LEARNING - ICANN 2021, PT III(2021)
摘要
Iterative Knowledge Distillation (IKD) [20] is an iterative variant of Hinton's knowledge distillation framework for deep neural network compression. IKD has shown promising model compression results for image classification tasks where a large amount of training data is available for training the teacher and student models. In this paper, we consider problems where training data is not available, making it impractical to use the usual IKD approach. We propose a variant of the IKD framework, called Data-Free IKD (or DF-IKD), that adopts recent results from data-free learning of deep models [2]. This exploits generative adversarial networks (GANs), in which a readily available pre-trained teacher model is regarded as a fixed discriminator, and a generator (a deep network) is used to generate training samples. The goal of the generator is to generate samples that can obtain a maximum predictive response from the discriminator. In DF-IKD, the student model at every IKD iteration is a compressed version of the original discriminator ('teacher'). Our experiments suggest: (a) DF-IKD results in a student model that is significantly smaller in size than the original parent model; (b) the predictive performance of the compressed student model is comparable to that of the parent model.
更多查看译文
关键词
Model compression, Data-free learning, Efficient ML
AI 理解论文
溯源树
样例
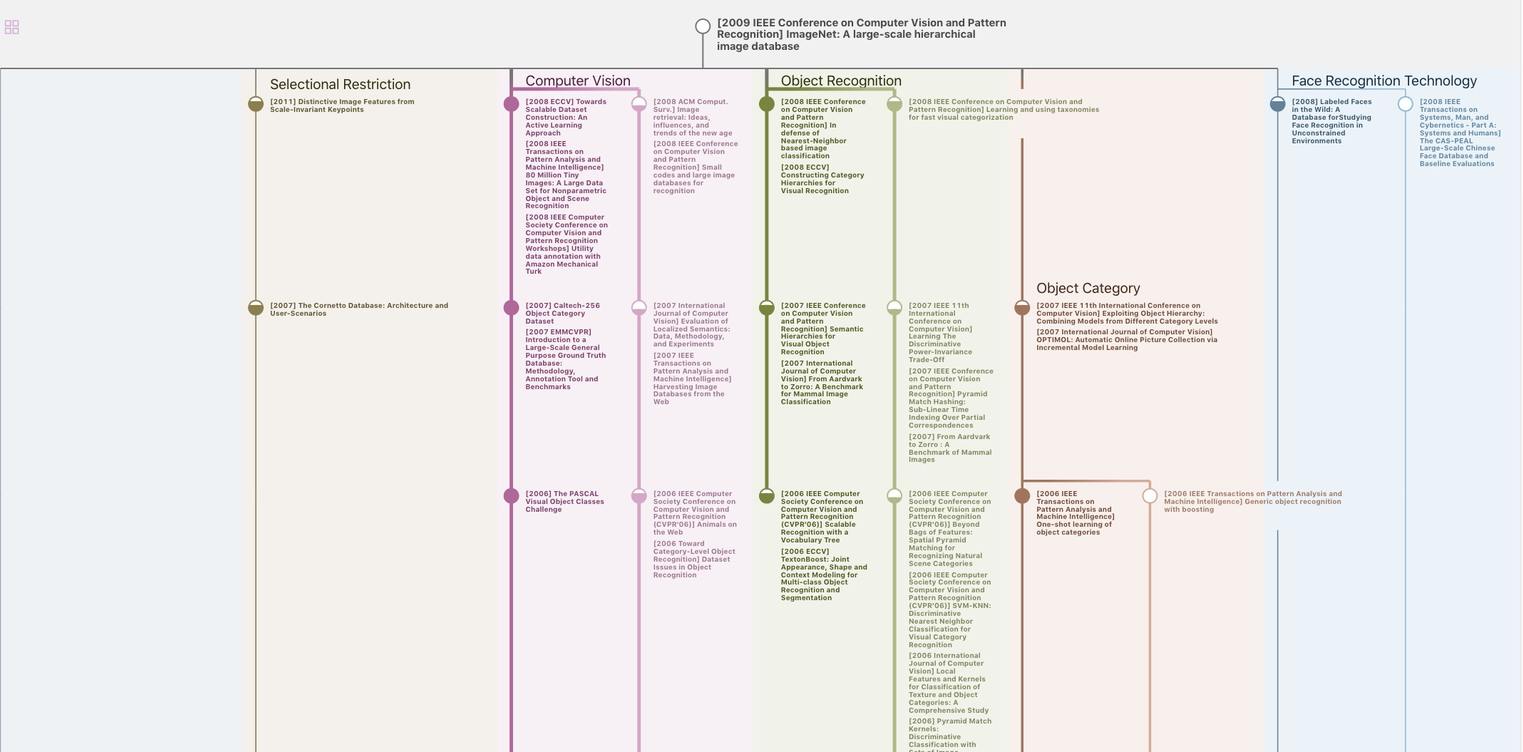
生成溯源树,研究论文发展脉络
Chat Paper
正在生成论文摘要