Layer-wise Adaptive Graph Convolution Networks Using Generalized Pagerank
arxiv(2022)
摘要
We investigate adaptive layer-wise graph convolution in deep GCN models. We propose AdaGPR to learn generalized Pageranks at each layer of a GCNII network to induce adaptive convolution. We show that the generalization bound for AdaGPR is bounded by a polynomial of the eigenvalue spectrum of the normalized adjacency matrix in the order of the number of generalized Pagerank coefficients. By analysing the generalization bounds we show that oversmoothing depends on both the convolutions by the higher orders of the normalized adjacency matrix and the depth of the model. We performed evaluations on node-classification using benchmark real data and show that AdaGPR provides improved accuracies compared to existing graph convolution networks while demonstrating robustness against oversmoothing. Further, we demonstrate that analysis of coefficients of layer-wise generalized Pageranks allows us to qualitatively understand convolution at each layer enabling model interpretations.
更多查看译文
关键词
networks,graph,layer-wise
AI 理解论文
溯源树
样例
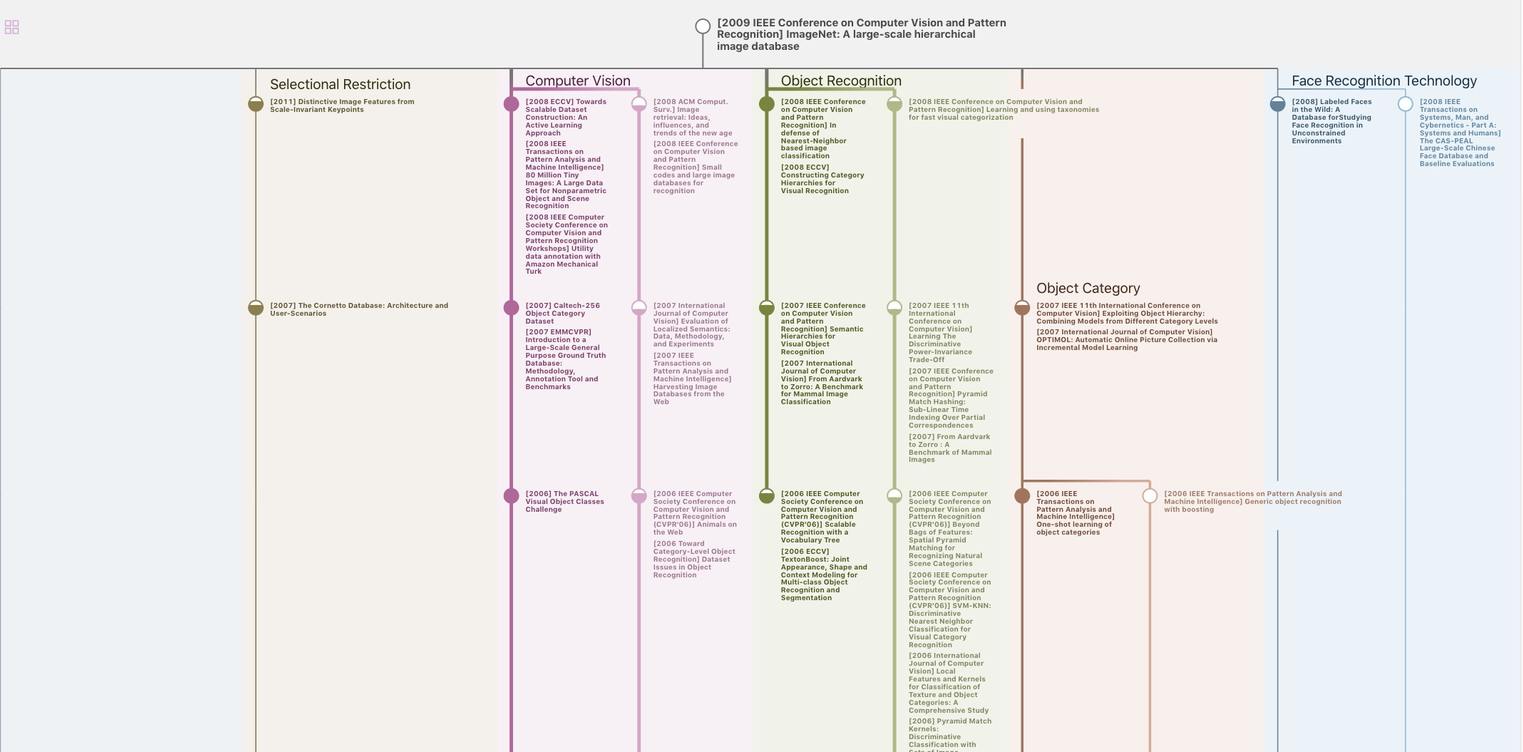
生成溯源树,研究论文发展脉络
Chat Paper
正在生成论文摘要