Order Optimal Bounds for One-Shot Federated Learning over non-Convex Loss Functions
arxiv(2021)
摘要
We consider the problem of federated learning in a one-shot setting in which
there are m machines, each observing n sample functions from an unknown
distribution on non-convex loss functions. Let F:[-1,1]^d→ℝ be the
expected loss function with respect to this unknown distribution. The goal is
to find an estimate of the minimizer of F. Based on its observations, each
machine generates a signal of bounded length B and sends it to a server. The
server collects signals of all machines and outputs an estimate of the
minimizer of F. We show that the expected loss of any algorithm is lower
bounded by max(1/(√(n)(mB)^1/d), 1/√(mn)), up to a
logarithmic factor. We then prove that this lower bound is order optimal in m
and n by presenting a distributed learning algorithm, called Multi-Resolution
Estimator for Non-Convex loss function (MRE-NC), whose expected loss matches
the lower bound for large mn up to polylogarithmic factors.
更多查看译文
关键词
Federated learning,Distributed learning,Communication efficiency,non-Convex Optimization
AI 理解论文
溯源树
样例
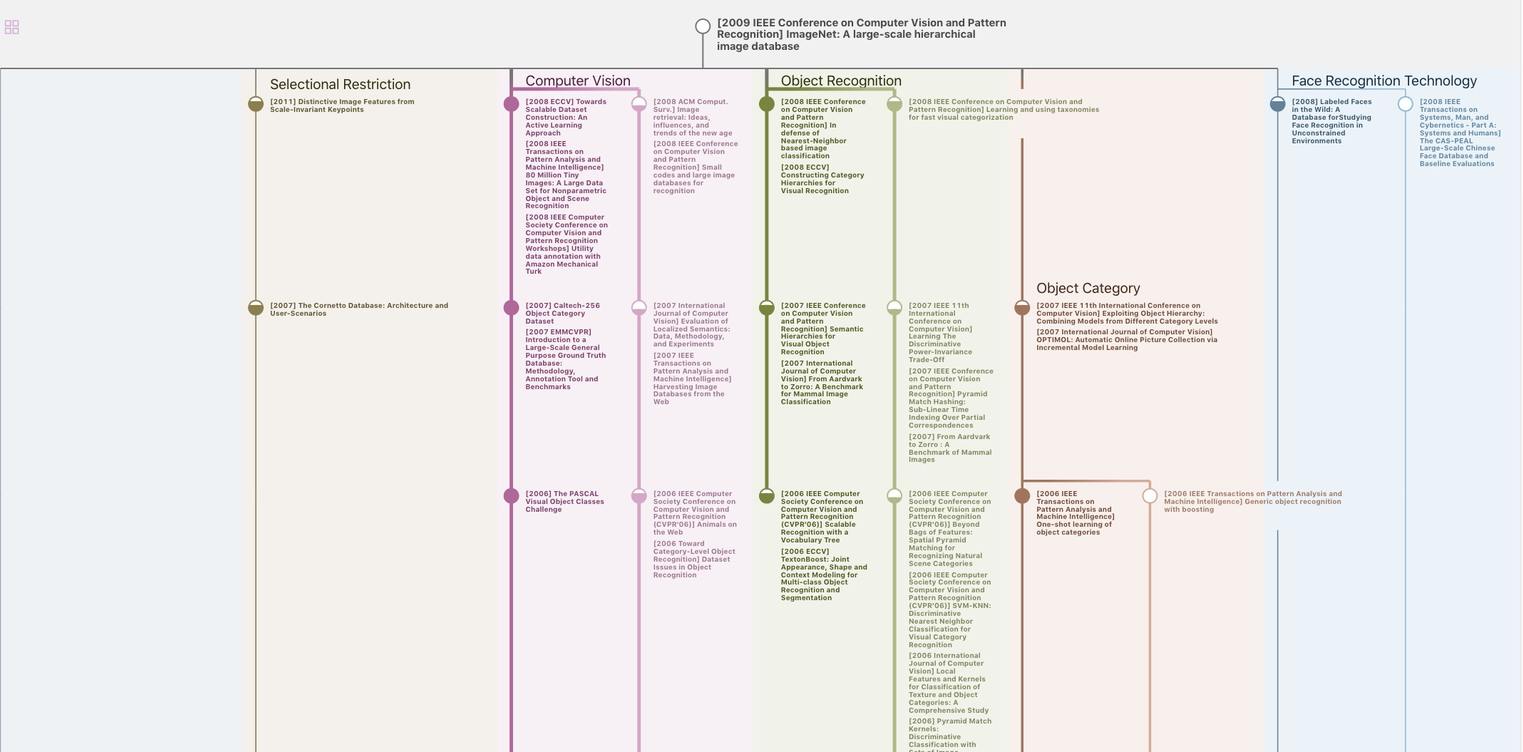
生成溯源树,研究论文发展脉络
Chat Paper
正在生成论文摘要