ARAPReg - An As-Rigid-As Possible Regularization Loss for Learning Deformable Shape Generators.
ICCV(2021)
摘要
This paper introduces an unsupervised loss for training parametric deformation shape generators. The key idea is to enforce the preservation of local rigidity among the generated shapes. Our approach builds on an approximation of the as-rigid-as possible (or ARAP) deformation energy. We show how to develop the unsupervised loss via a spectral decomposition of the Hessian of the ARAP energy. Our loss nicely decouples pose and shape variations through a robust norm. The loss admits simple closed-form expressions. It is easy to train and can be plugged into any standard generation models, e.g., variational auto-encoder (VAE) and auto-decoder (AD). Experimental results show that our approach outperforms existing shape generation approaches considerably on public benchmark datasets of various shape categories such as human, animal and bone.
更多查看译文
关键词
Neural generative models,Optimization and learning methods
AI 理解论文
溯源树
样例
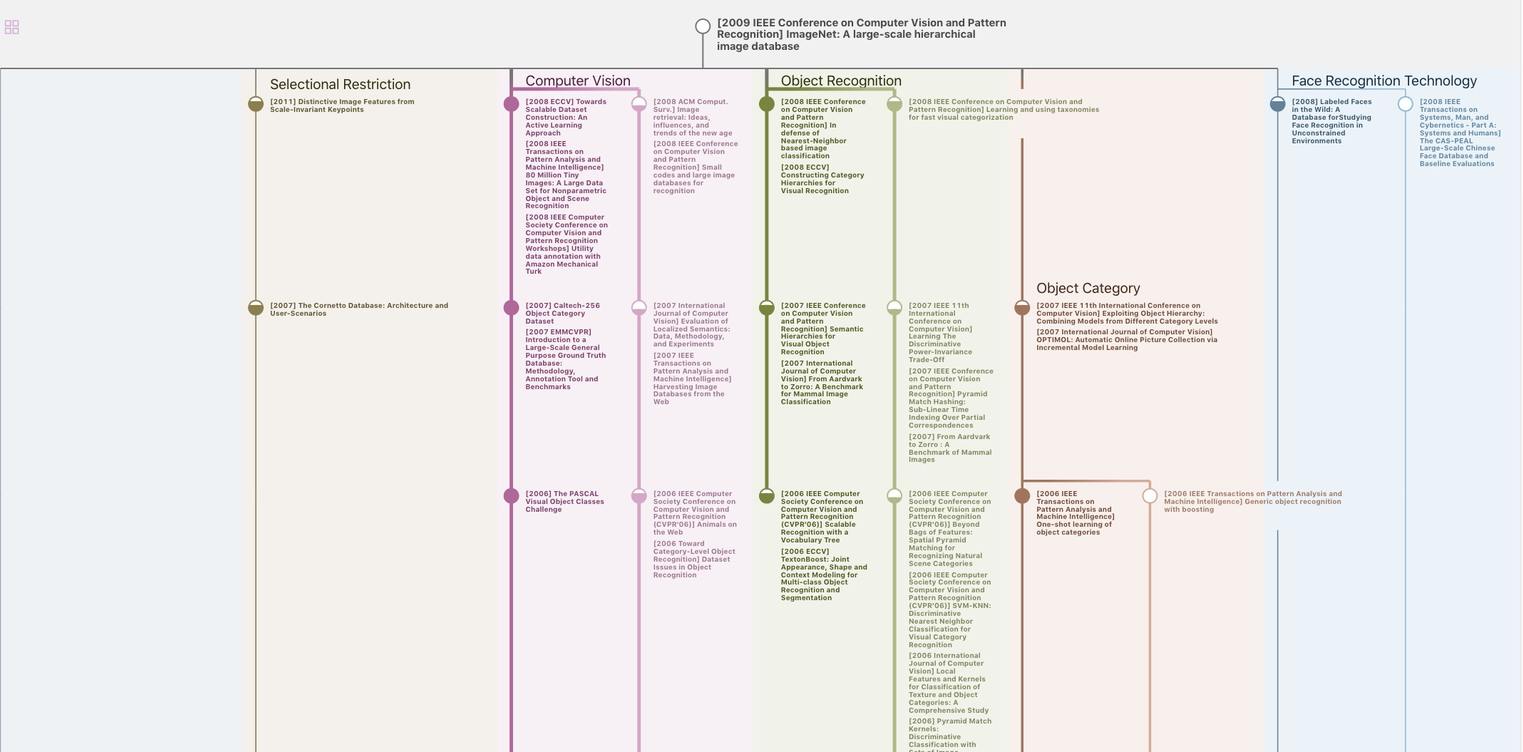
生成溯源树,研究论文发展脉络
Chat Paper
正在生成论文摘要