Massive Ultrasonic Data Compression Using Wavelet Packet Transformation Optimized by Convolutional Autoencoders
IEEE Transactions on Neural Networks and Learning Systems(2023)
摘要
Ultrasonic signal acquisition platforms generate considerable amounts of data to be stored and processed, especially when multichannel scanning or beamforming is employed. Reducing the mass storage and allowing high-speed data transmissions necessitate the compression of ultrasonic data into a representation with fewer bits. High compression accuracy is crucial in many applications, such as ultrasonic medical imaging and nondestructive testing (NDT). In this study, we present learning models for massive ultrasonic data compression on the order of megabytes. A common and highly efficient compression method for ultrasonic data is signal decomposition and subband elimination using wavelet packet transformation (WPT). We designed an algorithm for finding the wavelet kernel that provides maximum energy compaction and the optimal subband decomposition tree structure for a given ultrasonic signal. Furthermore, the WPT convolutional autoencoder (WPTCAE) compression algorithm is proposed based on the WPT compression tree structure and the use of machine learning for estimating the optimal kernel. To further improve the compression accuracy, an autoencoder (AE) is incorporated into the WPTCAE model to build a hybrid model. The performance of the WPTCAE compression model is examined and benchmarked against other compression algorithms using ultrasonic radio frequency (RF) datasets acquired in NDT and medical imaging applications. The experimental results clearly show that the WPTCAE compression model provides improved compression ratios while maintaining high signal fidelity. The proposed learning models can achieve a compression accuracy of 98% by using only 6% of the original data.
更多查看译文
关键词
Convolutional autoencoder (CAE),data compression,ultrasonic signals,unsupervised learning,wavelet packet transformation (WPT)
AI 理解论文
溯源树
样例
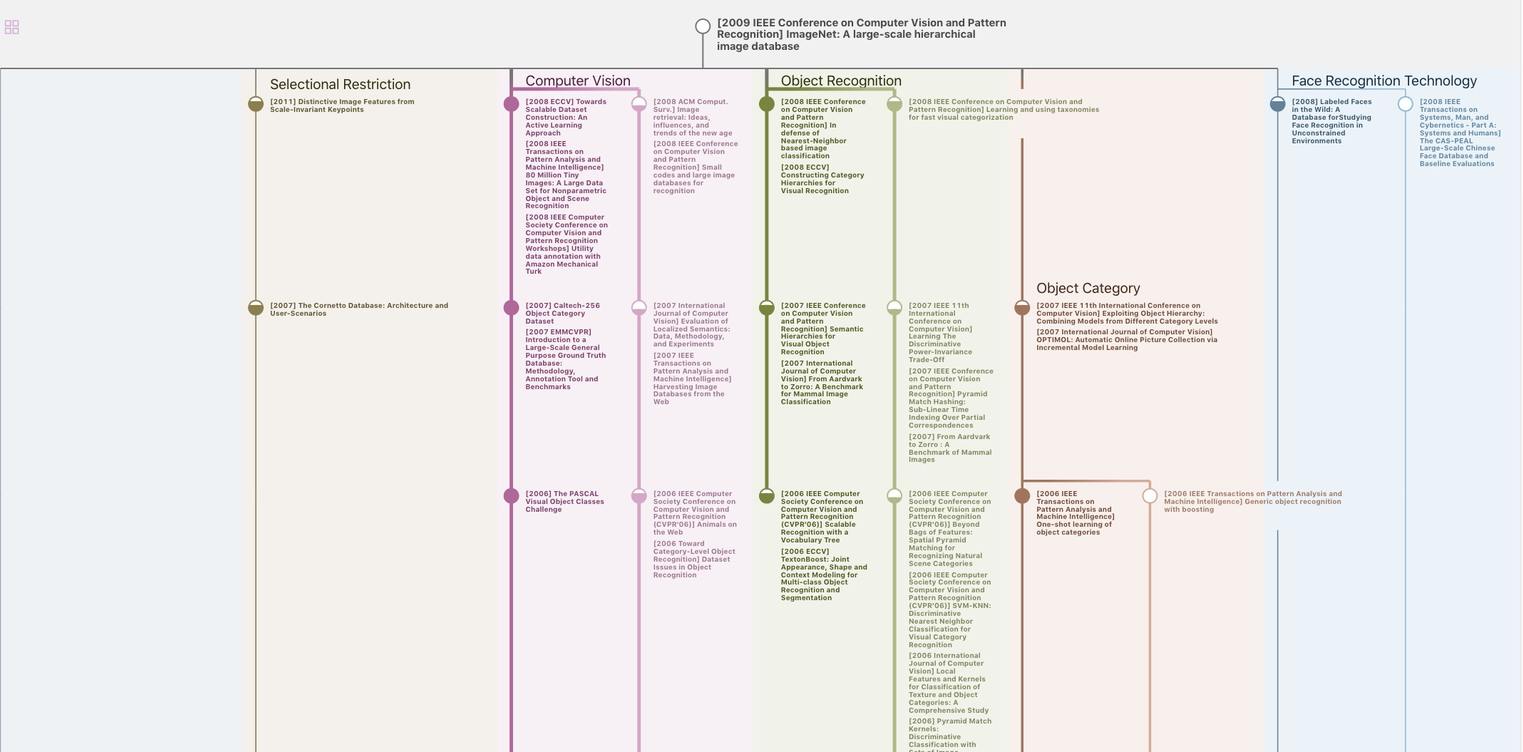
生成溯源树,研究论文发展脉络
Chat Paper
正在生成论文摘要