A Voxel Graph CNN for Object Classification with Event Cameras.
arxiv(2022)
摘要
Event cameras attract researchers' attention due to their low power consumption, high dynamic range, and extremely high temporal resolution. Learning models on event-based object classification have recently achieved massive success by accumulating sparse events into dense frames to apply traditional 2D learning methods. Yet, these approaches necessitate heavy-weight models and are with high computational complexity due to the redundant information introduced by the sparse-to-dense conversion, limiting the potential of event cameras on real-life applications. This study aims to address the core problem of balancing accuracy and model complexity for event-based classification models. To this end, we introduce a novel graph representation for event data to exploit their sparsity better and customize a lightweight voxel graph convolutional neural network (EV-VGCNN) for event-based classification. Specifically, (1) using voxel-wise vertices rather than previous point-wise inputs to explicitly exploit regional 2D semantics of event streams while keeping the sparsity; (2) proposing a multi-scale feature relational layer (MFRL) to extract spatial and motion cues from each vertex discriminatively concerning its distances to neighbors. Comprehensive experiments show that our model can advance state-of-the-art classification accuracy with extremely low model complexity (merely 0.84M parameters).
更多查看译文
关键词
voxel graph cnn,object classification,event
AI 理解论文
溯源树
样例
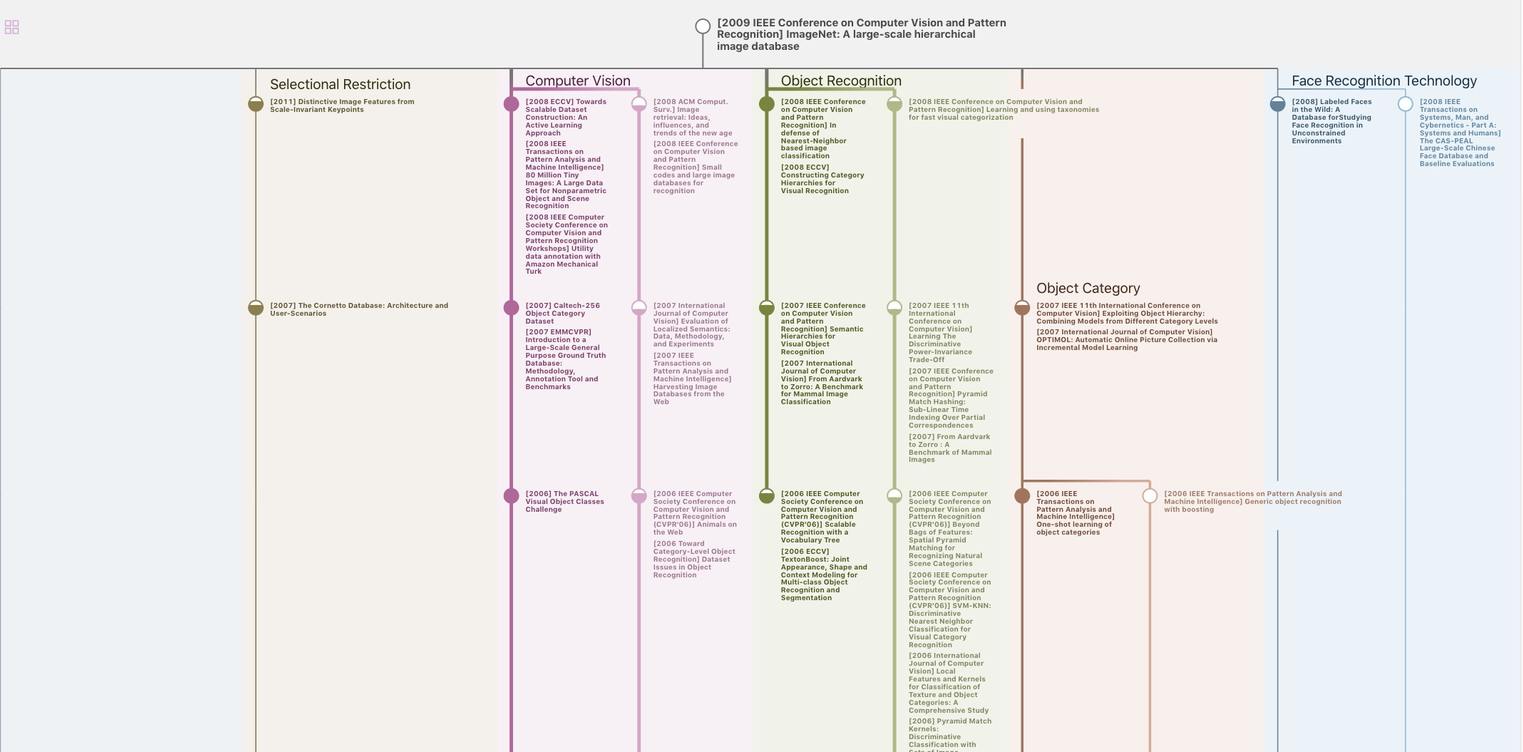
生成溯源树,研究论文发展脉络
Chat Paper
正在生成论文摘要