Analog Joint Source-Channel Coding For Distributed Functional Compression Using Deep Neural Networks
2021 IEEE INTERNATIONAL SYMPOSIUM ON INFORMATION THEORY (ISIT)(2021)
摘要
In this paper, we study Joint Source-Channel Coding (JSCC) for distributed analog functional compression over both Gaussian Multiple Access Channel (MAC) and AWGN channels. Notably, we propose a deep neural network based solution for learning encoders and decoders. We propose three methods of increasing performance. The first one frames the problem as an autoencoder; the second one incorporates the power constraint in the objective by using a Lagrange multiplier; the third method derives the objective from the information bottleneck principle. We show that all proposed methods are variational approximations to upper bounds on the indirect rate-distortion problem's minimization objective. Further, we show that the third method is the variational approximation of a tighter upper bound compared to the other two. Finally, we show empirical performance results for image classification. We compare with existing work and showcase the performance improvement yielded by the proposed methods.
更多查看译文
关键词
analog Joint Source-Channel Coding,distributed functional compression,deep neural networks,distributed analog functional compression,deep neural network based solution,encoders,decoders,information bottleneck principle,variational approximation,indirect rate-distortion problem,empirical performance results,Gaussian Multiple Access Channel,AWGN channels,Lagrange multiplier
AI 理解论文
溯源树
样例
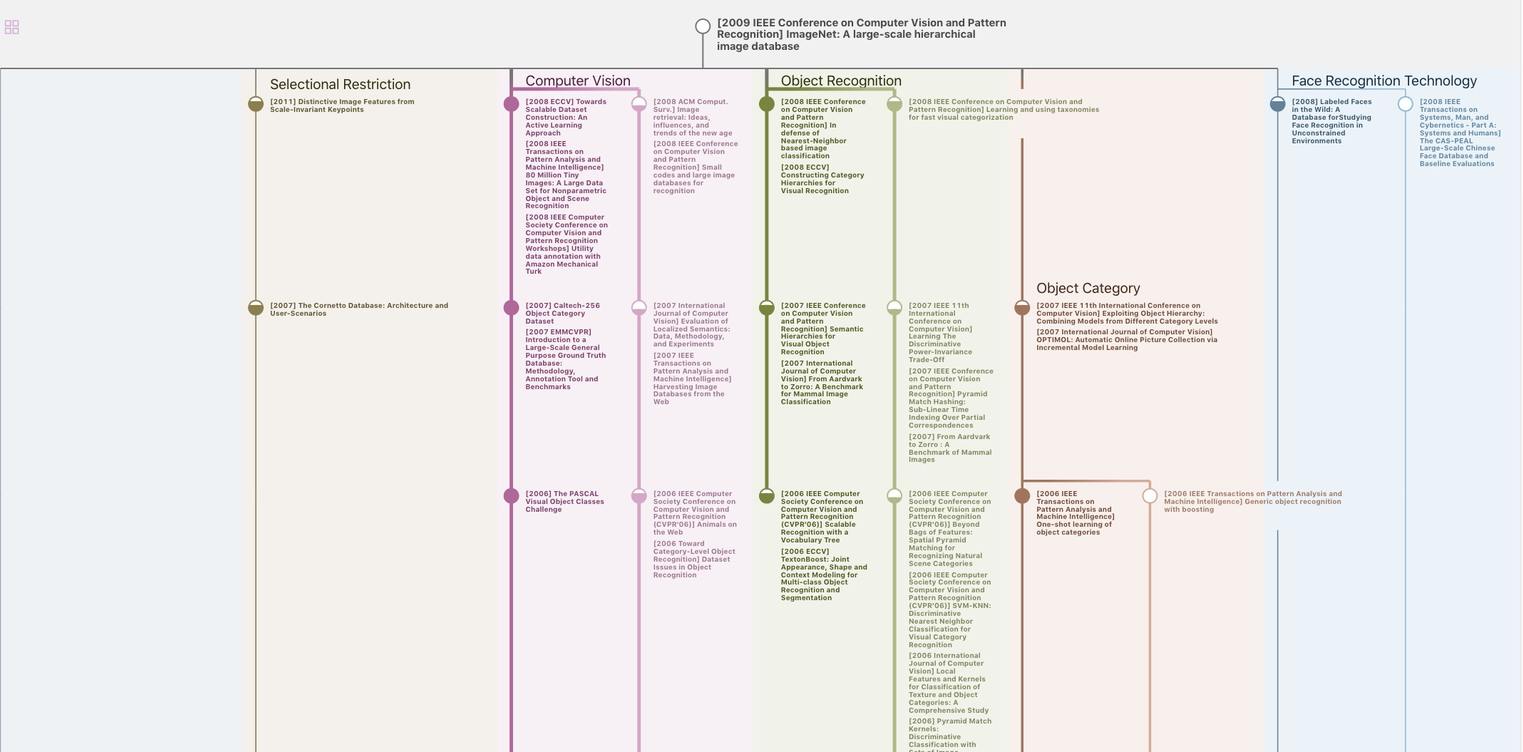
生成溯源树,研究论文发展脉络
Chat Paper
正在生成论文摘要