MTUNet: Few-shot Image Classification with Visual Explanations
2021 IEEE/CVF CONFERENCE ON COMPUTER VISION AND PATTERN RECOGITION WORKSHOPS (CVPRW 2021)(2021)
摘要
Few-shot learning (FSL) approaches, mostly neural network-based, are assuming that the pre-trained knowledge can be obtained from base (seen) categories and transferred to novel (unseen) categories. However, the black-box nature of neural networks makes it difficult to understand what is actually transferred, which may hamper its application in some risk-sensitive areas. In this paper, we reveal a new way to perform explainable FSL for image classification, using discriminative patterns and pairwise matching. Experimental results prove that the proposed method can achieve satisfactory explainability on two mainstream datasets. Code is available*.
更多查看译文
关键词
MTUNet,few-shot image classification,visual explanations,neural network,pre-trained knowledge,black-box nature,risk-sensitive areas,explainable FSL,discriminative patterns,pairwise matching,few-shot learning
AI 理解论文
溯源树
样例
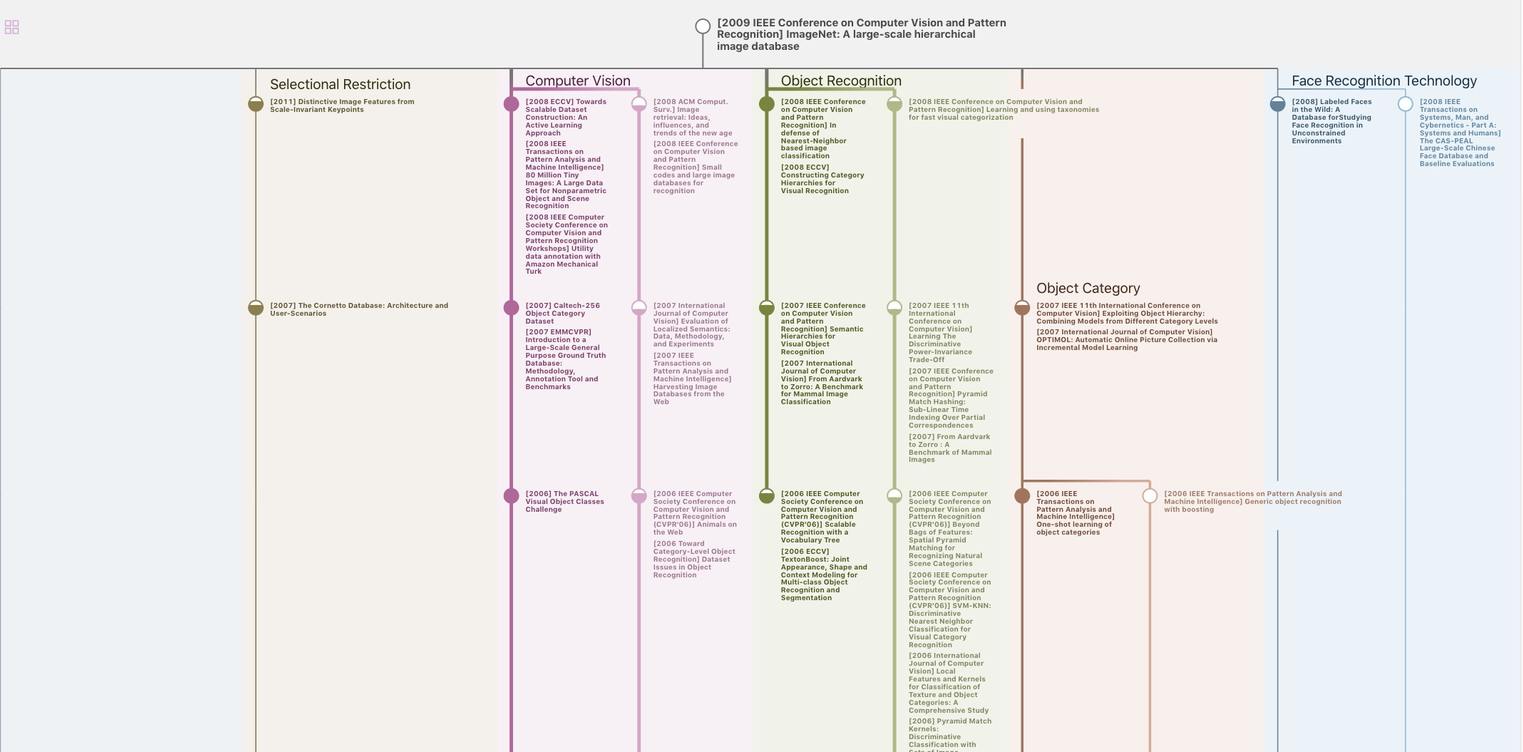
生成溯源树,研究论文发展脉络
Chat Paper
正在生成论文摘要