Compound Multiscale Weak Dense Network With Hybrid Attention For Hyperspectral Image Classification
REMOTE SENSING(2021)
摘要
Recently, hyperspectral image (HSI) classification has become a popular research direction in remote sensing. The emergence of convolutional neural networks (CNNs) has greatly promoted the development of this field and demonstrated excellent classification performance. However, due to the particularity of HSIs, redundant information and limited samples pose huge challenges for extracting strong discriminative features. In addition, addressing how to fully mine the internal correlation of the data or features based on the existing model is also crucial in improving classification performance. To overcome the above limitations, this work presents a strong feature extraction neural network with an attention mechanism. Firstly, the original HSI is weighted by means of the hybrid spectral-spatial attention mechanism. Then, the data are input into a spectral feature extraction branch and a spatial feature extraction branch, composed of multiscale feature extraction modules and weak dense feature extraction modules, to extract high-level semantic features. These two features are compressed and fused using the global average pooling and concat approaches. Finally, the classification results are obtained by using two fully connected layers and one Softmax layer. A performance comparison shows the enhanced classification performance of the proposed model compared to the current state of the art on three public datasets.
更多查看译文
关键词
hyperspectral image classification, deep learning, attention mechanism, multiscale feature extraction, feature fusion, skip connection
AI 理解论文
溯源树
样例
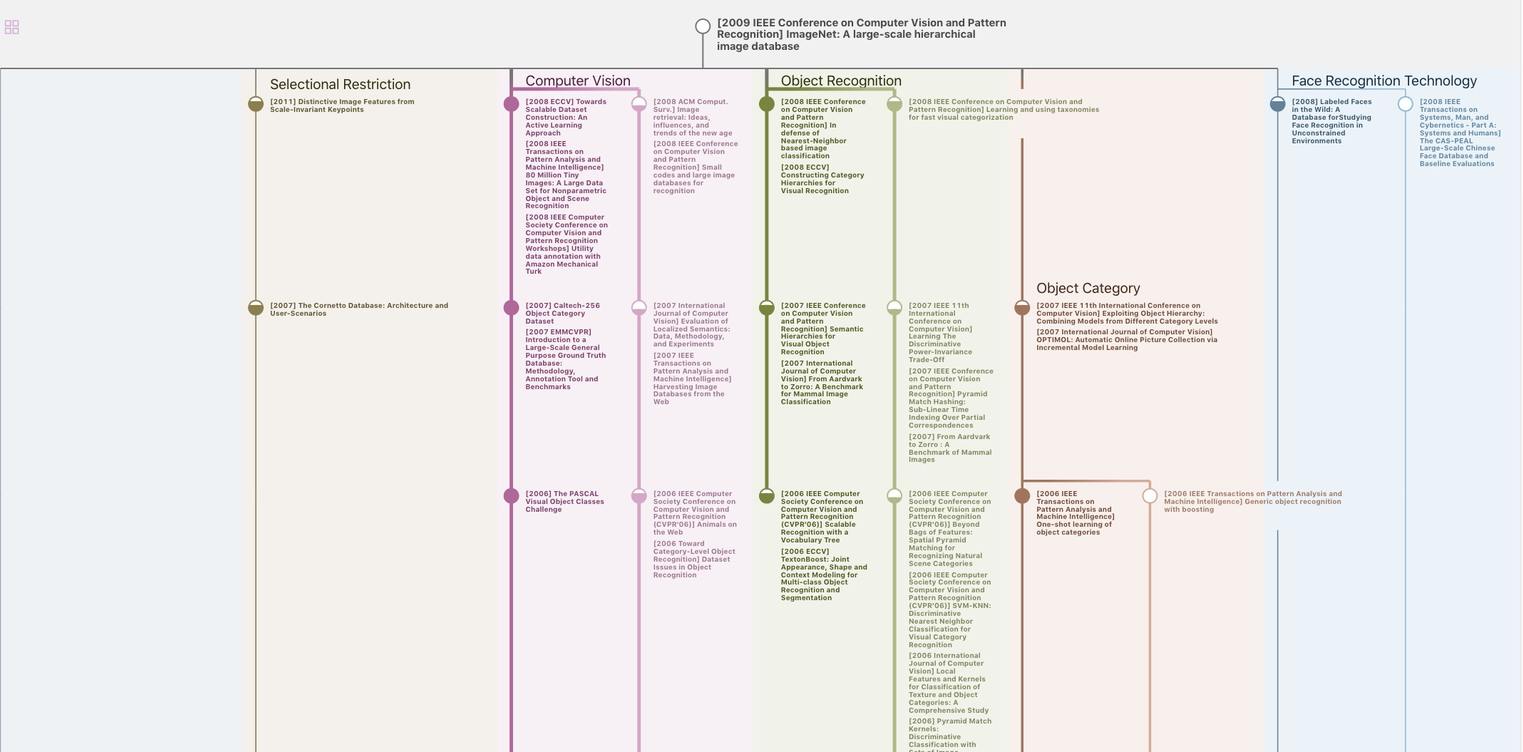
生成溯源树,研究论文发展脉络
Chat Paper
正在生成论文摘要