Latent Tree Decomposition Parsers for AMR-to-Text Generation
arxiv(2021)
摘要
Graph encoders in AMR-to-text generation models often rely on neighborhood convolutions or global vertex attention. While these approaches apply to general graphs, AMRs may be amenable to encoders that target their tree-like structure. By clustering edges into a hierarchy, a tree decomposition summarizes graph structure. Our model encodes a derivation forest of tree decompositions and extracts an expected tree. From tree node embeddings, it builds graph edge features used in vertex attention of the graph encoder. Encoding TD forests instead of shortest-pairwise paths in a self-attentive baseline raises BLEU by 0.7 and chrF++ by 0.3. The forest encoder also surpasses a convolutional baseline for molecular property prediction by 1.92% ROC-AUC.
更多查看译文
关键词
generation,decomposition,amr-to-text
AI 理解论文
溯源树
样例
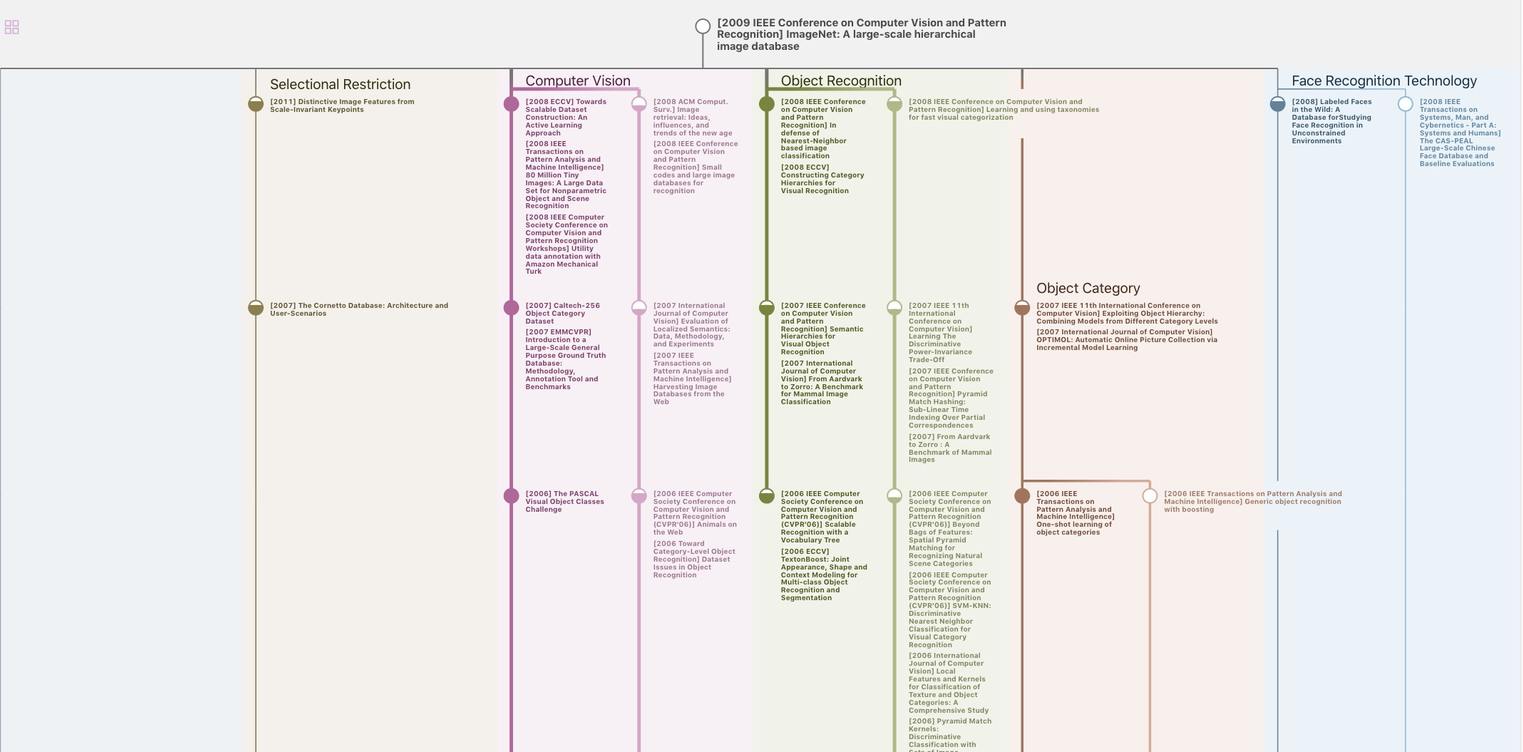
生成溯源树,研究论文发展脉络
Chat Paper
正在生成论文摘要