A Semantics-aware Transformer Model of Relation Linking for Knowledge Base Question Answering.
ACL-IJCNLP 2021: THE 59TH ANNUAL MEETING OF THE ASSOCIATION FOR COMPUTATIONAL LINGUISTICS AND THE 11TH INTERNATIONAL JOINT CONFERENCE ON NATURAL LANGUAGE PROCESSING, VOL 2(2021)
摘要
Relation linking is a crucial component of Knowledge Base Question Answering systems. Existing systems use a wide variety of heuristics, or ensembles of multiple systems, heavily relying on the surface question text. However, the explicit semantic parse of the question is a rich source of relation information that is not taken advantage of. We propose a simple transformer-based neural model for relation linking that leverages the AMR semantic parse of a sentence. Our system significantly outperforms the state-of-the-art on 4 popular benchmark datasets. These are based on either DBpedia or Wikidata, demonstrating that our approach is effective across KGs.
更多查看译文
关键词
Transformer (machine learning model),Semantics (computer science),Relation (database),Natural language processing,Computer science,Artificial intelligence,Knowledge base question answering
AI 理解论文
溯源树
样例
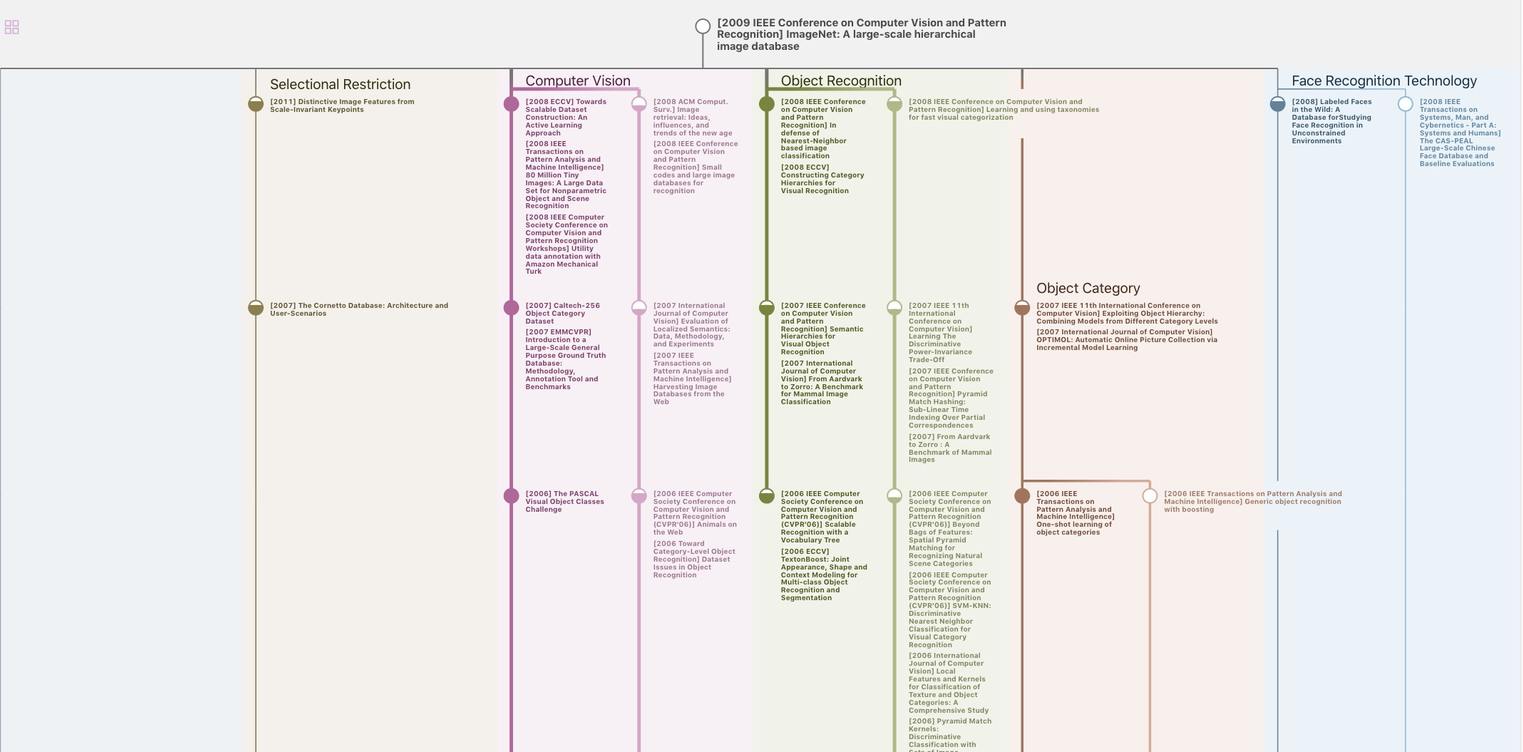
生成溯源树,研究论文发展脉络
Chat Paper
正在生成论文摘要