Towards Structured Dynamic Sparse Pre-Training of BERT
arxiv(2021)
摘要
Identifying algorithms for computational efficient unsupervised training of large language models is an important and active area of research. In this work, we develop and study a straightforward, dynamic always-sparse pre-training approach for BERT language modeling task, which leverages periodic compression steps based on magnitude pruning followed by random parameter re-allocation. This approach enables us to achieve Pareto improvements in terms of the number of floating-point operations (FLOPs) over statically sparse and dense models across a broad spectrum of network sizes. Furthermore, we demonstrate that training remains FLOP-efficient when using coarse-grained block sparsity, making it particularly promising for efficient execution on modern hardware accelerators.
更多查看译文
关键词
bert,dynamic,pre-training
AI 理解论文
溯源树
样例
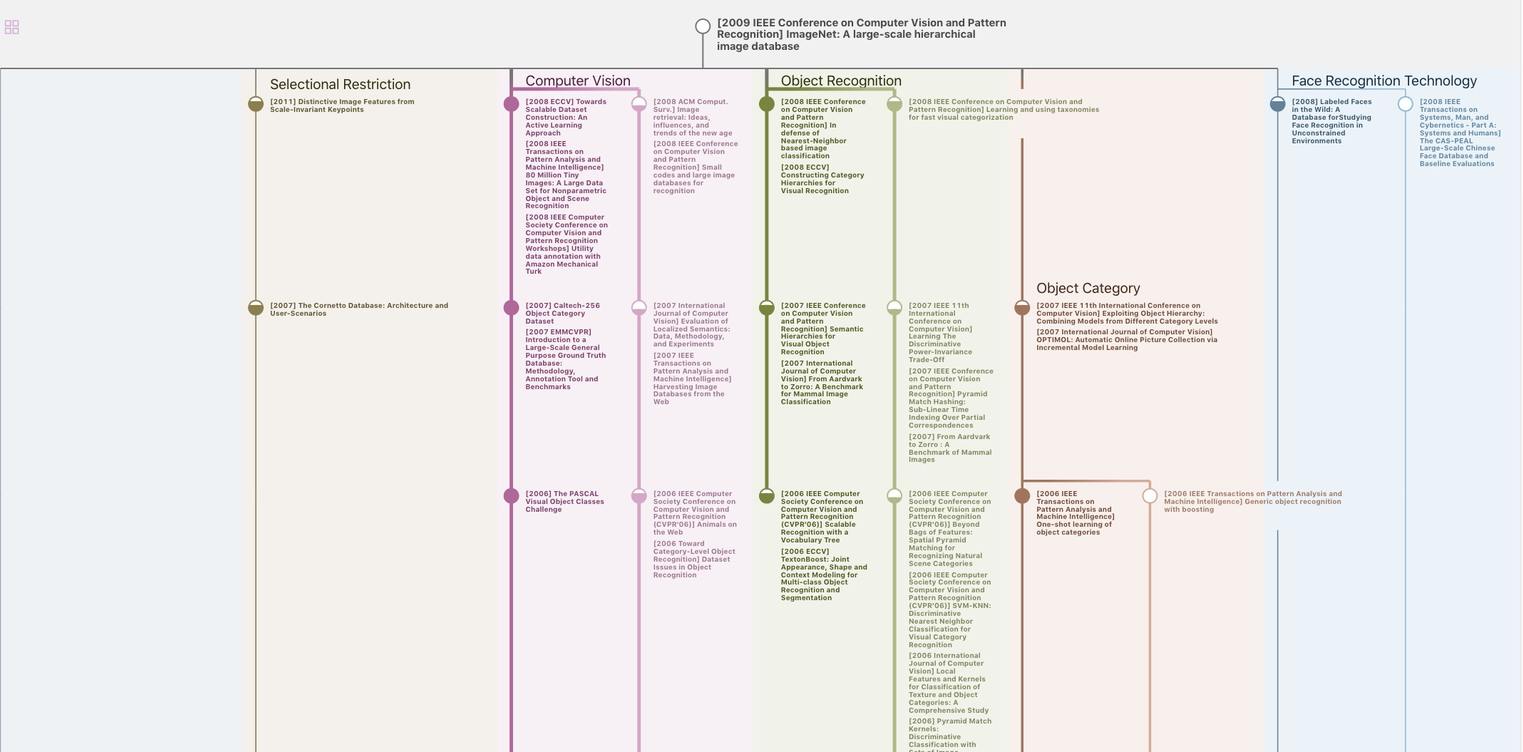
生成溯源树,研究论文发展脉络
Chat Paper
正在生成论文摘要