Self-Attention-Based Conditional Variational Auto-Encoder Generative Adversarial Networks For Hyperspectral Classification
REMOTE SENSING(2021)
摘要
Hyperspectral classification is an important technique for remote sensing image analysis. For the current classification methods, limited training data affect the classification results. Recently, Conditional Variational Autoencoder Generative Adversarial Network (CVAEGAN) has been used to generate virtual samples to augment the training data, which could improve the classification performance. To further improve the classification performance, based on the CVAEGAN, we propose a Self-Attention-Based Conditional Variational Autoencoder Generative Adversarial Network (SACVAEGAN). Compared with CVAEGAN, we first use random latent vectors to obtain more enhanced virtual samples, which can improve the generalization performance. Then, we introduce the self-attention mechanism into our model to force the training process to pay more attention to global information, which can achieve better classification accuracy. Moreover, we explore model stability by incorporating the WGAN-GP loss function into our model to reduce the mode collapse probability. Experiments on three data sets and a comparison of the state-of-art methods show that SACVAEGAN has great advantages in accuracy compared with state-of-the-art HSI classification methods.
更多查看译文
关键词
Generative Adversarial Network (GAN), hyperspectral classification, self-attention
AI 理解论文
溯源树
样例
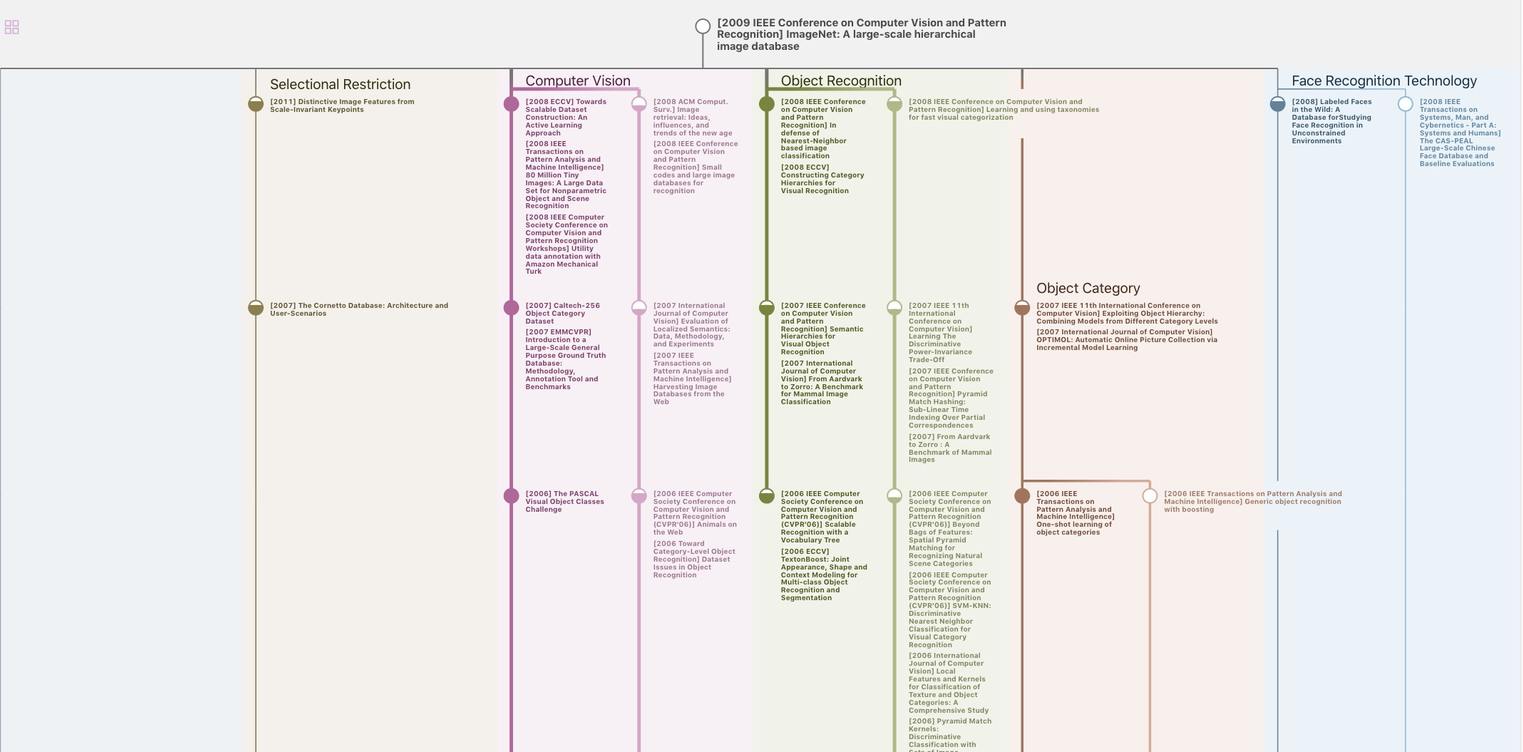
生成溯源树,研究论文发展脉络
Chat Paper
正在生成论文摘要