Explainable Artificial Intelligence for Bias Detection in COVID CT-Scan Classifiers.
Sensors (Basel, Switzerland)(2021)
摘要
PROBLEM:An application of Explainable Artificial Intelligence Methods for COVID CT-Scan classifiers is presented.
MOTIVATION:It is possible that classifiers are using spurious artifacts in dataset images to achieve high performances, and such explainable techniques can help identify this issue.
AIM:For this purpose, several approaches were used in tandem, in order to create a complete overview of the classificatios.
METHODOLOGY:The techniques used included GradCAM, LIME, RISE, Squaregrid, and direct Gradient approaches (Vanilla, Smooth, Integrated).
MAIN RESULTS:Among the deep neural networks architectures evaluated for this image classification task, VGG16 was shown to be most affected by biases towards spurious artifacts, while DenseNet was notably more robust against them. Further impacts: Results further show that small differences in validation accuracies can cause drastic changes in explanation heatmaps for DenseNet architectures, indicating that small changes in validation accuracy may have large impacts on the biases learned by the networks. Notably, it is important to notice that the strong performance metrics achieved by all these networks (Accuracy, F1 score, AUC all in the 80 to 90% range) could give users the erroneous impression that there is no bias. However, the analysis of the explanation heatmaps highlights the bias.
更多查看译文
AI 理解论文
溯源树
样例
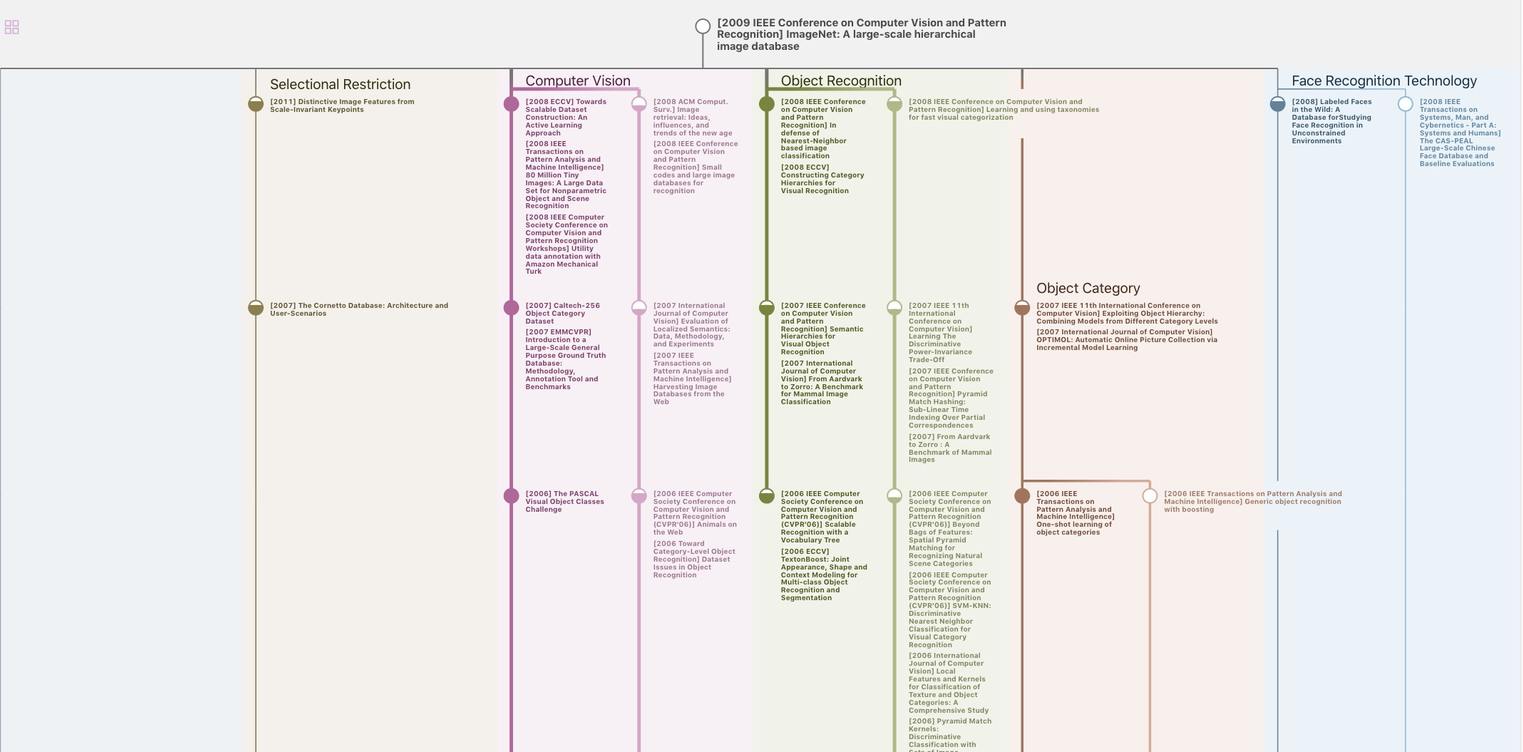
生成溯源树,研究论文发展脉络
Chat Paper
正在生成论文摘要