Boosting Search Engines with Interactive Agents
arxiv(2021)
摘要
Can machines learn to use a search engine as an interactive tool for finding information? That would have far reaching consequences for making the world's knowledge more accessible. This paper presents first steps in designing agents that learn meta-strategies for contextual query refinements. Our approach uses machine reading to guide the selection of refinement terms from aggregated search results. Agents are then empowered with simple but effective search operators to exert fine-grained and transparent control over queries and search results. We develop a novel way of generating synthetic search sessions, which leverages the power of transformer-based generative language models through (self-)supervised learning. We also present a reinforcement learning agent with dynamically constrained actions that can learn interactive search strategies completely from scratch. In both cases, we obtain significant improvements over one-shot search with a strong information retrieval baseline. Finally, we provide an in-depth analysis of the learned search policies.
更多查看译文
关键词
interactive agents,search engines
AI 理解论文
溯源树
样例
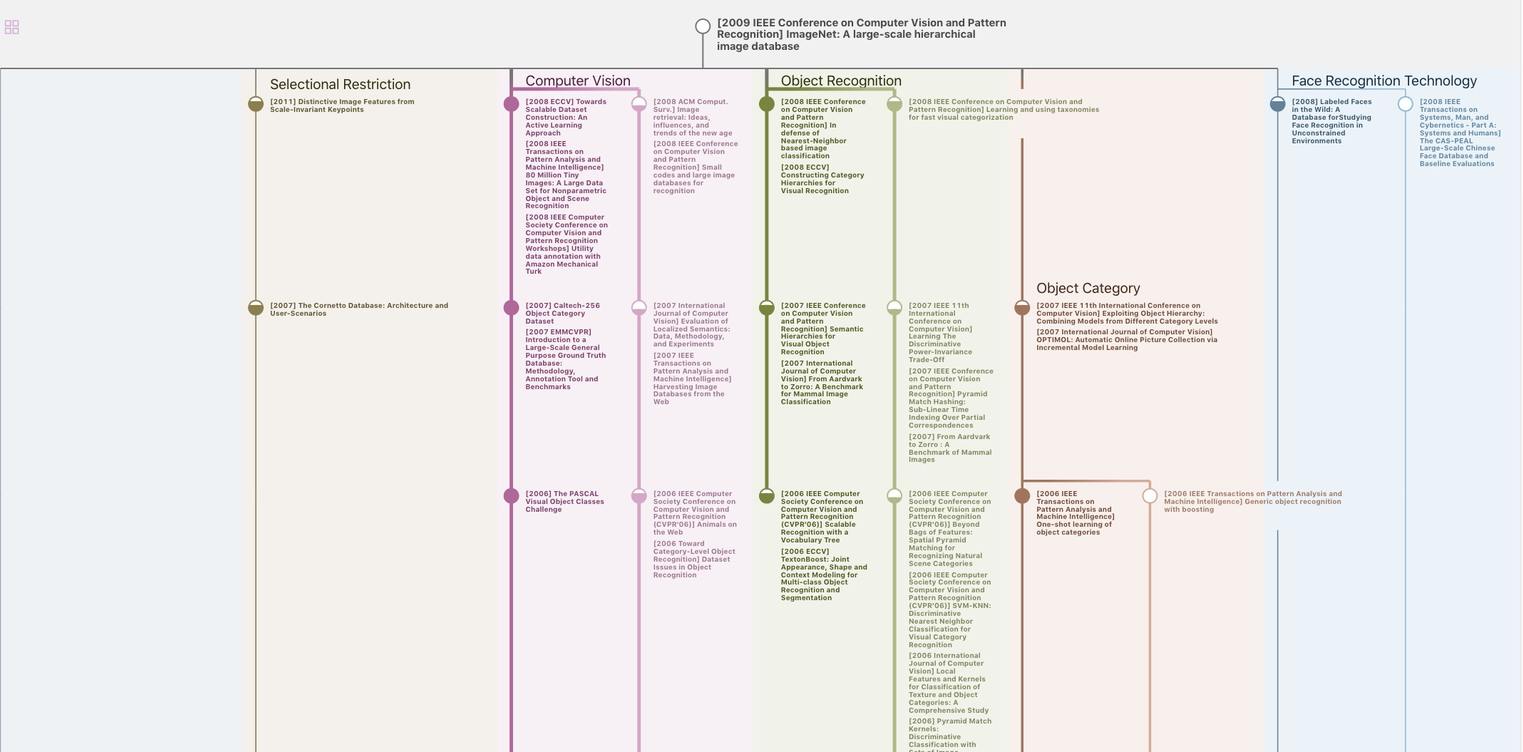
生成溯源树,研究论文发展脉络
Chat Paper
正在生成论文摘要