Effective Tensor Completion via Element-Wise Weighted Low-Rank Tensor Train With Overlapping Ket Augmentation
IEEE Transactions on Circuits and Systems for Video Technology(2022)
摘要
Tensor completion methods based on the tensor train (TT) have the issues of inaccurate weight assignment and ineffective tensor augmentation pre-processing. In this work, we propose a novel tensor completion approach via the element-wise weighted technique. Accordingly, a novel formulation for tensor completion and an effective optimization algorithm, called tensor completion by parallel weighted matrix factorization via tensor train (TWMac-TT), is proposed. In addition, we specifically consider the recovery quality of edge elements from adjacent blocks. Different from traditional reshaping and ket augmentation, we utilize a new tensor augmentation technique called overlapping ket augmentation, which can further avoid blocking artifacts. We then conduct extensive performance evaluations on synthetic data and several real image data sets. Our experimental results demonstrate that the proposed algorithm TWMac-TT outperforms several other competing tensor completion methods. The code is available at
https://github.com/yzcv/
TWMac-TT-OKA
更多查看译文
关键词
Tensor completion,tensor train,overlapping,element-wise weighted,ket augmentation
AI 理解论文
溯源树
样例
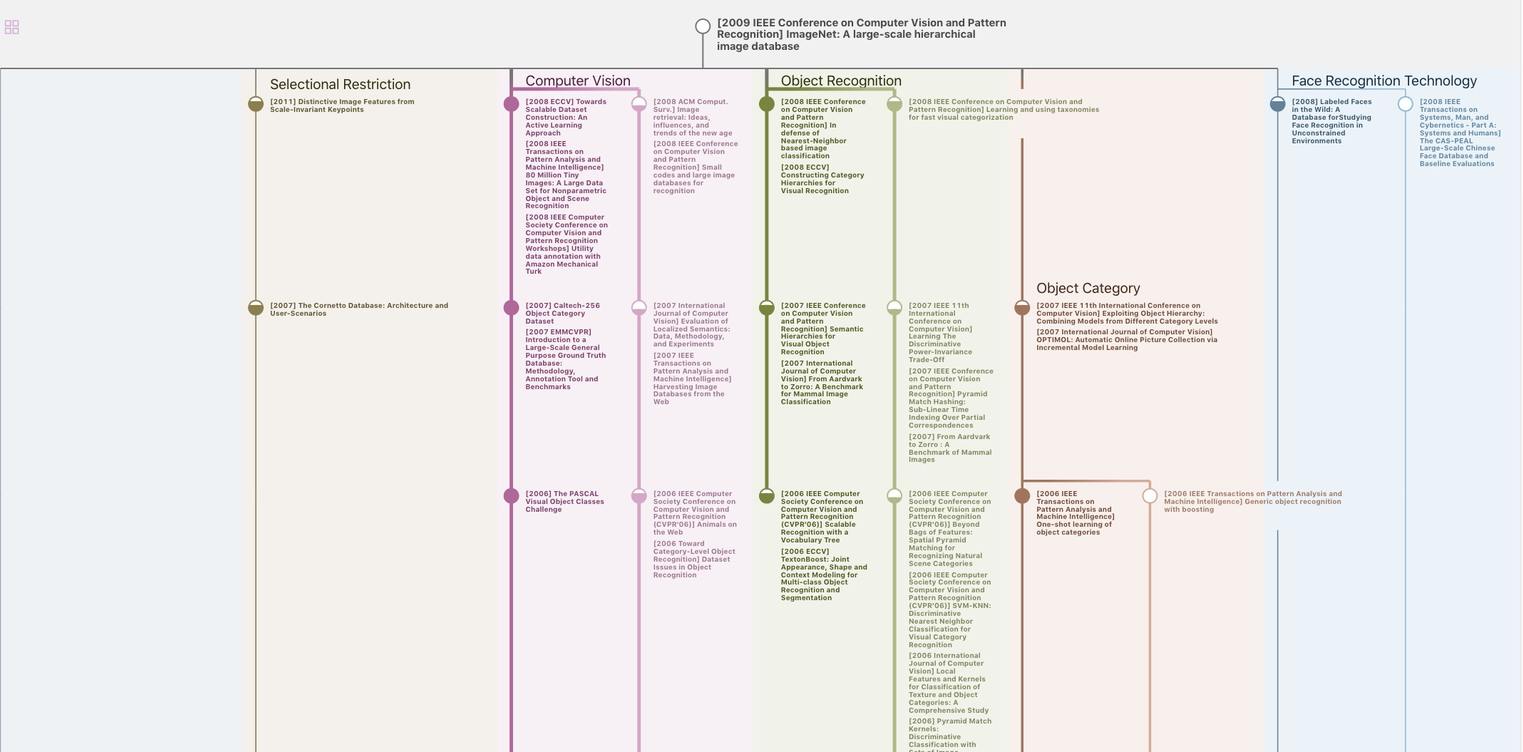
生成溯源树,研究论文发展脉络
Chat Paper
正在生成论文摘要