Sleep pattern inference using IoT sonar monitoring and machine learning with Kennard-stone balance algorithm
Periodicals(2021)
摘要
AbstractAbstractA new paradigm of IoT monitoring using sonar sensors and microphones is studied as a contactless alternative to the traditional devices for sleep disorders in this paper. A pair of sonar sensors are used to measure the distance from the bottom and side of the body to the bedside respectively, and the basic posture of the human body during sleep can be inferred by using machine learning. When a person sleeps still, two streams of sonar signals from two adjacent sides remain unchanged. Any movement would disrupt the stationary sonar streams. Hence it could be detected as change of posture from lying still. Different sonar patterns could be recognized as specific sleeping postures by some non-linear machine learning model. This simple and novel solution could potentially be used as an alternative or supplementary to video analytics which can feedback to the user about their sleeping pattern. A new data transformation method namely Kennard-stone Balance (KSB) algorithm is also proposed for simplifying the data streams and enhancing the accuracy of the machine learning model. Simulation results show the feasibility of this sonar method, and KSB is able to improve the pattern recognition performance.
更多查看译文
关键词
Sleep pattern analysis, Data stream mining, Sonar sensors, IoT monitoring, Data sampling
AI 理解论文
溯源树
样例
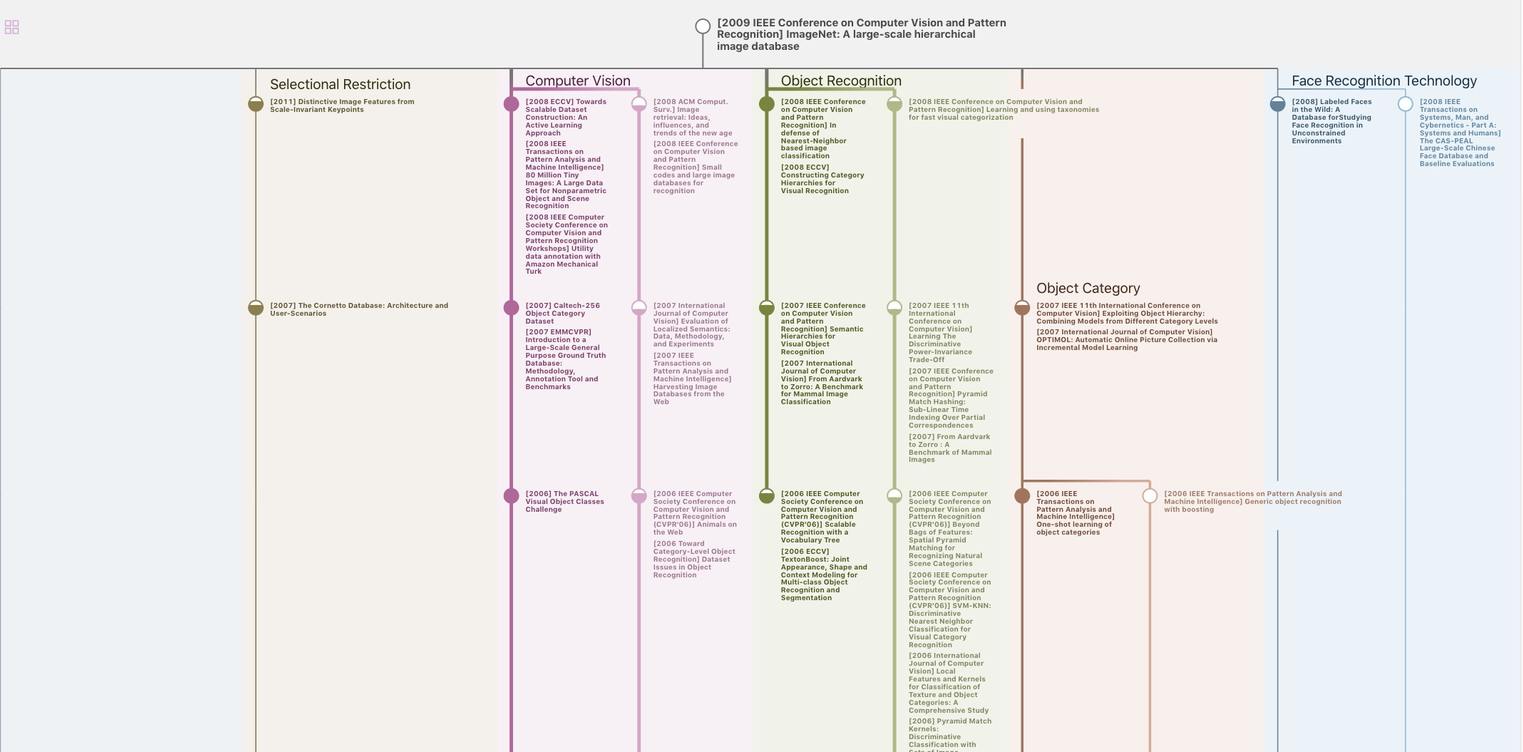
生成溯源树,研究论文发展脉络
Chat Paper
正在生成论文摘要