Structure-Attribute-Based Social Network Deanonymization With Spectral Graph Partitioning
IEEE Transactions on Computational Social Systems(2022)
摘要
Online social networks have gained tremendous popularity and have dramatically changed the way we communicate in recent years. However, the publishing of social network data raises more and more privacy concerns. To protect user privacy, social networking data are usually anonymized before being released. Nevertheless, existing anonymization techniques do not have sufficient protection effects. A large number of deanonymization attacks have arisen, and they mainly make use of either network topology or node attribute information to successfully reidentify anonymized users. In this article, we model a social network as a structure-attribute network (SAN) integrating the structural characteristics and the attribute information associated with social network users. A novel similarity measurement of social network nodes is proposed by considering the structural similarity and attribute similarity. A two-phase scheme is then designed to perform deanonymization by first dividing a social network (graph) into smaller subgraphs based on spectral graph partitioning and then applying the proposed deanonymization algorithm on each matched subgraph pair. We simulate the deanonymization attack with extensive experiments on three real-world datasets, and the experimental results demonstrate that our approach can improve the accuracy and time complexity of deanonymization compared with the state of the art.
更多查看译文
关键词
Deanonymization,differential privacy,k-anonymity,social network
AI 理解论文
溯源树
样例
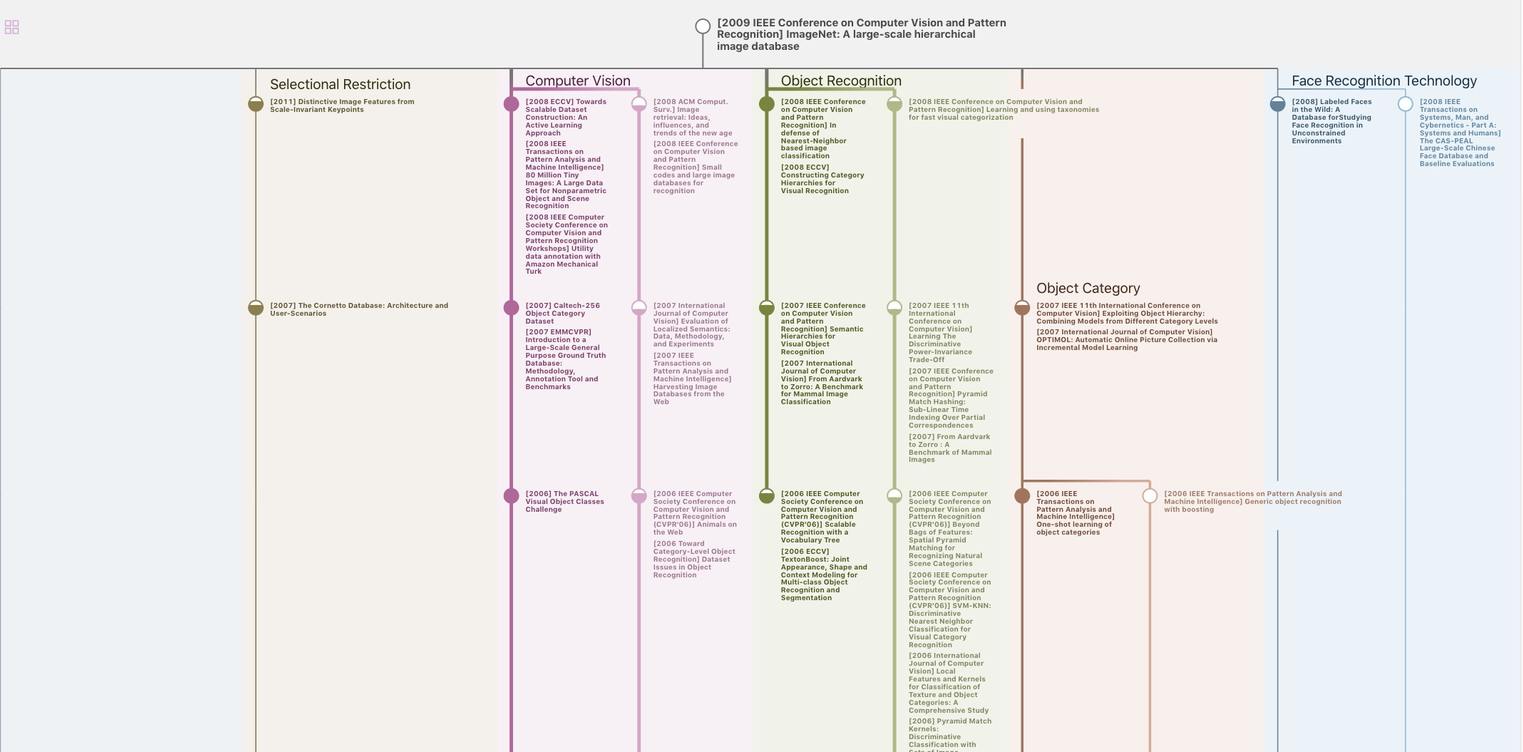
生成溯源树,研究论文发展脉络
Chat Paper
正在生成论文摘要