Pixel-Perfect Structure-from-Motion with Featuremetric Refinement.
ICCV(2021)
摘要
Finding local features that are repeatable across multiple views is a cornerstone of sparse 3D reconstruction. The classical image matching paradigm detects keypoints per-image once and for all, which can yield poorly-localized features and propagate large errors to the final geometry. In this paper, we refine two key steps of structure-from-motion by a direct alignment of low-level image information from multiple views: we first adjust the initial keypoint locations prior to any geometric estimation, and subsequently refine points and camera poses as a post-processing. This refinement is robust to large detection noise and appearance changes, as it optimizes a featuremetric error based on dense features predicted by a neural network. This significantly improves the accuracy of camera poses and scene geometry for a wide range of keypoint detectors, challenging viewing conditions, and off-the-shelf deep features. Our system easily scales to large image collections, enabling pixel-perfect crowd-sourced localization at scale. Our code is publicly available at https://github.com/cvg/pixel-perfect-sfm as an add-on to the popular SfM software COLMAP.
更多查看译文
关键词
Bundle adjustment,feature matching,featuremetric optimization,structure-from-motion,visual localization
AI 理解论文
溯源树
样例
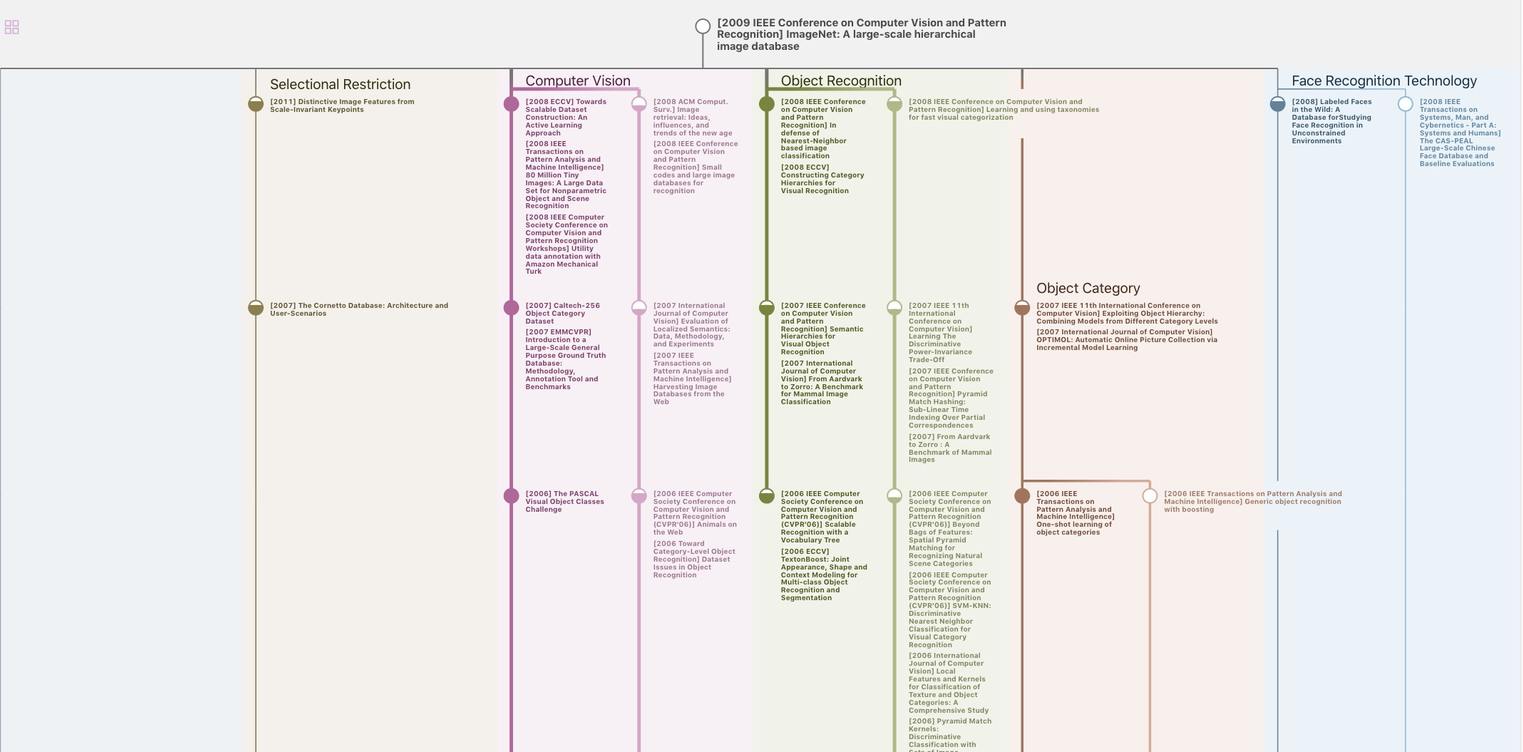
生成溯源树,研究论文发展脉络
Chat Paper
正在生成论文摘要