Mare: Self-Supervised Multi-Attention Resu-Net For Semantic Segmentation In Remote Sensing
REMOTE SENSING(2021)
摘要
Scene understanding of satellite and aerial images is a pivotal task in various remote sensing (RS) practices, such as land cover and urban development monitoring. In recent years, neural networks have become a de-facto standard in many of these applications. However, semantic segmentation still remains a challenging task. With respect to other computer vision (CV) areas, in RS large labeled datasets are not very often available, due to their large cost and to the required manpower. On the other hand, self-supervised learning (SSL) is earning more and more interest in CV, reaching state-of-the-art in several tasks. In spite of this, most SSL models, pretrained on huge datasets like ImageNet, do not perform particularly well on RS data. For this reason, we propose a combination of a SSL algorithm (particularly, Online Bag of Words) and a semantic segmentation algorithm, shaped for aerial images (namely, Multistage Attention ResU-Net), to show new encouraging results (i.e., 81.76% mIoU with ResNet-18 backbone) on the ISPRS Vaihingen dataset.
更多查看译文
关键词
semantic segmentation, self-supervised learning, linear attention, Vaihingen dataset
AI 理解论文
溯源树
样例
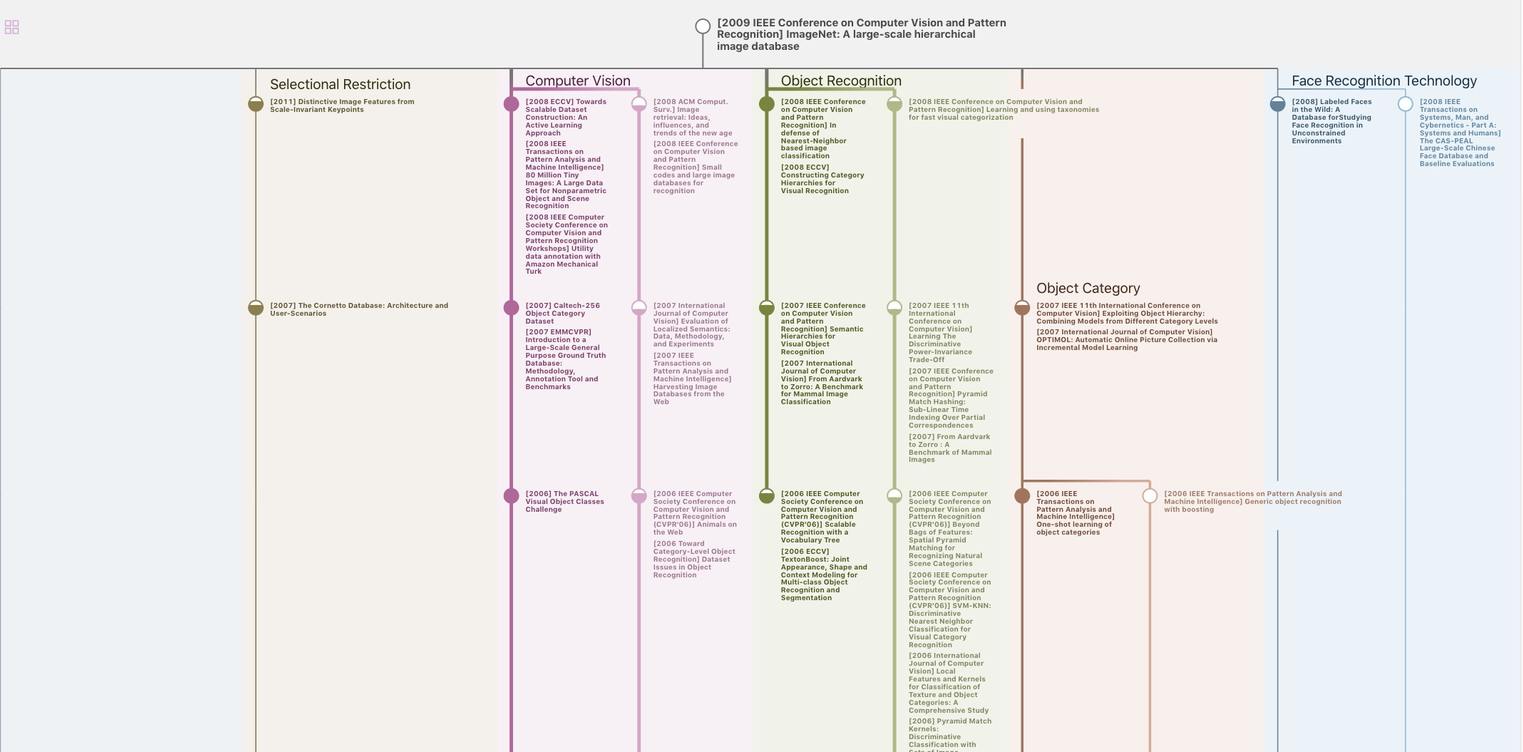
生成溯源树,研究论文发展脉络
Chat Paper
正在生成论文摘要