Estimation of Riemannian distances between covariance operators and Gaussian processes
arxiv(2021)
摘要
In this work we study two Riemannian distances between infinite-dimensional positive definite Hilbert-Schmidt operators, namely affine-invariant Riemannian and Log-Hilbert-Schmidt distances, in the context of covariance operators associated with functional stochastic processes, in particular Gaussian processes. Our first main results show that both distances converge in the Hilbert-Schmidt norm. Using concentration results for Hilbert space-valued random variables, we then show that both distances can be consistently and efficiently estimated from (i) sample covariance operators, (ii) finite, normalized covariance matrices, and (iii) finite samples generated by the given processes, all with dimension-independent convergence. Our theoretical analysis exploits extensively the methodology of reproducing kernel Hilbert space (RKHS) covariance and cross-covariance operators. The theoretical formulation is illustrated with numerical experiments on covariance operators of Gaussian processes.
更多查看译文
关键词
covariance operators,riemannian distances,estimation
AI 理解论文
溯源树
样例
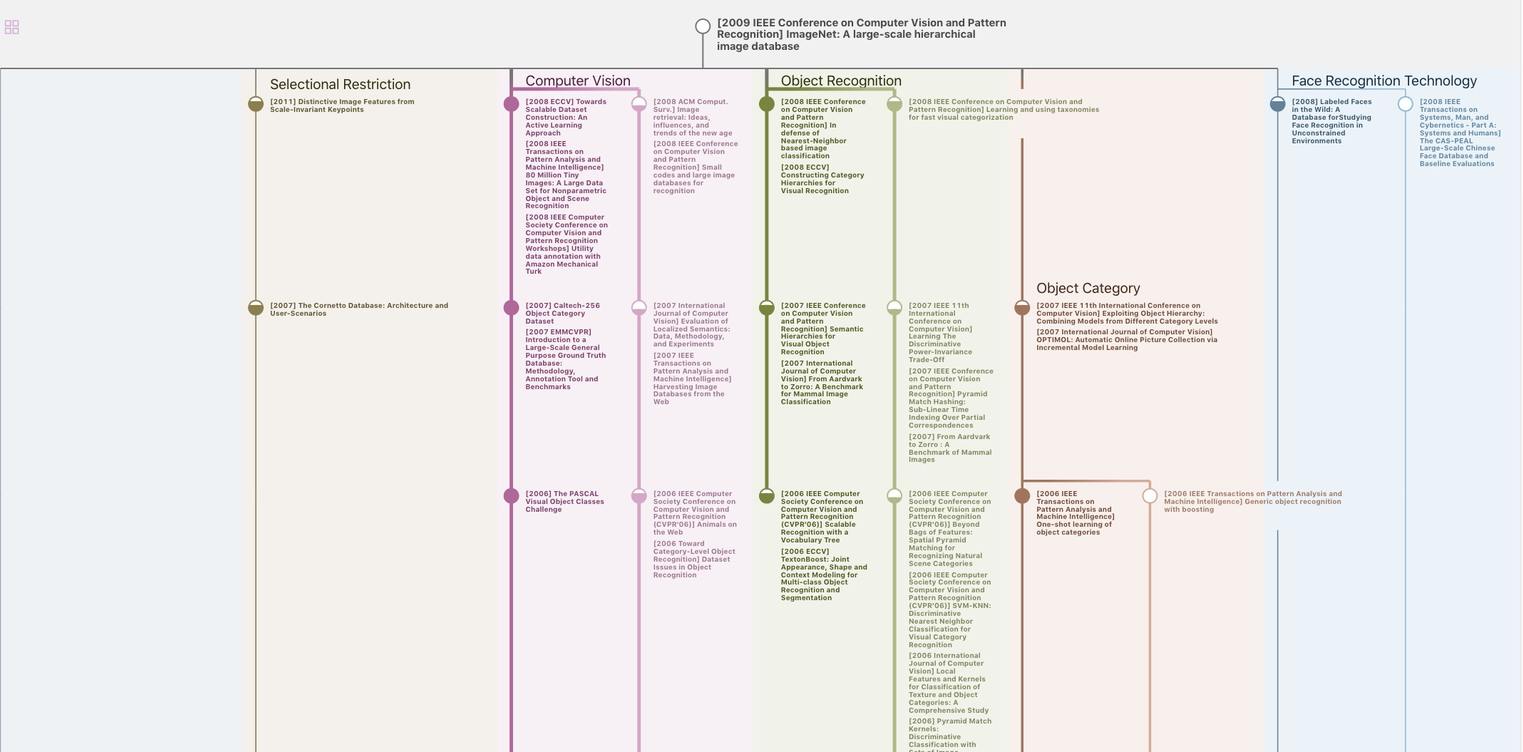
生成溯源树,研究论文发展脉络
Chat Paper
正在生成论文摘要