Modeling Fashion Compatibility with Explanation by using Bidirectional LSTM
2021 IEEE/CVF CONFERENCE ON COMPUTER VISION AND PATTERN RECOGITION WORKSHOPS (CVPRW 2021)(2021)
摘要
The goal of this paper is to model the fashion compatibility of an outfit and provide the explanations. We first extract features of all attributes of all items via convolutional neural networks, and then train the bidirectional Long Shortterm Memory (Bi-LSTM) model to learn the compatibility of an outfit by treating these attribute features as a sequence. Gradient penalty regularization is exploited for training inter-factor compatibility net which is used to compute the loss for judgment and provide its explanation which is generated from the recognized reasons related to the judgment. To train and evaluate the proposed approach, we expanded the EVALUATION3 dataset in terms of the number of items and attributes. Experiment results show that our approach can successfully evaluate compatibility with reason.
更多查看译文
关键词
bidirectional LSTM,convolutional neural networks,bidirectional long short-term memory,attribute features,gradient penalty regularization,training inter-factor compatibility net,EVALUATION3 dataset,modeling fashion compatibility,Bi-LSTM
AI 理解论文
溯源树
样例
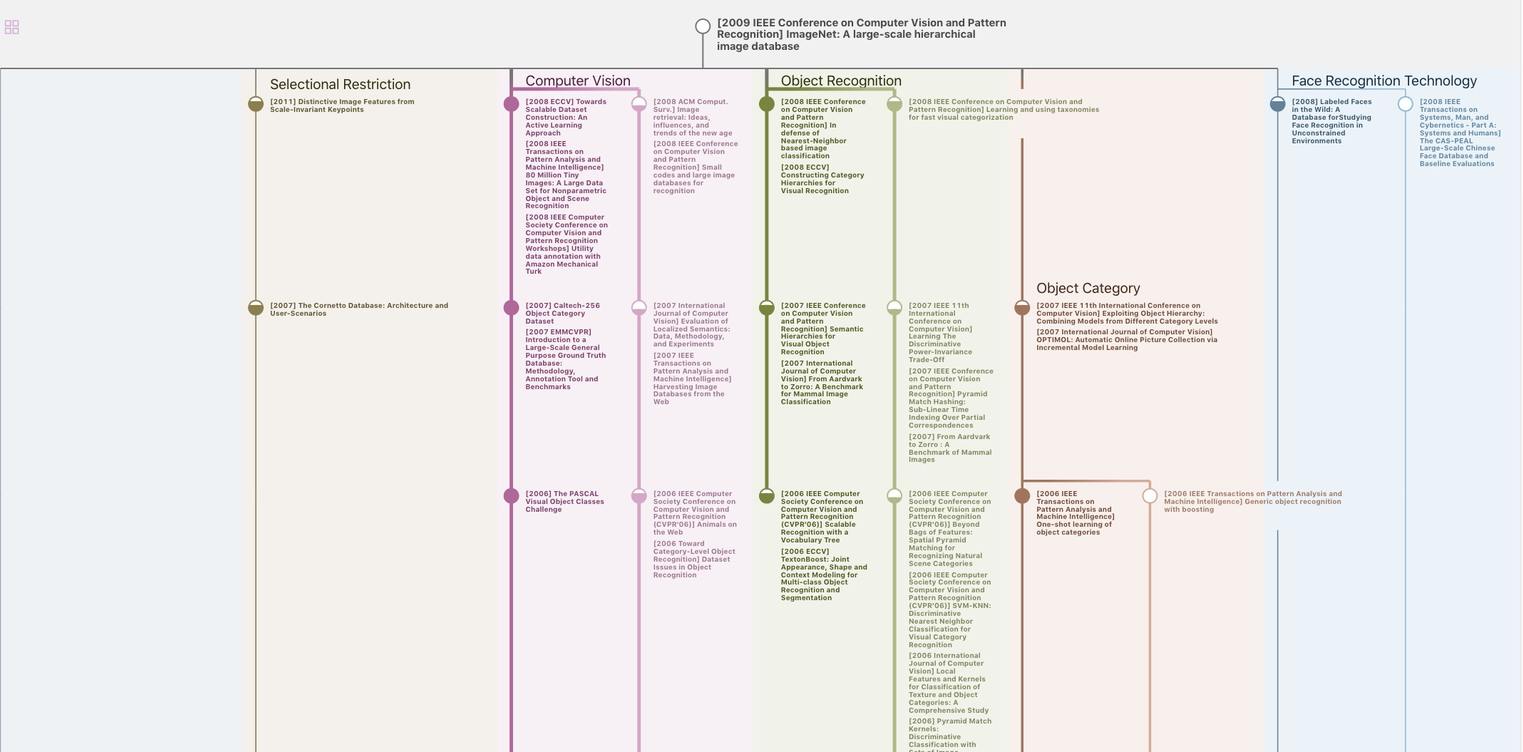
生成溯源树,研究论文发展脉络
Chat Paper
正在生成论文摘要