HHGN: A Hierarchical Reasoning-based Heterogeneous Graph Neural Network for fact verification
Information Processing & Management(2021)
摘要
Fact verification aims to retrieve related evidence from raw text to verify the correctness of a given claim. Existing works mainly leverage the single-granularity features for the representation learning of evidences, i.e., sentence features, ignoring other features like entity-level and context-level features. In addition, they usually focus on improving the prediction accuracy while lacking the interpretability of the inference process, which leads to unreliable results. Thus, in this paper, to investigate how to utilize multi-granularity semantic units for evidence representation as well as to improve the explainability of evidence reasoning, we propose a Hierarchical Reasoning-based Heterogeneous Graph Neural Network for fact verification (HHGN). HHGN combines multiple features of entity, sentence as well as context for evidence representation, and employs a heterogeneous graph to capture their semantic relations. Inspired by the human inference process, we design a hierarchical reasoning-based node updating strategy to propagate the evidence features. Then, we extract the potential reasoning paths from the graph to predict the label, which aggregates the results of different paths weighted by their relevance to the claim. We evaluate our proposal on FEVER, a large-scale benchmark dataset for fact verification. Our experimental results demonstrate the superiority of HHGN over the competitive baselines in both single evidence and multiple evidences settings. In addition, HHGN presents reasonable interpretability in the form of aggregating the features of relevant entity units and selecting the evidence sentences with high confidence.
更多查看译文
关键词
Fact verification,Graph neural network,Hierarchical reasoning,Heterogeneous graph
AI 理解论文
溯源树
样例
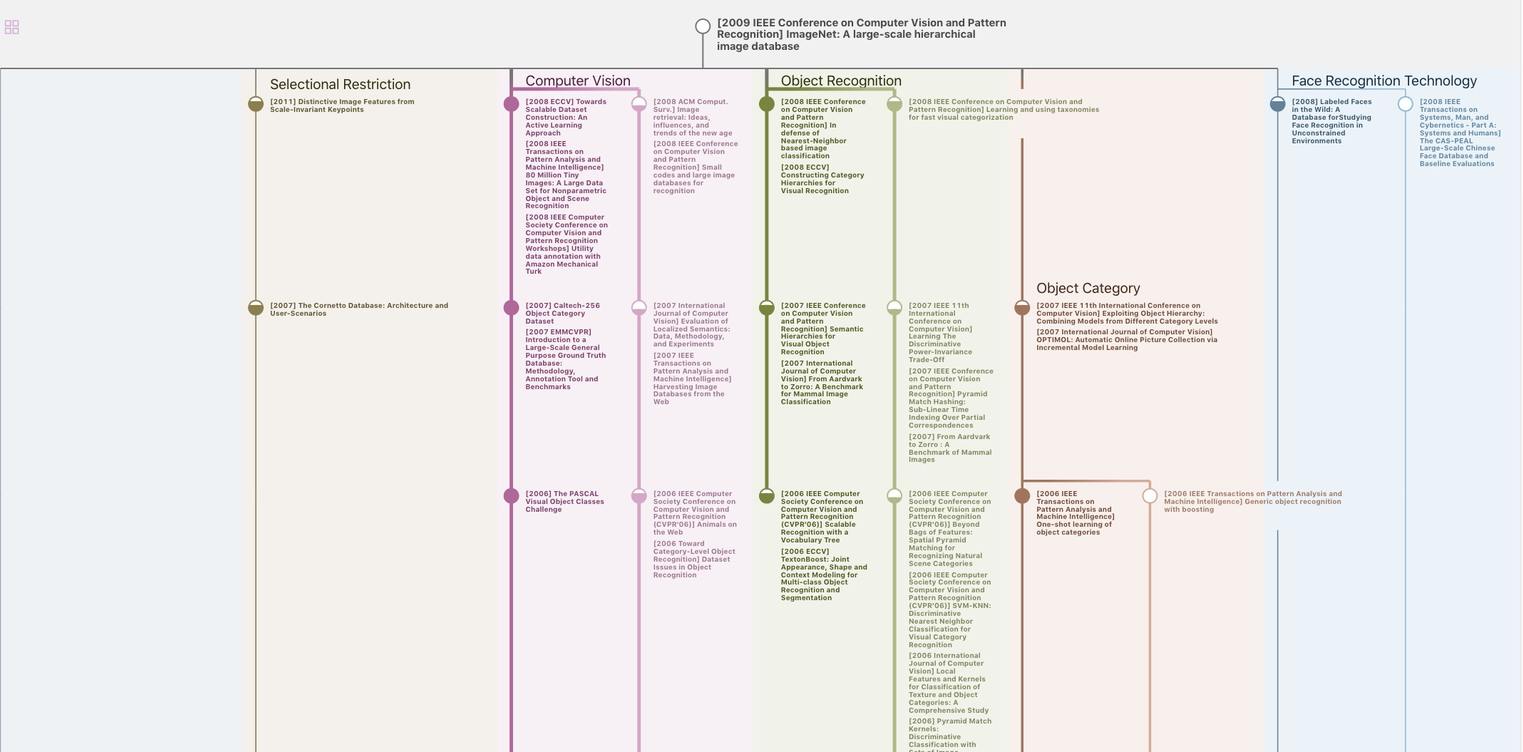
生成溯源树,研究论文发展脉络
Chat Paper
正在生成论文摘要