Learning to Navigate Intersections with Unsupervised Driver Trait Inference.
IEEE International Conference on Robotics and Automation(2022)
摘要
Navigation through uncontrolled intersections is one of the key challenges for autonomous vehicles. Identifying the subtle differences in hidden traits of other drivers can bring significant benefits when navigating in such environments. We propose an unsupervised method for inferring driver traits such as driving styles from observed vehicle trajectories. We use a variational autoencoder with recurrent neural networks to learn a latent representation of traits without any ground truth trait labels. Then, we use this trait representation to learn a policy for an autonomous vehicle to navigate through a T-intersection with deep reinforcement learning. Our pipeline enables the autonomous vehicle to adjust its actions when dealing with drivers of different traits to ensure safety and efficiency. Our method demonstrates promising performance and outperforms state-of-the-art baselines in the T-intersection scenario.
更多查看译文
关键词
navigate intersections,unsupervised driver trait inference,navigation,uncontrolled intersections,autonomous vehicle,subtle differences,hidden traits,unsupervised method,driver traits,observed vehicle trajectories,variational autoencoder,recurrent neural networks,ground truth trait labels,trait representation,deep reinforcement learning,different traits,T-intersection scenario
AI 理解论文
溯源树
样例
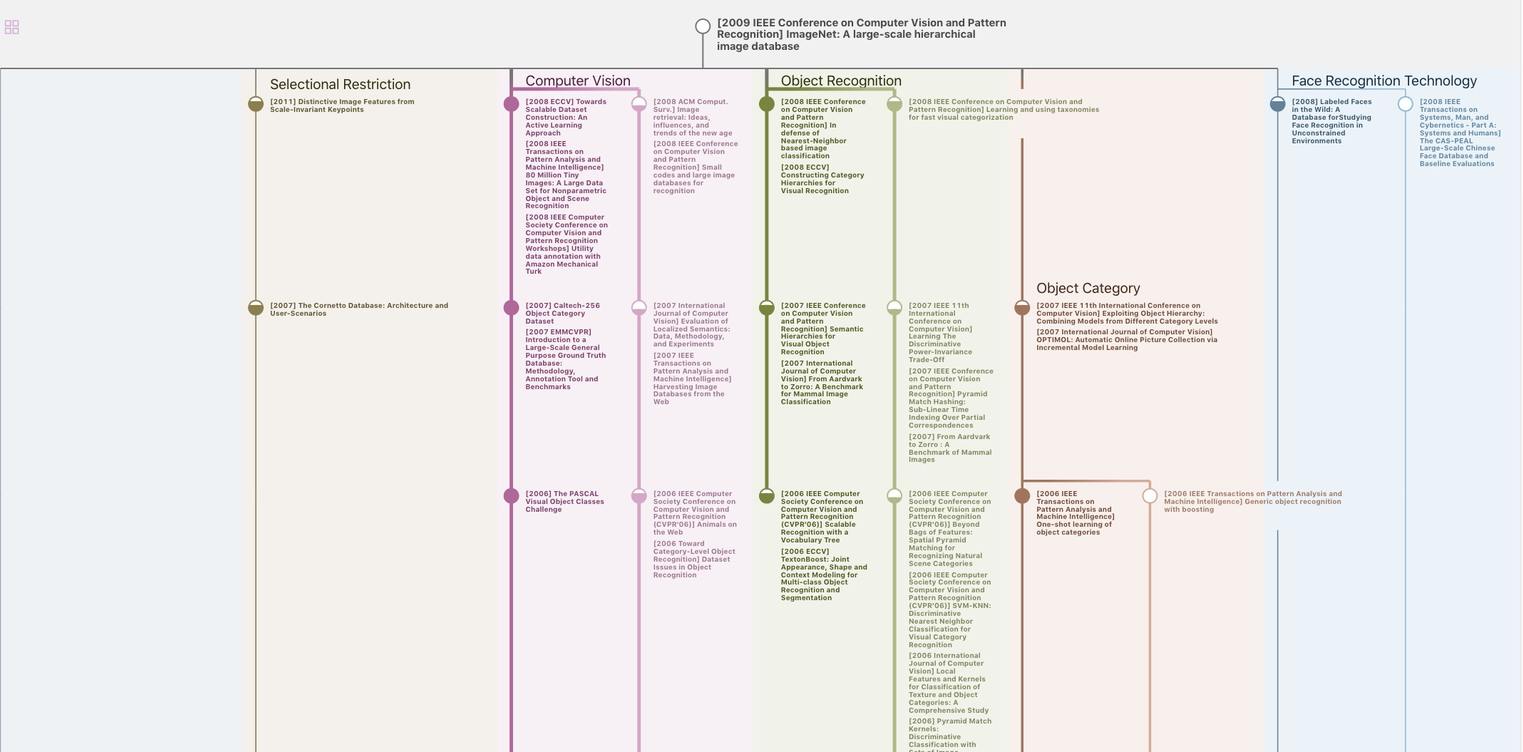
生成溯源树,研究论文发展脉络
Chat Paper
正在生成论文摘要