Binary Differential Evolution based Feature Selection Method with Mutual Information for Imbalanced Classification Problems
2021 IEEE CONGRESS ON EVOLUTIONARY COMPUTATION (CEC 2021)(2021)
摘要
The feature selection process aims to eliminate redundant attributes in the data set, thus it leads to improved classification accuracy. The solution to a feature selection problem is challenging due to the ever-increasing data volume. This problem gets more complicated in the case of imbalanced data sets. Most of the traditional feature selection methods weigh on the majority class when selecting the informative feature subset, thus the selected features often get a bias towards the majority class and neglect the significance of the minority class in the whole process, which results in poor classification performance in the case of minority class objects. Multiple evolutionary algorithms based feature selection methods have been introduced in the past but most of them ignore the class imbalance problem while selecting the most informative feature subset. In this article, we propose a binary differential evolution algorithm with Manhattan distance-based mutation, which employs a joint mutual information maximization based feature selection criteria along with a novel class distribution-based weight assignment scheme to tackle the class imbalance problem. In the experimental studies, we have tested the performance of the proposed method on well-known data sets using three widely-used performance metrics (Average Classification Accuracy, F-measure, G-Means). According to the empirical results, the proposed method performs better than its contenders in most of the data sets.
更多查看译文
关键词
Feature Selection, Differential Evolution, Class Imbalance, Evolutionary Algorithms
AI 理解论文
溯源树
样例
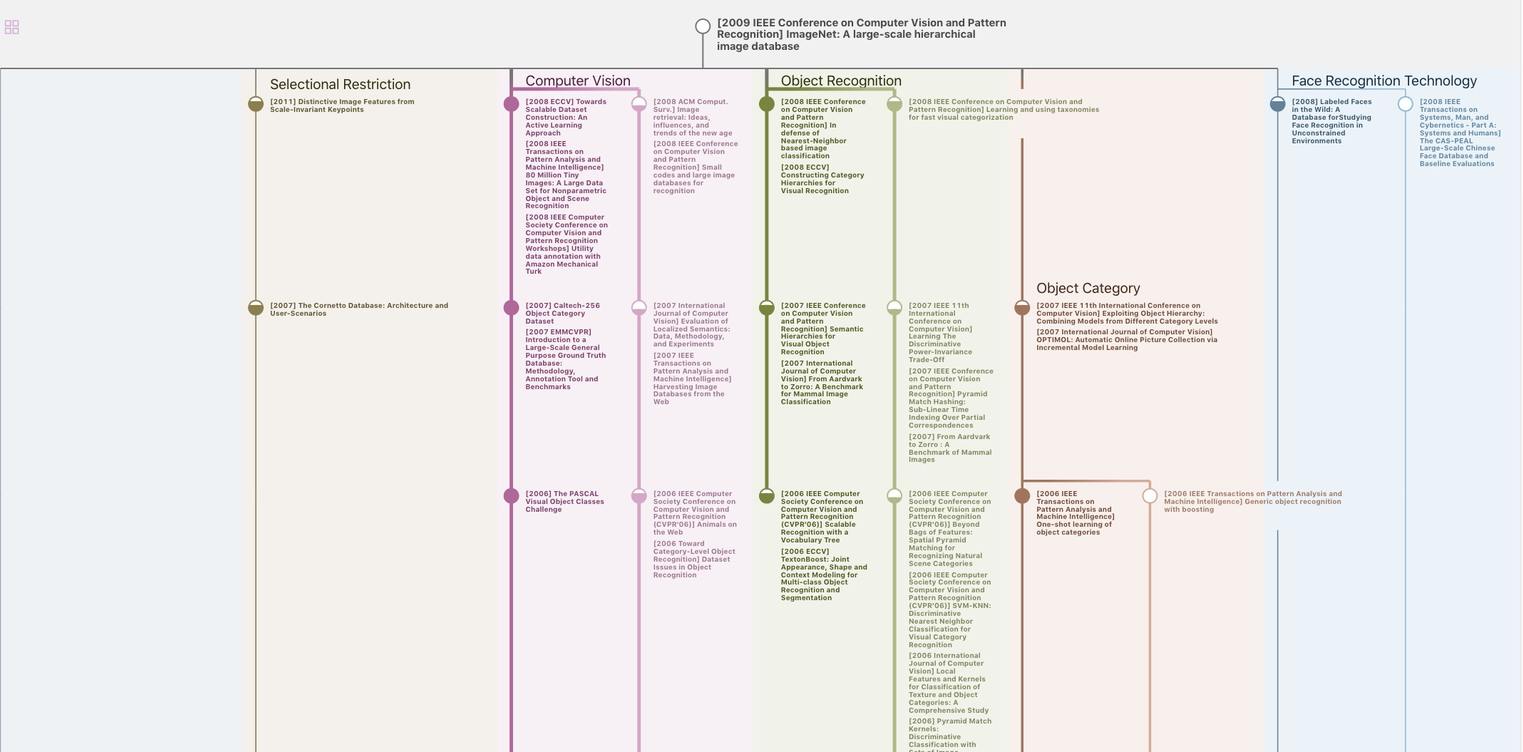
生成溯源树,研究论文发展脉络
Chat Paper
正在生成论文摘要