Investigating a Baseline Of Self Supervised Learning Towards Reducing Labeling Costs For Image Classification
CoRR(2021)
摘要
Data labeling in supervised learning is considered an expensive and infeasible tool in some conditions. The self-supervised learning method is proposed to tackle the learning effectiveness with fewer labeled data, however, there is a lack of confidence in the size of labeled data needed to achieve adequate results. This study aims to draw a baseline on the proportion of the labeled data that models can appreciate to yield competent accuracy when compared to training with additional labels. The study implements the kaggle.com' cats-vs-dogs dataset, Mnist and Fashion-Mnist to investigate the self-supervised learning task by implementing random rotations augmentation on the original datasets. To reveal the true effectiveness of the pretext process in self-supervised learning, the original dataset is divided into smaller batches, and learning is repeated on each batch with and without the pretext pre-training. Results show that the pretext process in the self-supervised learning improves the accuracy around 15% in the downstream classification task when compared to the plain supervised learning.
更多查看译文
关键词
self supervised learning,labeling costs,supervised learning,image classification
AI 理解论文
溯源树
样例
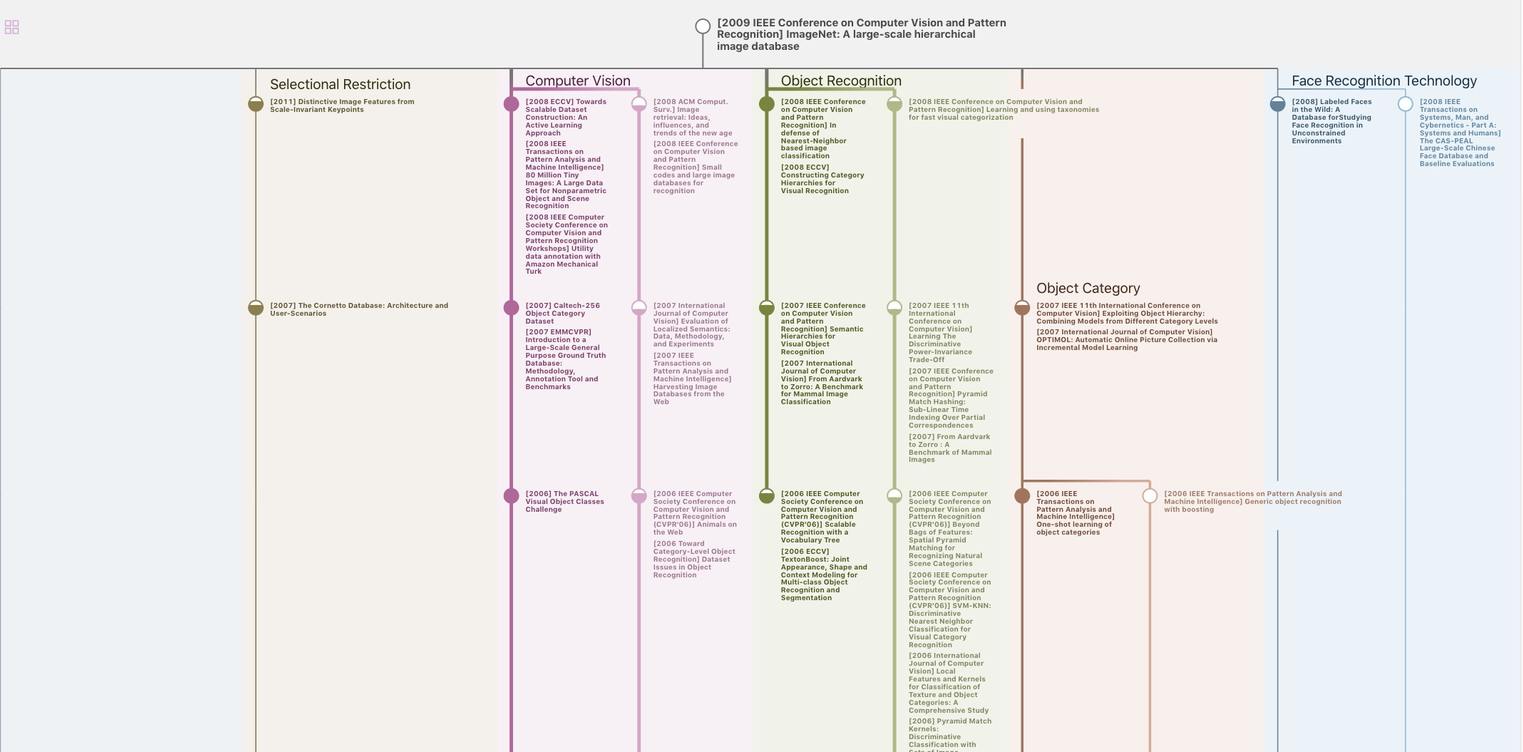
生成溯源树,研究论文发展脉络
Chat Paper
正在生成论文摘要