Machine Learning for Predicting the B-z Magnetic Field Component From Upstream in Situ Observations of Solar Coronal Mass Ejections
SPACE WEATHER-THE INTERNATIONAL JOURNAL OF RESEARCH AND APPLICATIONS(2021)
摘要
Predicting the B-z magnetic field embedded within interplanetary coronal mass ejections (ICMEs), also known as the B-z problem, is a key challenge in space weather forecasting. We study the hypothesis that upstream in situ measurements of the sheath region and the first few hours of the magnetic obstacle provide sufficient information for predicting the downstream B-z component. To do so, we develop a predictive tool based on machine learning that is trained and tested on 348 ICMEs from Wind, STEREO-A, and STEREO-B measurements. We train the machine learning models to predict the minimum value of the B-z component and the maximum value of the total magnetic field B-t in the magnetic obstacle. To validate the tool, we let the ICMEs sweep over the spacecraft and assess how continually feeding in situ measurements into the tool improves the B-z prediction. We specifically find that the predictive tool can predict the minimum value of the B-z component in the magnetic obstacle with a mean absolute error of 3.12 nT and a Pearson correlation coefficient of 0.71 when the sheath region and the first 4 hr of the magnetic obstacle are observed. While the underlying hypothesis is unlikely to solve the B-z problem, the tool shows promise for ICMEs that have a recognizable magnetic flux rope signature. Transitioning the tool to operations could lead to improved space weather forecasting.
更多查看译文
关键词
Coronal mass ejections
AI 理解论文
溯源树
样例
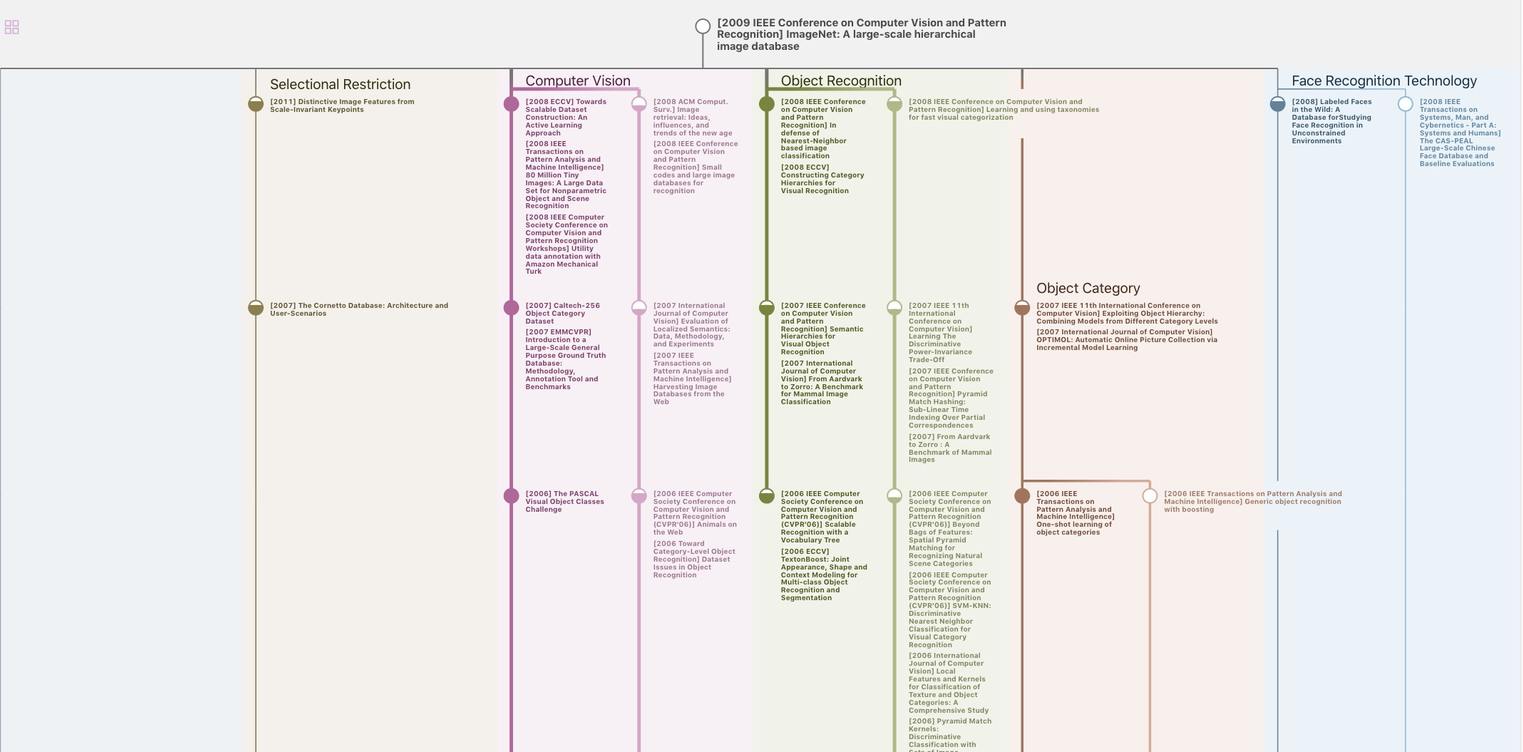
生成溯源树,研究论文发展脉络
Chat Paper
正在生成论文摘要